

Capturing the benefits of remote learning
How education experts are applying lessons learned in the pandemic to promote positive outcomes for all students
Vol. 52 No. 6 Print version: page 46
- Schools and Classrooms

With schools open again after more than a year of teaching students outside the classroom, the pandemic sometimes feels like a distant memory. The return to classrooms this fall brings major relief for many families and educators. Factors such as a lack of reliable technology and family support, along with an absence of school resources, resulted in significant academic setbacks, not to mention stress for everyone involved.
But for all the downsides of distance learning, educators, psychologists, and parents have seen some benefits as well. For example, certain populations of students found new ways to be more engaged in learning, without the distractions and difficulties they faced in the classroom, and the general challenges of remote learning and the pandemic brought mental health to the forefront of the classroom experience.
Peter Faustino, PsyD, a school psychologist in Scarsdale, New York, said the pandemic also prompted educators and school psychologists to find creative new ways of ensuring students’ emotional and academic well-being. “So many students were impacted by the pandemic, so we couldn’t just assume they would find resources on their own,” said Faustino. “We had to work hard at figuring out new ways to connect with them.”
Here are some of the benefits of distance learning that school psychologists and educators have observed and the ways in which they’re implementing those lessons post-pandemic, with the goal of creating a more equitable, productive environment for all students.
Prioritizing mental health
Faustino said that during the pandemic, he had more mental health conversations with students, families, and teachers than ever. “Because COVID-19 affected everyone, we’re now having mental health discussions as school leaders on a daily and weekly basis,” he said.
This renewed focus on mental health has the potential to improve students’ well-being in profound ways—starting with helping them recover from the pandemic’s effects. In New York City, for example, schools are hiring more than 600 new clinicians, including psychologists , to screen students’ mental health and help them process pandemic-related trauma and adjust to the “new normal” of attending school in person.
Educators and families are also realizing the importance of protecting students’ mental health more generally—not only for their health and safety but for their learning. “We’ve been seeing a broader appreciation for the fact that mental health is a prerequisite for learning rather than an extracurricular pursuit,” said Eric Rossen, PhD, director of professional development and standards at the National Association of School Psychologists.
As a result, Rossen hopes educators will embed social and emotional learning components into daily instruction. For example, teachers could teach mindfulness techniques in the classroom and take in-the-moment opportunities to help kids resolve conflicts or manage stress.
Improved access to mental health resources in schools is another positive effect. Because of physical distancing guidelines, school leaders had to find ways to deliver mental health services remotely, including via online referrals and teletherapy with school psychologists and counselors.
Early in the pandemic, Faustino said he was hesitant about teletherapy’s effectiveness; now, he hopes to continue offering a virtual option. Online scheduling and remote appointments make it easier for students to access mental health resources, and some students even enjoy virtual appointments more, as they can attend therapy in their own spaces rather than showing up in the counselor’s office. For older students, Faustino said that level of comfort often leads to more productive, open conversations.
Autonomy as a key to motivation
Research suggests that when students have more choices about their materials and activities, they’re more motivated—which may translate to increased learning and academic success. In a 2016 paper, psychology researcher Allan Wigfield, PhD, and colleagues make the case that control and autonomy in reading activities can improve both motivation and comprehension ( Child Development Perspectives , Vol. 10, No. 3 ).
During the period of online teaching, some students had opportunities to learn at their own pace, which educators say improved their learning outcomes—especially in older students. In a 2020 survey of more than 600 parents, researchers found the second-most-valued benefit of distance learning was flexibility—not only in schedule but in method of learning.
In a recent study, researchers found that 18% of parents pointed to greater flexibility in a child’s schedule or way of learning as the biggest benefit or positive outcome related to remote learning ( School Psychology , Roy, A., et al., in press).
This individualized learning helps students find more free time for interests and also allows them to conduct their learning at a time they’re most likely to succeed. During the pandemic, Mark Gardner, an English teacher at Hayes Freedom High School in Camas, Washington, said he realized how important student-centered learning is and that whether learning happens should take precedence over how and when it occurs.
For example, one of his students thrived when he had the choice to do work later at night because he took care of his siblings during the day. Now, Gardner posts homework online on Sundays so students can work at their own pace during the week. “Going forward, we want to create as many access points as we can for kids to engage with learning,” he said.
Rosanna Breaux , PhD, an assistant professor of psychology and assistant director of the Child Study Center at Virginia Tech, agrees. “I’d like to see this flexibility continue in some way, where—similar to college—students can guide their own learning based on their interests or when they’re most productive,” she said.
During the pandemic, many educators were forced to rethink how to keep students engaged. Rossen said because many school districts shared virtual curricula during the period of remote learning, older students could take more challenging or interesting courses than they could in person. The same is true for younger students: Megan Hibbard, a teacher in White Bear Lake, Minnesota, said many of her fifth graders enjoyed distance learning more than in-person because they could work on projects that aligned with their interests.
“So much of motivation is discovering the unique things the student finds interesting,” said Hunter Gehlbach, PhD, a professor and vice dean at the Johns Hopkins School of Education. “The more you can facilitate students spending more time on the things they’re really interested in, the better.”
Going forward, Rossen hopes virtual curricula will allow students greater opportunities to pursue their interests, such as by taking AP classes, foreign languages, or vocational electives not available at their own schools.
Conversely, Hibbard’s goal is to increase opportunities for students to pursue their interests in the in-person setting. For example, she plans to increase what she calls “Genius Hours,” a time at the end of the school day when students can focus on high-interest projects they’ll eventually share with the class.
Better understanding of children's needs
One of the most important predictors of a child’s success in school is parental involvement in their education. For example, in a meta-analysis of studies, researchers linked parental engagement in their middle schoolers’ education with greater measures of success (Hill, N. E., & Tyson, D. F., Developmental Psychology , Vol. 45, No. 3, 2009).
During the pandemic, parents had new opportunities to learn about their kids and, as a result, help them learn. According to a study by Breaux and colleagues, many parents reported that the pandemic allowed them a better understanding of their child’s learning style, needs, or curriculum.
James C. Kaufman , PhD, a professor of educational psychology at the University of Connecticut and the father of an elementary schooler and a high schooler, said he’s had a front-row seat for his sons’ learning for the first time. “Watching my kids learn and engage with classmates has given me some insight in how to parent them,” he said.
Stephen Becker , PhD, a pediatric psychologist at Cincinnati Children’s Hospital Medical Center, said some parents have observed their children’s behavior or learning needs for the first time, which could prompt them to consider assessment and Individualized Education Program (IEP) services. Across the board, Gehlbach said parents are realizing how they can better partner with schools to ensure their kids’ well-being and academic success.
For example, Samantha Marks , PsyD, a Florida-based clinical psychologist, said she realized how much help her middle school daughter, a gifted and talented student with a 504 plan (a plan for how the school will offer support for a student’s disability) for anxiety, needed with independence. “Bringing the learning home made it crystal clear what we needed to teach our daughter to be independent and improve executive functioning” she said. “My takeaway from this is that more parents need to be involved in their children’s education in a healthy, helpful way.”
Marks also gained a deeper understanding of her daughter’s mental health needs. Through her 504 plan, she received help managing her anxiety at school—at home, though, Marks wasn’t always available to help, which taught her the importance of helping her daughter manage her anxiety independently.
Along with parents gaining a deeper understanding of their kids’ needs, the pandemic also prompted greater parent participation in school. For example, Rossen said his kids’ school had virtual school board meetings; he hopes virtual options continue for events like back-to-school information sessions and parenting workshops. “These meetings are often in the evening, and if you’re a single parent or sole caregiver, you may not want to pay a babysitter in order to attend,” he said.
Brittany Greiert, PhD, a school psychologist in Aurora, Colorado, says culturally and linguistically diverse families at her schools benefited from streamlined opportunities to communicate with administrators and teachers. Her district used an app that translates parent communication into 150 languages. Parents can also remotely participate in meetings with school psychologists or teachers, which Greiert says she plans to continue post-pandemic.
Decreased bullying
During stay-at-home orders, kids with neurodevelopmental disorders experienced less bullying than pre-pandemic (McFayden, T. C., et al., Journal of Rural Mental Health , No. 45, Vol. 2, 2021). According to 2019 research, children with emotional, behavioral, and physical health needs experience increased rates of bullying victimization ( Lebrun-Harris, L. A., et al., ), and from the U.S. Department of Education suggests the majority of bullying takes place in person and in unsupervised areas (PDF) .
Scott Graves , PhD, an associate professor of educational studies at The Ohio State University and a member of APA’s Coalition for Psychology in Schools and Education (CPSE), said the supervision by parents and teachers in remote learning likely played a part in reducing bullying. As a result, he’s less worried his Black sons will be victims of microaggressions and racist behavior during online learning.
Some Asian American families also report that remote learning offered protection against racism students may have experienced in person. Shereen Naser, PhD, an associate professor of psychology at Cleveland State University and a member of CPSE, and colleagues found that students are more comfortable saying discriminatory things in school when their teachers are also doing so; Naser suspects this trickle-down effect is less likely to happen when students learn from home ( School Psychology International , 2019).
Reductions in bullying and microaggressions aren’t just beneficial for students’ long-term mental health. Breaux said less bullying at school results in less stress, which can improve students’ self-esteem and mood—both of which impact their ability to learn.
Patricia Perez, PhD, an associate professor of international psychology at The Chicago School of Professional Psychology and a member of CPSE, said it’s important for schools to be proactive in providing spaces for support and cultural expression for students from vulnerable backgrounds, whether in culture-specific clubs, all-school assemblies that address racism and other diversity-related topics, or safe spaces to process feelings with teachers.
According to Rossen, many schools are already considering how to continue supporting students at risk for bullying, including by restructuring the school environment.
One principal, Rossen said, recently switched to single-use bathrooms to avoid congregating in those spaces once in-person learning commences to maintain social distancing requirements. “The principal received feedback from students about how going to the bathroom is much less stressful for these students in part due to less bullying,” he said.
More opportunities for special needs students
In Becker and Breaux’s research, parents of students with attention-deficit/hyperactivity disorder (ADHD), particularly those with a 504 plan and IEP, reported greater difficulties with remote learning. But some students with special learning needs—including those with IEPs and 504 plans—thrived in an at-home learning environment. Recent reporting in The New York Times suggests this is one reason many students want to continue online learning.
According to Cara Laitusis, PhD, a principal research scientist at Educational Testing Service ( ETS ) and a member of CPSE, reduced distractions may improve learning outcomes for some students with disabilities that impact attention in a group setting. “In assessments, small group or individual settings are frequently requested accommodations for some students with ADHD, anxiety, or autism. Being in a quiet place alone without peers for part of the instructional day may also allow for more focus,” she said. However, she also pointed out the benefits of inclusion in the classroom for developing social skills with peers.
Remote learning has improved academic outcomes for students with different learning needs, too. Marks said her seventh-grade daughter, a visual learner, appreciated the increase in video presentations and graphics. Similarly, Hibbard said many of her students who struggle to grasp lessons on the first try have benefited from the ability to watch videos over again until they understand. Post-pandemic, she plans to record bite-size lessons—for example, a 1-minute video of a long division problem—so her students can rewatch and process at their own rate.
Learners with anxiety also appreciate the option not to be in the classroom, because the social pressures of being surrounded by peers can make it hard to focus on academics. “Several of my students have learned more in the last year simply due to the absence of anxiety,” said Rosie Reid, an English teacher at Ygnacio Valley High School in Concord, California, and a 2019 California Teacher of the Year. “It’s just one less thing to negotiate in a learning environment.”
On online learning platforms, it’s easier for kids with social anxiety or shyness to participate. One of Gardner’s students with social anxiety participated far more in virtual settings and chats. Now, Gardner is brainstorming ways to encourage students to chat in person, such as by projecting a chat screen on the blackboard.
Technology has helped school psychologists better engage students, too. For example, Greiert said the virtual setting gave her a new understanding of her students’ personalities and needs. “Typing out their thoughts, they were able to demonstrate humor or complex thoughts they never demonstrated in person,” she said. “I really want to keep incorporating technology into sessions so kids can keep building on their strengths.”
Reid says that along with the high school students she teaches, she’s seen her 6-year-old daughter benefit from learning at her own pace in the familiarity of her home. Before the pandemic, she was behind academically, but by guiding her own learning—writing poems, reading books, playing outside with her siblings—she’s blossomed. “For me, as both a mother and as a teacher, this whole phenomenon has opened the door to what education can be,” Reid said.
Eleanor Di Marino-Linnen, PhD, a psychologist and superintendent of the Rose Tree Media School District in Media, Pennsylvania, says the pandemic afforded her district a chance to rethink old routines and implement new ones. “As challenging as it is, it’s definitely an exciting time to be in education when we have a chance to reenvision what schools have looked like for many years,” she said. “We want to capitalize on what we’ve learned.”
Further reading
Why are some kids thriving during remote learning? Fleming, N., Edutopia, 2020
Remote learning has been a disaster for many students. But some kids have thrived. Gilman, A., The Washington Post , Oct. 3, 2020
A preliminary examination of key strategies, challenges, and benefits of remote learning expressed by parents during the COVID-19 pandemic Roy, A., et al., School Psychology , in press
Remote learning during COVID-19: Examining school practices, service continuation, and difficulties for adolescents with and without attention-deficit/hyperactivity disorder Becker S. P., et al., Journal of Adolescent Health , 2020
Recommended Reading

Contact APA
You may also like.
Information
- Author Services
Initiatives
You are accessing a machine-readable page. In order to be human-readable, please install an RSS reader.
All articles published by MDPI are made immediately available worldwide under an open access license. No special permission is required to reuse all or part of the article published by MDPI, including figures and tables. For articles published under an open access Creative Common CC BY license, any part of the article may be reused without permission provided that the original article is clearly cited. For more information, please refer to https://www.mdpi.com/openaccess .
Feature papers represent the most advanced research with significant potential for high impact in the field. A Feature Paper should be a substantial original Article that involves several techniques or approaches, provides an outlook for future research directions and describes possible research applications.
Feature papers are submitted upon individual invitation or recommendation by the scientific editors and must receive positive feedback from the reviewers.
Editor’s Choice articles are based on recommendations by the scientific editors of MDPI journals from around the world. Editors select a small number of articles recently published in the journal that they believe will be particularly interesting to readers, or important in the respective research area. The aim is to provide a snapshot of some of the most exciting work published in the various research areas of the journal.
Original Submission Date Received: .
- Active Journals
- Find a Journal
- Proceedings Series
- For Authors
- For Reviewers
- For Editors
- For Librarians
- For Publishers
- For Societies
- For Conference Organizers
- Open Access Policy
- Institutional Open Access Program
- Special Issues Guidelines
- Editorial Process
- Research and Publication Ethics
- Article Processing Charges
- Testimonials
- Preprints.org
- SciProfiles
- Encyclopedia
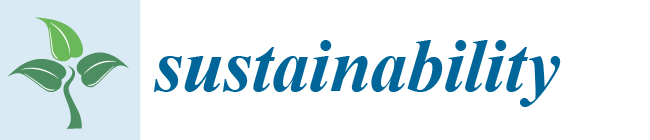
Article Menu
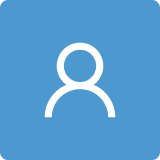
- Subscribe SciFeed
- Recommended Articles
- Google Scholar
- on Google Scholar
- Table of Contents
Find support for a specific problem in the support section of our website.
Please let us know what you think of our products and services.
Visit our dedicated information section to learn more about MDPI.
JSmol Viewer
Assessing the impact of online-learning effectiveness and benefits in knowledge management, the antecedent of online-learning strategies and motivations: an empirical study.
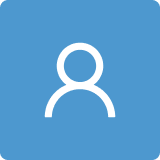
1. Introduction
2. literature review and research hypothesis, 2.1. online-learning self-efficacy terminology, 2.2. online-learning monitoring terminology, 2.3. online-learning confidence in technology terminology, 2.4. online-learning willpower terminology, 2.5. online-learning attitude terminology, 2.6. online-learning motivation terminology, 2.7. online-learning strategies and online-learning effectiveness terminology, 2.8. online-learning effectiveness terminology, 3. research method, 3.1. instruments, 3.2. data analysis and results, 4.1. reliability and validity analysis, 4.2. hypothesis result, 5. discussion, 6. conclusions, 7. limitations and future directions, author contributions, institutional review board statement, informed consent statement, data availability statement, conflicts of interest.
- UNESCO. COVID-19 Educational Disruption and Response ; UNESCO: Paris, France, 2020. [ Google Scholar ]
- Moore, D.R. E-learning and the science of instruction: Proven guidelines for consumers and designers of multimedia learning. Educ. Technol. Res. Dev. 2006 , 54 , 197–200. [ Google Scholar ] [ CrossRef ]
- McDonald, E.W.; Boulton, J.L.; Davis, J.L. E-learning and nursing assessment skills and knowledge–An integrative review. Nurse Educ. Today 2018 , 66 , 166–174. [ Google Scholar ] [ CrossRef ] [ PubMed ]
- Homan, S.R.; Wood, K. Taming the mega-lecture: Wireless quizzing. Syllabus Sunnyvale Chatsworth 2003 , 17 , 23–27. [ Google Scholar ]
- Emran, M.A.; Shaalan, K. E-podium technology: A medium of managing knowledge at al buraimi university college via mlearning. In Proceedings of the 2nd BCS International IT Conference, Abu Dhabi, United Arab Emirates, 9–10 March 2014; pp. 1–4. [ Google Scholar ]
- Tenório, T.; Bittencourt, I.I.; Isotani, S.; Silva, A.P. Does peer assessment in on-line learning environments work? A systematic review of the literature. Comput. Hum. Behav. 2016 , 64 , 94–107. [ Google Scholar ] [ CrossRef ]
- Sheshasaayee, A.; Bee, M.N. Analyzing online learning effectiveness for knowledge society. In Information Systems Design and Intelligent Applications ; Bhateja, V., Nguyen, B., Nguyen, N., Satapathy, S., Le, D.N., Eds.; Springer: Singapore, 2018; pp. 995–1002. [ Google Scholar ]
- Panigrahi, R.; Srivastava, P.R.; Sharma, D. Online learning: Adoption, continuance, and learning outcome—A review of literature. Int. J. Inform. Manag. 2018 , 43 , 1–14. [ Google Scholar ] [ CrossRef ]
- Al-Rahmi, W.M.; Alias, N.; Othman, M.S.; Alzahrani, A.I.; Alfarraj, O.; Saged, A.A. Use of e-learning by university students in Malaysian higher educational institutions: A case in Universiti Teknologi Malaysia. IEEE Access 2018 , 6 , 14268–14276. [ Google Scholar ] [ CrossRef ]
- Al-Rahmi, W.M.; Yahaya, N.; Aldraiweesh, A.A.; Alamri, M.M.; Aljarboa, N.A.; Alturki, U. Integrating technology acceptance model with innovation diffusion theory: An empirical investigation on students’ intention to use E-learning systems. IEEE Access 2019 , 7 , 26797–26809. [ Google Scholar ] [ CrossRef ]
- Gunawan, I.; Hui, L.K.; Ma’sum, M.A. Enhancing learning effectiveness by using online learning management system. In Proceedings of the 6th International Conference on Education and Technology (ICET), Beijing, China, 18–20 June 2021; pp. 48–52. [ Google Scholar ]
- Nguyen, P.H.; Tangworakitthaworn, P.; Gilbert, L. Individual learning effectiveness based on cognitive taxonomies and constructive alignment. In Proceedings of the IEEE Region 10 Conference (Tencon), Osaka, Japan, 16–19 November 2020; pp. 1002–1006. [ Google Scholar ]
- Pee, L.G. Enhancing the learning effectiveness of ill-structured problem solving with online co-creation. Stud. High. Educ. 2020 , 45 , 2341–2355. [ Google Scholar ] [ CrossRef ]
- Kintu, M.J.; Zhu, C.; Kagambe, E. Blended learning effectiveness: The relationship between student characteristics, design features and outcomes. Int. J. Educ. Technol. High. Educ. 2017 , 14 , 1–20. [ Google Scholar ] [ CrossRef ] [ Green Version ]
- Wang, M.H.; Vogel, D.; Ran, W.J. Creating a performance-oriented e-learning environment: A design science approach. Inf. Manag. 2011 , 48 , 260–269. [ Google Scholar ] [ CrossRef ] [ Green Version ]
- Hew, K.F.; Cheung, W.S. Students’ and instructors’ use of massive open online courses (MOOCs): Motivations and challenges. Educ. Res. Rev. 2014 , 12 , 45–58. [ Google Scholar ] [ CrossRef ]
- Bryant, J.; Bates, A.J. Creating a constructivist online instructional environment. TechTrends 2015 , 59 , 17–22. [ Google Scholar ] [ CrossRef ]
- Lee, M.C. Explaining and predicting users’ continuance intention toward e-learning: An extension of the expectation–confirmation model. Comput. Educ. 2010 , 54 , 506–516. [ Google Scholar ] [ CrossRef ]
- Lin, K.M. E-Learning continuance intention: Moderating effects of user e-learning experience. Comput. Educ. 2011 , 56 , 515–526. [ Google Scholar ] [ CrossRef ]
- Huang, E.Y.; Lin, S.W.; Huang, T.K. What type of learning style leads to online participation in the mixed-mode e-learning environment? A study of software usage instruction. Comput. Educ. 2012 , 58 , 338–349. [ Google Scholar ]
- Chu, T.H.; Chen, Y.Y. With good we become good: Understanding e-learning adoption by theory of planned behavior and group influences. Comput. Educ. 2016 , 92 , 37–52. [ Google Scholar ] [ CrossRef ]
- Bandura, A. Self-efficacy: Toward a unifying theory of behavioral change. Psychol. Rev. 1977 , 84 , 191–215. [ Google Scholar ] [ CrossRef ]
- Torkzadeh, G.; Van Dyke, T.P. Development and validation of an Internet self-efficacy scale. Behav. Inform. Technol. 2001 , 20 , 275–280. [ Google Scholar ] [ CrossRef ]
- Saadé, R.G.; Kira, D. Computer anxiety in e-learning: The effect of computer self-efficacy. J. Inform. Technol. Educ. Res. 2009 , 8 , 177–191. [ Google Scholar ] [ CrossRef ] [ Green Version ]
- Tucker, J.; Gentry, G. Developing an E-Learning strategy in higher education. In Proceedings of the SITE 2009–Society for Information Technology & Teacher Education International Conference, Charleston, SC, USA, 2–6 March 2009; pp. 2702–2707. [ Google Scholar ]
- Wang, Y.; Peng, H.M.; Huang, R.H.; Hou, Y.; Wang, J. Characteristics of distance learners: Research on relationships of learning motivation, learning strategy, self-efficacy, attribution and learning results. Open Learn. J. Open Distance Elearn. 2008 , 23 , 17–28. [ Google Scholar ] [ CrossRef ]
- Mahmud, B.H. Study on the impact of motivation, self-efficacy and learning strategies of faculty of education undergraduates studying ICT courses. In Proceedings of the 4th International Postgraduate Research Colloquium (IPRC) Proceedings, Bangkok, Thailand, 29 October 2009; pp. 59–80. [ Google Scholar ]
- Yusuf, M. Investigating relationship between self-efficacy, achievement motivation, and self-regulated learning strategies of undergraduate Students: A study of integrated motivational models. Procedia Soc. Behav. Sci. 2011 , 15 , 2614–2617. [ Google Scholar ] [ CrossRef ] [ Green Version ]
- De la Fuente, J.; Martínez-Vicente, J.M.; Peralta-Sánchez, F.J.; GarzónUmerenkova, A.; Vera, M.M.; Paoloni, P. Applying the SRL vs. ERL theory to the knowledge of achievement emotions in undergraduate university students. Front. Psychol. 2019 , 10 , 2070. [ Google Scholar ] [ CrossRef ] [ PubMed ]
- Ahmadi, S. Academic self-esteem, academic self-efficacy and academic achievement: A path analysis. J. Front. Psychol. 2020 , 5 , 155. [ Google Scholar ]
- Meyen, E.L.; Aust, R.J.; Bui, Y.N. Assessing and monitoring student progress in an E-learning personnel preparation environment. Teach. Educ. Spec. Educ. 2002 , 25 , 187–198. [ Google Scholar ] [ CrossRef ] [ Green Version ]
- Dunlosky, J.; Kubat-Silman, A.K.; Christopher, H. Training monitoring skills improves older adults’ self-paced associative learning. Psychol. Aging 2003 , 18 , 340–345. [ Google Scholar ] [ CrossRef ]
- Zhang, H.J. Research on the relationship between English learning motivation. Self-monitoring and Test Score. Ethnic Educ. Res. 2005 , 6 , 66–71. [ Google Scholar ]
- Rosenberg, M.J. E-Learning: Strategies for Delivering Knowledge in the Digital Age ; McGraw-Hill: New York, NY, USA, 2001. [ Google Scholar ]
- Bhat, S.A.; Bashir, M. Measuring ICT orientation: Scale development & validation. Educ. Inf. Technol. 2018 , 23 , 1123–1143. [ Google Scholar ]
- Achuthan, K.; Francis, S.P.; Diwakar, S. Augmented reflective learning and knowledge retention perceived among students in classrooms involving virtual laboratories. Educ. Inf. Technol. 2017 , 22 , 2825–2855. [ Google Scholar ] [ CrossRef ]
- Hu, X.; Yelland, N. An investigation of preservice early childhood teachers’ adoption of ICT in a teaching practicum context in Hong Kong. J. Early Child. Teach. Educ. 2017 , 38 , 259–274. [ Google Scholar ] [ CrossRef ]
- Fraillon, J.; Ainley, J.; Schulz, W.; Friedman, T.; Duckworth, D. Preparing for Life in a Digital World: The IEA International Computer and Information Literacy Study 2018 International Report ; Springer: New York, NY, USA, 2019. [ Google Scholar ]
- Huber, S.G.; Helm, C. COVID-19 and schooling: Evaluation, assessment and accountability in times of crises—Reacting quickly to explore key issues for policy, practice and research with the school barometer. Educ. Assess. Eval. Account. 2020 , 32 , 237–270. [ Google Scholar ] [ CrossRef ]
- Eickelmann, B.; Gerick, J. Learning with digital media: Objectives in times of Corona and under special consideration of social Inequities. Dtsch. Schule. 2020 , 16 , 153–162. [ Google Scholar ]
- Shehzadi, S.; Nisar, Q.A.; Hussain, M.S.; Basheer, M.F.; Hameed, W.U.; Chaudhry, N.I. The role of e-learning toward students’ satisfaction and university brand image at educational institutes of Pakistan: A post-effect of COVID-19. Asian Educ. Dev. Stud. 2020 , 10 , 275–294. [ Google Scholar ] [ CrossRef ]
- Miller, E.M.; Walton, G.M.; Dweck, C.S.; Job, V.; Trzesniewski, K.; McClure, S. Theories of willpower affect sustained learning. PLoS ONE 2012 , 7 , 38680. [ Google Scholar ] [ CrossRef ] [ PubMed ]
- Moriña, A.; Molina, V.M.; Cortés-Vega, M.D. Voices from Spanish students with disabilities: Willpower and effort to survive university. Eur. J. Spec. Needs Educ. 2018 , 33 , 481–494. [ Google Scholar ] [ CrossRef ]
- Koballa, T.R., Jr.; Crawley, F.E. The influence of attitude on science teaching and learning. Sch. Sci. Math. 1985 , 85 , 222–232. [ Google Scholar ] [ CrossRef ]
- Chao, C.Y.; Chen, Y.T.; Chuang, K.Y. Exploring students’ learning attitude and achievement in flipped learning supported computer aided design curriculum: A study in high school engineering education. Comput. Appl. Eng. Educ. 2015 , 23 , 514–526. [ Google Scholar ] [ CrossRef ]
- Stefan, M.; Ciomos, F. The 8th and 9th grades students’ attitude towards teaching and learning physics. Acta Didact. Napocensia. 2010 , 3 , 7–14. [ Google Scholar ]
- Sedighi, F.; Zarafshan, M.A. Effects of attitude and motivation on the use of language learning strategies by Iranian EFL University students. J. Soc. Sci. Humanit. Shiraz Univ. 2007 , 23 , 71–80. [ Google Scholar ]
- Megan, S.; Jennifer, H.C.; Stephanie, V.; Kyla, H. The relationship among middle school students’ motivation orientations, learning strategies, and academic achievement. Middle Grades Res. J. 2013 , 8 , 1–12. [ Google Scholar ]
- Nasser, O.; Majid, V. Motivation, attitude, and language learning. Procedia Soc. Behav. Sci. 2011 , 29 , 994–1000. [ Google Scholar ]
- Özhan, Ş.Ç.; Kocadere, S.A. The effects of flow, emotional engagement, and motivation on success in a gamified online learning environment. J. Educ. Comput. Res. 2020 , 57 , 2006–2031. [ Google Scholar ] [ CrossRef ]
- Wang, A.P.; Che, H.S. A research on the relationship between learning anxiety, learning attitude, motivation and test performance. Psychol. Dev. Educ. 2005 , 21 , 55–59. [ Google Scholar ]
- Lin, C.H.; Zhang, Y.N.; Zheng, B.B. The roles of learning strategies and motivation in online language learning: A structural equation modeling analysis. Comput. Educ. 2017 , 113 , 75–85. [ Google Scholar ] [ CrossRef ]
- Deschênes, M.F.; Goudreau, J.; Fernandez, N. Learning strategies used by undergraduate nursing students in the context of a digital educational strategy based on script concordance: A descriptive study. Nurse Educ. Today 2020 , 95 , 104607. [ Google Scholar ] [ CrossRef ] [ PubMed ]
- Jerusalem, M.; Schwarzer, R. Self-efficacy as a resource factor in stress appraisal processes. In Self-Efficacy: Thought Control of Action ; Schwarzer, R., Ed.; Hemisphere Publishing Corp: Washington, DC, USA, 1992; pp. 195–213. [ Google Scholar ]
- Zimmerman, B.J. Becoming a self-regulated learner: An overview. Theory Pract. 2002 , 41 , 64–70. [ Google Scholar ] [ CrossRef ]
- Pintrich, P.R.; Smith, D.A.F.; García, T.; McKeachie, W.J. A Manual for the Use of the Motivated Strategies Questionnaire (MSLQ) ; University of Michigan, National Center for Research to Improve Post Secondary Teaching and Learning: Ann Arbor, MI, USA, 1991. [ Google Scholar ]
- Knowles, E.; Kerkman, D. An investigation of students attitude and motivation toward online learning. InSight Collect. Fac. Scholarsh. 2007 , 2 , 70–80. [ Google Scholar ] [ CrossRef ]
- Hair, J.F., Jr.; Black, W.C.; Babin, B.J.; Anderson, R.E. Multivariate Data Analysis: A Global Perspective , 7th ed.; Pearson Education International: Upper Saddle River, NJ, USA, 2010. [ Google Scholar ]
- Fornell, C.; Larcker, D.F. Evaluating structural equation models with unobservable variables and measurement error. J. Mark. Res. 1981 , 18 , 39–50. [ Google Scholar ] [ CrossRef ]
- Hair, J.F., Jr.; Hult, G.T.M.; Ringle, C.; Sarstedt, M. A Primer on Partial Least Squares Structural Equation Modeling (PLS-SEM) ; Sage: Los Angeles, CA, USA, 2016. [ Google Scholar ]
- Kiliç-Çakmak, E. Learning strategies and motivational factors predicting information literacy self-efficacy of e-learners. Aust. J. Educ. Technol. 2010 , 26 , 192–208. [ Google Scholar ] [ CrossRef ] [ Green Version ]
- Zheng, C.; Liang, J.C.; Li, M.; Tsai, C. The relationship between English language learners’ motivation and online self-regulation: A structural equation modelling approach. System 2018 , 76 , 144–157. [ Google Scholar ] [ CrossRef ]
- May, M.; George, S.; Prévôt, P. TrAVis to enhance students’ self-monitoring in online learning supported by computer-mediated communication tools. Int. J. Comput. Inform. Syst. Ind. Manag. Appl. 2011 , 3 , 623–634. [ Google Scholar ]
- Rafart, M.A.; Bikfalvi, A.; Soler, J.; Poch, J. Impact of using automatic E-Learning correctors on teaching business subjects to engineers. Int. J. Eng. Educ. 2019 , 35 , 1630–1641. [ Google Scholar ]
- Lee, P.M.; Tsui, W.H.; Hsiao, T.C. A low-cost scalable solution for monitoring affective state of students in E-learning environment using mouse and keystroke data. In Intelligent Tutoring Systems ; Cerri, S.A., Clancey, W.J., Papadourakis, G., Panourgia, K., Eds.; Springer: Berlin, Germany, 2012; pp. 679–680. [ Google Scholar ]
- Metz, D.; Karadgi, S.S.; Müller, U.J.; Grauer, M. Self-Learning monitoring and control of manufacturing processes based on rule induction and event processing. In Proceedings of the 4th International Conference on Information, Process, and Knowledge Management eKNOW, Valencia, Spain, 21–25 November 2012; pp. 78–85. [ Google Scholar ]
- Fitch, J.L.; Ravlin, E.C. Willpower and perceived behavioral control: Intention-behavior relationship and post behavior attributions. Soc. Behav. Pers. Int. J. 2005 , 33 , 105–124. [ Google Scholar ] [ CrossRef ]
- Sridharan, B.; Deng, H.; Kirk, J.; Brian, C. Structural equation modeling for evaluating the user perceptions of e-learning effectiveness in higher education. In Proceedings of the ECIS 2010: 18th European Conference on Information Systems, Pretoria, South Africa, 7–9 June 2010. [ Google Scholar ]
- Tarhini, A.; Hone, K.; Liu, X. The effects of individual differences on e-learning users’ behaviour in developing countries: A structural equation model. Comput. Hum. Behav. 2014 , 41 , 153–163. [ Google Scholar ] [ CrossRef ] [ Green Version ]
- de Leeuw, R.A.; Logger, D.N.; Westerman, M.; Bretschneider, J.; Plomp, M.; Scheele, F. Influencing factors in the implementation of postgraduate medical e-learning: A thematic analysis. BMC Med. Educ. 2019 , 19 , 300. [ Google Scholar ] [ CrossRef ] [ Green Version ]
- Erenler, H.H.T. A structural equation model to evaluate students’ learning and satisfaction. Comput. Appl. Eng. Educ. 2020 , 28 , 254–267. [ Google Scholar ] [ CrossRef ]
- Fee, K. Delivering E-learning: A complete strategy for design, application and assessment. Dev. Learn. Organ. 2013 , 27 , 40–52. [ Google Scholar ] [ CrossRef ]
- So, W.W.N.; Chen, Y.; Wan, Z.H. Multimedia e-Learning and self-regulated science learning: A study of primary school learners’ experiences and perceptions. J. Sci. Educ. Technol. 2019 , 28 , 508–522. [ Google Scholar ] [ CrossRef ]
Click here to enlarge figure
Variables | Category | Frequency | Percentage |
---|---|---|---|
Gender | Male | 243 | 51.81 |
Female | 226 | 48.19 | |
Education program level | Undergraduate program | 210 | 44.78 |
Master program | 154 | 32.84 | |
Doctoral program | 105 | 22.39 | |
Online learning tools | Smartphone | 255 | 54.37 |
Computer/PC | 125 | 26.65 | |
Tablet | 89 | 18.98 | |
Online learning media | Google Meet | 132 | 28.14 |
Microsoft Teams | 99 | 21.11 | |
Zoom | 196 | 41.79 | |
Others | 42 | 8.96 |
Construct | Measurement Items | Factor Loading/Coefficient (t-Value) | AVE | Composite Reliability | Cronbach’s Alpha |
---|---|---|---|---|---|
Online Learning Benefit (LBE) | LBE1 | 0.88 | 0.68 | 0.86 | 0.75 |
LBE2 | 0.86 | ||||
LBE3 | 0.71 | ||||
Online-learning effectiveness (LEF) | LEF1 | 0.83 | 0.76 | 0.90 | 0.84 |
LEF2 | 0.88 | ||||
LEF3 | 0.90 | ||||
Online-learning motivation (LMT) | LMT1 | 0.86 | 0.77 | 0.91 | 0.85 |
LMT2 | 0.91 | ||||
LMT3 | 0.85 | ||||
Online-learning strategies (LST) | LST1 | 0.90 | 0.75 | 0.90 | 0.84 |
LST2 | 0.87 | ||||
LST3 | 0.83 | ||||
Online-learning attitude (OLA) | OLA1 | 0.89 | 0.75 | 0.90 | 0.84 |
OLA2 | 0.83 | ||||
OLA3 | 0.87 | ||||
Online-learning confidence-in-technology (OLC) | OLC1 | 0.87 | 0.69 | 0.87 | 0.76 |
OLC2 | 0.71 | ||||
OLC3 | 0.89 | ||||
Online-learning monitoring (OLM) | OLM1 | 0.88 | 0.75 | 0.89 | 0.83 |
OLM2 | 0.91 | ||||
OLM3 | 0.79 | ||||
Online-learning self-efficacy (OLS) | OLS1 | 0.79 | 0.64 | 0.84 | 0.73 |
OLS2 | 0.81 | ||||
OLS3 | 0.89 | ||||
Online-learning willpower (OLW) | OLW1 | 0.91 | 0.69 | 0.87 | 0.77 |
OLW2 | 0.84 | ||||
OLW3 | 0.73 |
LBE | LEF | LMT | LST | OLA | OLC | OLM | OLS | OLW | |
---|---|---|---|---|---|---|---|---|---|
LBE | |||||||||
LEF | 0.82 | ||||||||
LMT | 0.81 | 0.80 | |||||||
LST | 0.80 | 0.84 | 0.86 | ||||||
OLA | 0.69 | 0.63 | 0.78 | 0.81 | |||||
OLC | 0.76 | 0.79 | 0.85 | 0.79 | 0.72 | ||||
OLM | 0.81 | 0.85 | 0.81 | 0.76 | 0.63 | 0.83 | |||
OLS | 0.71 | 0.59 | 0.69 | 0.57 | 0.56 | 0.69 | 0.75 | ||
OLW | 0.75 | 0.75 | 0.80 | 0.74 | 0.64 | 0.81 | 0.80 | 0.79 |
LBE | LEF | LMT | LST | OLA | OLC | OLM | OLS | OLW | |
---|---|---|---|---|---|---|---|---|---|
LBE1 | 0.88 | 0.76 | 0.87 | 0.66 | 0.54 | 0.79 | 0.78 | 0.63 | 0.74 |
LBE2 | 0.86 | 0.68 | 0.74 | 0.63 | 0.57 | 0.75 | 0.91 | 0.73 | 0.79 |
LBE3 | 0.71 | 0.54 | 0.59 | 0.71 | 0.63 | 0.55 | 0.50 | 0.36 | 0.53 |
LEF1 | 0.63 | 0.83 | 0.72 | 0.65 | 0.51 | 0.62 | 0.69 | 0.46 | 0.57 |
LEF2 | 0.77 | 0.88 | 0.78 | 0.71 | 0.55 | 0.73 | 0.78 | 0.52 | 0.69 |
LEF3 | 0.72 | 0.90 | 0.80 | 0.83 | 0.57 | 0.72 | 0.76 | 0.58 | 0.69 |
LMT1 | 0.88 | 0.76 | 0.87 | 0.66 | 0.54 | 0.79 | 0.78 | 0.63 | 0.74 |
LMT2 | 0.79 | 0.89 | 0.91 | 0.79 | 0.62 | 0.73 | 0.88 | 0.61 | 0.67 |
LMT3 | 0.72 | 0.65 | 0.85 | 0.77 | 0.89 | 0.72 | 0.67 | 0.59 | 0.69 |
LST1 | 0.61 | 0.63 | 0.68 | 0.90 | 0.78 | 0.64 | 0.57 | 0.39 | 0.57 |
LST2 | 0.74 | 0.59 | 0.72 | 0.87 | 0.78 | 0.68 | 0.61 | 0.48 | 0.63 |
LST3 | 0.72 | 0.90 | 0.80 | 0.83 | 0.57 | 0.72 | 0.76 | 0.58 | 0.69 |
OLA1 | 0.72 | 0.65 | 0.85 | 0.79 | 0.89 | 0.72 | 0.67 | 0.59 | 0.69 |
OLA2 | 0.51 | 0.48 | 0.55 | 0.59 | 0.83 | 0.58 | 0.47 | 0.42 | 0.43 |
OLA3 | 0.52 | 0.44 | 0.55 | 0.70 | 0.87 | 0.55 | 0.43 | 0.39 | 0.47 |
OLC1 | 0.78 | 0.70 | 0.73 | 0.65 | 0.53 | 0.87 | 0.77 | 0.65 | 0.91 |
OLC2 | 0.51 | 0.53 | 0.57 | 0.62 | 0.75 | 0.71 | 0.46 | 0.39 | 0.47 |
OLC3 | 0.81 | 0.73 | 0.78 | 0.69 | 0.55 | 0.89 | 0.80 | 0.66 | 0.75 |
OLM1 | 0.79 | 0.89 | 0.91 | 0.79 | 0.62 | 0.73 | 0.88 | 0.61 | 0.69 |
OLM2 | 0.86 | 0.68 | 0.74 | 0.63 | 0.57 | 0.75 | 0.91 | 0.73 | 0.79 |
OLM3 | 0.69 | 0.55 | 0.57 | 0.47 | 0.39 | 0.67 | 0.79 | 0.61 | 0.73 |
OLS1 | 0.41 | 0.23 | 0.35 | 0.28 | 0.39 | 0.41 | 0.40 | 0.69 | 0.49 |
OLS2 | 0.45 | 0.41 | 0.48 | 0.38 | 0.43 | 0.48 | 0.52 | 0.81 | 0.49 |
OLS3 | 0.75 | 0.66 | 0.72 | 0.60 | 0.49 | 0.69 | 0.77 | 0.89 | 0.82 |
OLW1 | 0.78 | 0.70 | 0.73 | 0.65 | 0.53 | 0.87 | 0.77 | 0.65 | 0.91 |
OLW2 | 0.75 | 0.65 | 0.71 | 0.59 | 0.51 | 0.69 | 0.77 | 0.87 | 0.84 |
OLW3 | 0.57 | 0.49 | 0.54 | 0.59 | 0.57 | 0.57 | 0.53 | 0.39 | 0.73 |
Hypothesis | Path | Standardized Path Coefficient | t-Value | Result |
---|---|---|---|---|
H1 | OLS → LST | 0.29 *** | 2.14 | Accepted |
H2 | OLM → LST | 0.24 *** | 2.29 | Accepted |
H3 | OLC → LST | 0.28 *** | 1.99 | Accepted |
H4 | OLC → LMT | 0.36 *** | 2.96 | Accepted |
H5 | OLW → LMT | 0.26 *** | 2.55 | Accepted |
H6 | OLA → LMT | 0.34 *** | 4.68 | Accepted |
H7 | LMT → LST | 0.71 *** | 4.96 | Accepted |
H8 | LMT → LEF | 0.60 *** | 5.89 | Accepted |
H9 | LST → LEF | 0.32 *** | 3.04 | Accepted |
H10 | LEF → LBE | 0.81 *** | 23.6 | Accepted |
MDPI stays neutral with regard to jurisdictional claims in published maps and institutional affiliations. |
Share and Cite
Hongsuchon, T.; Emary, I.M.M.E.; Hariguna, T.; Qhal, E.M.A. Assessing the Impact of Online-Learning Effectiveness and Benefits in Knowledge Management, the Antecedent of Online-Learning Strategies and Motivations: An Empirical Study. Sustainability 2022 , 14 , 2570. https://doi.org/10.3390/su14052570
Hongsuchon T, Emary IMME, Hariguna T, Qhal EMA. Assessing the Impact of Online-Learning Effectiveness and Benefits in Knowledge Management, the Antecedent of Online-Learning Strategies and Motivations: An Empirical Study. Sustainability . 2022; 14(5):2570. https://doi.org/10.3390/su14052570
Hongsuchon, Tanaporn, Ibrahiem M. M. El Emary, Taqwa Hariguna, and Eissa Mohammed Ali Qhal. 2022. "Assessing the Impact of Online-Learning Effectiveness and Benefits in Knowledge Management, the Antecedent of Online-Learning Strategies and Motivations: An Empirical Study" Sustainability 14, no. 5: 2570. https://doi.org/10.3390/su14052570
Article Metrics
Article access statistics, further information, mdpi initiatives, follow mdpi.
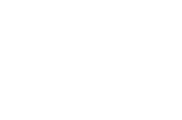
Subscribe to receive issue release notifications and newsletters from MDPI journals
How Effective Is Online Learning? What the Research Does and Doesn’t Tell Us

- Share article
Editor’s Note: This is part of a series on the practical takeaways from research.
The times have dictated school closings and the rapid expansion of online education. Can online lessons replace in-school time?
Clearly online time cannot provide many of the informal social interactions students have at school, but how will online courses do in terms of moving student learning forward? Research to date gives us some clues and also points us to what we could be doing to support students who are most likely to struggle in the online setting.
The use of virtual courses among K-12 students has grown rapidly in recent years. Florida, for example, requires all high school students to take at least one online course. Online learning can take a number of different forms. Often people think of Massive Open Online Courses, or MOOCs, where thousands of students watch a video online and fill out questionnaires or take exams based on those lectures.
In the online setting, students may have more distractions and less oversight, which can reduce their motivation.
Most online courses, however, particularly those serving K-12 students, have a format much more similar to in-person courses. The teacher helps to run virtual discussion among the students, assigns homework, and follows up with individual students. Sometimes these courses are synchronous (teachers and students all meet at the same time) and sometimes they are asynchronous (non-concurrent). In both cases, the teacher is supposed to provide opportunities for students to engage thoughtfully with subject matter, and students, in most cases, are required to interact with each other virtually.
Coronavirus and Schools
Online courses provide opportunities for students. Students in a school that doesn’t offer statistics classes may be able to learn statistics with virtual lessons. If students fail algebra, they may be able to catch up during evenings or summer using online classes, and not disrupt their math trajectory at school. So, almost certainly, online classes sometimes benefit students.
In comparisons of online and in-person classes, however, online classes aren’t as effective as in-person classes for most students. Only a little research has assessed the effects of online lessons for elementary and high school students, and even less has used the “gold standard” method of comparing the results for students assigned randomly to online or in-person courses. Jessica Heppen and colleagues at the American Institutes for Research and the University of Chicago Consortium on School Research randomly assigned students who had failed second semester Algebra I to either face-to-face or online credit recovery courses over the summer. Students’ credit-recovery success rates and algebra test scores were lower in the online setting. Students assigned to the online option also rated their class as more difficult than did their peers assigned to the face-to-face option.
Most of the research on online courses for K-12 students has used large-scale administrative data, looking at otherwise similar students in the two settings. One of these studies, by June Ahn of New York University and Andrew McEachin of the RAND Corp., examined Ohio charter schools; I did another with colleagues looking at Florida public school coursework. Both studies found evidence that online coursetaking was less effective.
About this series

This essay is the fifth in a series that aims to put the pieces of research together so that education decisionmakers can evaluate which policies and practices to implement.
The conveners of this project—Susanna Loeb, the director of Brown University’s Annenberg Institute for School Reform, and Harvard education professor Heather Hill—have received grant support from the Annenberg Institute for this series.
To suggest other topics for this series or join in the conversation, use #EdResearchtoPractice on Twitter.
Read the full series here .
It is not surprising that in-person courses are, on average, more effective. Being in person with teachers and other students creates social pressures and benefits that can help motivate students to engage. Some students do as well in online courses as in in-person courses, some may actually do better, but, on average, students do worse in the online setting, and this is particularly true for students with weaker academic backgrounds.
Students who struggle in in-person classes are likely to struggle even more online. While the research on virtual schools in K-12 education doesn’t address these differences directly, a study of college students that I worked on with Stanford colleagues found very little difference in learning for high-performing students in the online and in-person settings. On the other hand, lower performing students performed meaningfully worse in online courses than in in-person courses.
But just because students who struggle in in-person classes are even more likely to struggle online doesn’t mean that’s inevitable. Online teachers will need to consider the needs of less-engaged students and work to engage them. Online courses might be made to work for these students on average, even if they have not in the past.
Just like in brick-and-mortar classrooms, online courses need a strong curriculum and strong pedagogical practices. Teachers need to understand what students know and what they don’t know, as well as how to help them learn new material. What is different in the online setting is that students may have more distractions and less oversight, which can reduce their motivation. The teacher will need to set norms for engagement—such as requiring students to regularly ask questions and respond to their peers—that are different than the norms in the in-person setting.
Online courses are generally not as effective as in-person classes, but they are certainly better than no classes. A substantial research base developed by Karl Alexander at Johns Hopkins University and many others shows that students, especially students with fewer resources at home, learn less when they are not in school. Right now, virtual courses are allowing students to access lessons and exercises and interact with teachers in ways that would have been impossible if an epidemic had closed schools even a decade or two earlier. So we may be skeptical of online learning, but it is also time to embrace and improve it.
A version of this article appeared in the April 01, 2020 edition of Education Week as How Effective Is Online Learning?
Sign Up for EdWeek Tech Leader
Edweek top school jobs.
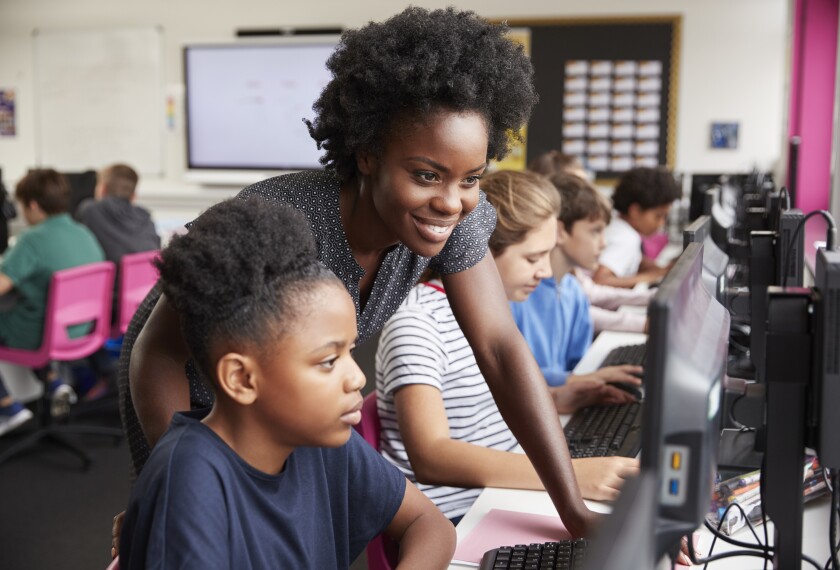
Sign Up & Sign In

- Share full article
Advertisement
Supported by
With Online Learning, ‘Let’s Take a Breath and See What Worked and Didn’t Work’
The massive expansion of online higher education created a worldwide laboratory to finally assess its value and its future.

By Jon Marcus
This article is part of our Learning special report about how the pandemic has continued to change how we approach education.
Kameshwari Shankar watched for years as college and university courses were increasingly taught online instead of face to face, but without a definitive way of understanding which students benefited the most from them, or what if anything they learned.
As an associate professor of economics at City College in New York, Dr. Shankar knew that one of the most important requirements of scientific research was often missing from studies of the effectiveness of online higher education: a control group.
Then came the Covid-19 pandemic, forcing almost everyone on earth online and creating a randomized trial on a planetary scale with a control group so big, it was a researcher’s wildest dream.
“The pandemic and the lockdown — that’s a great natural experiment,” said Dr. Shankar. A study she co-authored called it “a gold mine of evidence.”
Now the results of this experiment are starting to come in. They suggest that online higher education may work better than prepandemic research suggested, and that it is evolving decisively toward a combination of in-person and online, or “blended,” classes.
“For two years we’ve had sort of a petri dish of experimenting with learning online,” said Anant Agarwal, chief platform officer of the online program management company 2U and former CEO of edX, the online provider created by the Massachusetts Institute of Technology and Harvard and sold last year to 2U for $800 million. “Now people are sitting down and saying, ‘Let’s take a breath. Let’s see what worked and didn’t work.’ ”
We are having trouble retrieving the article content.
Please enable JavaScript in your browser settings.
Thank you for your patience while we verify access. If you are in Reader mode please exit and log into your Times account, or subscribe for all of The Times.
Thank you for your patience while we verify access.
Already a subscriber? Log in .
Want all of The Times? Subscribe .
Advertisement
Online learning in higher education: exploring advantages and disadvantages for engagement
- Published: 03 April 2018
- Volume 30 , pages 452–465, ( 2018 )
Cite this article
- Amber D. Dumford 1 &
- Angie L. Miller ORCID: orcid.org/0000-0002-5828-235X 2
63k Accesses
433 Citations
32 Altmetric
Explore all metrics
As the popularity of online education continues to rise, many colleges and universities are interested in how to best deliver course content for online learners. This study explores the ways in which taking courses through an online medium impacts student engagement, utilizing data from the National Survey of Student Engagement. Data was analyzed using a series of ordinary least squares regression models, also controlling for relevant student and institutional characteristics. The results indicated numerous significant relationships between taking online courses and student engagement for both first-year students and seniors. Those students taking greater numbers of online courses were more likely to engage in quantitative reasoning. However, they were less likely to engage in collaborative learning, student-faculty interactions, and discussions with diverse others, compared to their more traditional classroom counterparts. The students with greater numbers of online courses also reported less exposure to effective teaching practices and lower quality of interactions. The relationship between these engagement indicators and the percentage of classes taken online suggests that an online environment might benefit certain types of engagement, but may also be somewhat of a deterrent to others. Institutions should consider these findings when designing online course content, and encourage faculty to contemplate ways of encouraging student engagement across a variety of delivery types.
This is a preview of subscription content, log in via an institution to check access.
Access this article
Subscribe and save.
- Get 10 units per month
- Download Article/Chapter or Ebook
- 1 Unit = 1 Article or 1 Chapter
- Cancel anytime
Price includes VAT (Russian Federation)
Instant access to the full article PDF.
Rent this article via DeepDyve
Institutional subscriptions
Similar content being viewed by others
Instructional strategies to help online students learn: feedback from online students.
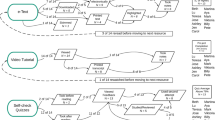
Graduate Students’ Use and Perceived Value of Learning Resources in Learning the Content in an Online Course
Improving Student Engagement, Retention and Success in Online Learning
Allen, E., & Seaman, J. (2013). Changing course: Ten years of tracking online education in the United States . Babson Park, MA: Babson Survey Research Group.
Google Scholar
Anaya, G. (1999). College impact on student learning: Comparing the use of self-reported gains, standardized test scores, and college grades. Research in Higher Education, 40, 499–526.
Article Google Scholar
Astin, A. W. (1993). What matters in college? Four critical years revisited . San Francisco, CA: Jossey-Bass.
Baird, L. (2005). College environments and climates: Assessments and their theoretical assumptions. Higher Education: Handbook of Theory and Research, 10, 507–537.
Braten, I., & Streomso, H. I. (2006). Epistemological beliefs, interest, and gender as predictors of Internet-based learning activities. Computers in Human Behavior, 22 (6), 1027–1042.
Cabrera, A. F., Crissman, J. L., Bernal, E. M., Nora, A., Terenzini, P. T., & Pascarella, E. T. (2002). Collaborative learning: Its impact on college students’ development and diversity. Journal of College Student Development, 43 (1), 20–34.
Chen, P. D., Lambert, A. D., & Guidry, K. R. (2010). Engaging online learners: The impact of web-based learning technology on student engagement. Computers & Education, 54, 1222–1232.
Cohen, V. L. (2003). Distance learning instruction: A new model of assessment. Journal of Computing in Higher Education, 14 (2), 98–120.
Dominguez, P. S., & Ridley, D. R. (2001). Assessing distance education courses and discipline differences in effectiveness. Journal of Instructional Psychology, 28 (1), 15–19.
Drysdale, J. S., Graham, C. R., Spring, K. J., & Halverson, L. R. (2013). An analysis of research trends in dissertations and theses studying blended learning. Internet and Higher Education, 17, 90–100. https://doi.org/10.1016/j.iheduc.2012.11.003 .
Evans, C. (2014). Twitter for teaching: Can social media be used to enhance the process of learning? British Journal of Educational Technology, 45 (5), 902–915.
Field, A. (2009). Discovering statistics using SPSS (3rd ed.). London: Sage Publications.
Friedman, J. (2014). Online education by discipline: A graduate student’s guide . Retrieved from http://www.usnews.com/education/online-education/articles/2014/09/17/online-education-by-discipline-a-graduate-students-guide .
Han, I., & Shin, W. S. (2016). The use of a mobile learning management system and academic achievement of online students. Computers & Education, 102, 79–89. https://doi.org/10.1016/j.compedu.2016.07.003 .
Hayek, J. C., Carini, R. M., O’Day, P. T., & Kuh, G. D. (2002). Triumph or tragedy: Comparing student engagement levels of members of Greek-letter organizations and other students. Journal of College Student Development, 43 (5), 643–663.
Henrie, C. R., Halverson, L. R., & Graham, C. R. (2015). Measuring student engagement in technology-mediated learning: A review. Computers & Education, 90, 36–53. https://doi.org/10.1016/j.compedu.2015.09.005 .
Hu, S., & Kuh, G. D. (2001). Computing experience and good practices in undergraduate education: Does the degree of campus ‘‘wiredness” matter? Education Policy Analysis Archives, 9 (49). http://epaa.asu.edu/epaa/v9n49.html .
Jacob, S., & Radhai, S. (2016). Trends in ICT e-learning: Challenges and expectations. International Journal of Innovative Research & Development, 5 (2), 196–201.
Junco, R. (2012). The relationship between frequency of Facebook use, participation in Facebook activities, and student engagement. Computers & Education, 58 (1), 162–171.
Junco, R., Elavsky, C. M., & Heiberger, G. (2013). Putting Twitter to the test: Assessing outcomes for student collaboration, engagement, and success. British Journal of Educational Technology, 44 (2), 273–287.
Junco, R., Heiberger, G., & Loken, E. (2010). The effect of Twitter on college student engagement and grades. Journal of Computer Assisted learning . https://doi.org/10.1111/j.1365-2729.2010.00387.x .
Kent, C., Laslo, E., & Rafaeli, S. (2016). Interactivity in online discussions and learning outcomes. Computers & Education, 97, 116–128. https://doi.org/10.1016/j.compedu.2016.03.002 .
Kim, K. J., & Bonk, C. J. (2006). The future of online teaching and learning in higher education: The survey says…. Educause Quarterly, 4, 22–30.
Kuh, G. D. (2001). The National Survey of Student Engagement: Conceptual framework and overview of psychometric properties . Bloomington, IN: Indiana University, Center for Postsecondary Research.
Kuh, G. D., & Hu, S. (2001a). The effects of student-faculty interaction in the 1990s. Review of Higher Education, 24 (3), 309–332.
Kuh, G. D., & Hu, S. (2001b). The relationships between computer and information technology use, student learning, and other college experiences. Journal of College Student Development, 42, 217–232.
McMillan, J. H., & Schumacher, S. (2001). Research in education: A conceptual introduction . New York: Longman.
Miller, A. L. (2012). Investigating social desirability bias in student self-report surveys. Educational Research Quarterly, 36 (1), 30–47.
Miller, A. L., Sarraf, S. A., Dumford, A. D., & Rocconi, L. M. (2016). Construct validity of NSSE engagement indicators (NSSE psychometric portfolio report). Bloomington, IN: Center for Postsecondary Research, Indiana University, School of Education. http://nsse.indiana.edu/pdf/psychometric_portfolio/Validity_ConstructValidity_FactorAnalysis_2013.pdf .
Moore, M. G., & Kearsley, G. (2011). Distance education: A systems view of online learning . Belmont, CA: Wadsworth.
Murayama, K., & Elliot, A. J. (2009). The joint influence of personal achievement goals and classroom goal structures on achievement-relevant outcomes. Journal of Educational Psychology, 101 (2), 432–447. https://doi.org/10.1037/a0014221 .
National Survey of Student Engagement. (2015). NSSE 2015 overview . Bloomington, IN: Indiana University, Center for Postsecondary Research.
Nelson Laird, T. F., Shoup, R., & Kuh, G. D. (2005). Measuring deep approaches to learning using the National Survey of Student Engagement . Paper presented at the annual meeting of the Association for Institutional Research, Chicago, IL. http://nsse.iub.edu/pdf/conference_presentations/2006/AIR2006DeepLearningFINAL.pdf .
Ormrod, J. E. (2011). Human learning (6th ed.). Upper Saddle River, NJ: Pearson.
Pace, C. R. (1980). Measuring the quality of student effort. Current issues in Higher Education, 2, 10–16.
Parsad, B., & Lewis, L. (2008). Distance education at degree-granting Postsecondary Institutions: 2006–2007 (NCES 2009–044). National Center for Education Statistics, Institute of Education Sciences. Washington, DC: US Department of Education. http://nces.ed.gov/pubs2009/2009044.pdf .
Pascarella, E. T., & Terenzini, P. T. (2005). How college affects students: A third decade of research (Vol. 2). San Francisco, CA: Jossey-Bass.
Pike, G. R. (1995). The relationship between self-reports of college experiences and achievement test scores. Research in Higher Education, 36 (1), 1–22.
Pintrich, P. R. (2004). A conceptual framework for assessing motivation and self-regulated learning in college students. Educational Psychology Review, 16 (4), 385–407. https://doi.org/10.1007/s10648-004-0006-x .
Pollack, P. H., & Wilson, B. M. (2002). Evaluating the impact of internet teaching: Preliminary evidence from American national government classes. PS. Political Science and Politics, 35 (3), 561–566.
Pukkaew, C. (2013). Assessment of the effectiveness of internet-based distance learning through the VClass e-Education platform. International Review of Research in Open and Distance Learning, 14 (4), 255–276.
Restauri, S. L., King, F. L., & Nelson, J. G. (2001). Assessment of students’ ratings for two methodologies of teaching via distance learning: An evaluative approach based on accreditation . ERIC document 460-148, reports-research (143).
Richardson, J. T. E., Morgan, A., & Woodley, A. (1999). Approaches to studying distance education. Higher Education, 37, 23–55.
Robinson, C. C., & Hullinger, H. (2008). New benchmarks in higher education: Student engagement in online learning. Journal of Education for Business, 84 (2), 101–108.
Serwatka, J. A. (2002). Improving student performance in distance learning courses. Technological Horizons in Education THE Journal, 29 (9), 48–51.
Shuey, S. (2002). Assessing online learning in higher education. Journal of Instruction Delivery Systems, 16, 13–18.
Stallings, D. (2002). Measuring success in the virtual university. The Journal of Academic Librarianship, 28, 47–53.
Tabachnick, B. G., & Fidell, L. S. (2001). Using multivariate statistics (4th ed.). Needham Heights, MA: Allyn & Bacon.
Tess, P. A. (2013). The role of social media in higher education classes (real and virtual)—A literature review. Computers in Human Behavior, 29 (3), A60–A68.
Thurmond, V., & Wambach, K. (2004). Understanding interactions in distance education: A review of the literature. International Journal of Instructional Technology & Distance Learning, 1 , 9–33. http://www.itdl.org/journal/Jan_04/article02.htm .
Tomei, L. A. (2006). The impact of online teaching on faculty load: Computing the ideal class size for online courses. Journal of Technology and Teacher Education, 14, 531–541.
Umbach, P. D. (2007). How effective are they? Exploring the impact of contingent faculty on undergraduate education. Review of Higher Education, 30 (2), 91–123. https://doi.org/10.1353/rhe.2006.0080 .
U.S. Department of Education, National Center for Education Statistics. (2016). Digest of education statistics, 2014 (NCES 2016-006), Table 311.15. Retrieved from https://nces.ed.gov/fastfacts/display.asp?id=80 .
Whitley, B. E. (2002). Principles of research in behavioral science (2nd ed.). New York, NY: Routlegde.
Wijekumar, K., Ferguson, L., & Wagoner, D. (2006). Problems with assessment validity and reliability in wed-based distance learning environments and solutions. Journal of Educational Multimedia and Hypermedia, 15 (2), 199–215.
Wojciechowski, A., & Palmer, L. B. (2005). Individual student characteristics: Can any be predictors of success in online classes? Online Journal of Distance Learning Administration, 8 (2), 13.
Wu, W., Wu, Y. J., Chen, C., Kao, H., & Lin, C. (2012). Review of trends from mobile learning studies: A meta-analysis. Computers & Education, 59, 817–827. https://doi.org/10.1016/j.compedu.2012.03.016 .
Xu, D., & Smith Jaggars, S. (2013). Adaptability to online learning: Differences across types of students and academic subject areas (CCRC Working Paper). New York, NY: Teachers College, Columbia University. Retrieved from http://ccrc.tc.columbia.edu/publications/adaptability-to-online-learning.html .
Zhou, L., & Zhang, D. (2008). Web 2.0 impact on student learning process. In K. McFerrin et al. (Eds.), Proceedings of society for information technology and teacher education international conference (pp. 2880–2882). Chesapeake, VA: AACE.
Download references
Author information
Authors and affiliations.
University of South Florida, 4202 E Fowler Ave, EDU 105, Tampa, FL, 33620, USA
Amber D. Dumford
Indiana University Bloomington, 1900 E. 10th St, Suite 419, Bloomington, IN, 47406, USA
Angie L. Miller
You can also search for this author in PubMed Google Scholar
Corresponding author
Correspondence to Angie L. Miller .
Ethics declarations
Conflict of interest.
The authors declare that they have no conflict of interest.
Rights and permissions
Reprints and permissions
About this article
Dumford, A.D., Miller, A.L. Online learning in higher education: exploring advantages and disadvantages for engagement. J Comput High Educ 30 , 452–465 (2018). https://doi.org/10.1007/s12528-018-9179-z
Download citation
Published : 03 April 2018
Issue Date : December 2018
DOI : https://doi.org/10.1007/s12528-018-9179-z
Share this article
Anyone you share the following link with will be able to read this content:
Sorry, a shareable link is not currently available for this article.
Provided by the Springer Nature SharedIt content-sharing initiative
- Online education
- Higher education
- Student engagement
- Find a journal
- Publish with us
- Track your research

University Administration
- Careers @ USA
- For Current Students
- Request Information
- Master of Occupational Therapy (MOT)
- Doctor of Occupational Therapy (OTD)
- Post-Professional Doctor of Occupational Therapy (PPOTD)
- Master of Health Administration (MHA)
- Doctor of Education (EdD)
- Graduate Certificates
- Clinical Orthopedic Residency (OCS)
- Orthopaedic Manual Physical Therapy Fellowship (OMPT)
- Continuing Professional Education (CPE)
- Doctor of Physical Therapy (DPT)
- Non-Degree Physical Therapy Online Courses
- Master of Science in Speech-Language Pathology (MS-SLP)
- Post-Graduate Nursing Certificates
- Master of Science in Nursing (MSN)
- Doctor of Nursing Practice (DNP)
- Browse All Degree-Programs
- Admissions & Aid Home
- Scholarships & Grants
- How to Apply
- Cost of Attendance
- Financial Aid
- Application Deadlines
- Academic Calendar
- Financial Aid FAQ
- Admissions FAQs
- Catalog/Handbook
- Our History
- Accreditation
- B Corp Certified
- Student Achievement Data
- Institutional Learning Outcomes (ILOs)
- Diversity & Inclusion
- San Marcos, California
- St. Augustine, Florida
- Miami, Florida
- Austin, Texas
- Dallas, Texas
- Our Faculty
- Board of Directors
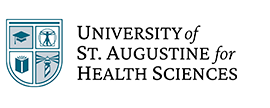
| 30 June 2020
Benefits of Online Learning – 16 Advantages to Learning Online

In fact, the pandemic has demonstrated what a practical, sustainable model online learning is. It keeps education accessible during a public health crisis, natural disaster, or other circumstance where students and faculty can’t travel. It can serve students across a wider geographic range, bringing in voices from across the country—and beyond. The online learning environment also promotes a better work-life balance. In this post, we outline the advantages of online learning and how you can leverage them to foster your personal and professional development.
Advantages of Online Learning
The format of online courses can help you thrive both personally and academically, helping you meet your goals.
1. You don’t need to relocate
With online classes, you don’t need to move to a different city or commute long distances in order to attend the program of your choice. You can stay where you are and keep your current job while you work toward enhancing your career with an online college or graduate degree (However, some programs require fieldwork experience, which may necessitate relocation.)
On the other hand, if you want to become a digital nomad—someone who embraces a location-independent, technology-enabled lifestyle—online education may allow you to do that too. You can watch lectures and complete your coursework wherever you are—whether that’s at home, in a café, or on an exotic beach.
2. It’s easier to achieve optimal school-life balance
Whether you’re a full-time or part-time online student, the online learning experience allows for a much more flexible schedule. Some elements may be synchronous: You may need to attend live lectures, demonstrations, or discussion sessions. But many elements will be asynchronous, meaning that you can complete them at your own pace, learning at the time of day and in the place that works best for you.
When you’re more in control of your schedule, time management goes more smoothly. It’s easier to maintain an active social and family life while earning your college degree or graduate degree. It is possible to have it all: education, professional development, and a vibrant personal life.
3. It helps you develop and stick to healthy habits

Another benefit of online learning and a better school-life balance is that you can develop greater discipline about healthy habits. Not everyone enjoys squeezing in a workout or yoga session at the crack of dawn or right before bed. So if you’re learning from home, you can take a midday jog or online Pilates class. You can even take a power nap, which research has shown can benefit memory and learning .
Studying from home can also give you more time to focus on self-care. Whether through self-reflection , meditation, or yoga, practicing self-care can help boost your confidence and emotional intelligence.
4. You have more time to do the things you love
If you’re taking in-person classes but don’t live near campus, commuting can take up a significant amount of your time. According to the U.S. Census Bureau , the average daily commute time is 54.2 minutes. If you’re like most people, you’d probably rather avoid traffic or crowded public transportation. And saving that 54 minutes per day (or more) will free up time you can spend working, exercising, having fun, or connecting with loved ones. In fact, spending time away from work has been shown to ultimately boost productivity and creativity .
5. Online learning makes it possible to keep your job
Many graduate students can’t afford to take time off from work. Or they want to keep working on career advancement while staying in their current position, improving their credentials and applying their learning on the job. So if you want to continue working at your current job while earning your degree, online education is a great way to go. You don’t have to choose between school and work—you can do both.
6. You can create your own office
It’s an advantage to be able to create your own workspace. You can set up your desk at just the right height and find a supportive office chair—or you can work from your couch. Not only can your setup be more physically comfortable, but studies show that 42% of workers believe they’re more productive working at home.
7. It’s easier to concentrate and participate
Since different people are productive at different times of day, online learning allows you to work at the time when your brain is most engaged. Also, some people have trouble concentrating and participating in crowded classrooms. Online learning can make it easier for students to focus and for more introverted students to chime in with questions or ideas, which can help boost their confidence and positivity .
Skills and Positive Traits Gained from Online Learning

8. Boost your time management skills
Staying organized is key to being a successful student. Find a method that works well for you, such as exploring these time management techniques , using planners , or trying out new virtual tools for productivity .
9. Learn new technical skills
Immersing yourself in the technology that powers online learning will enhance your technical literacy.
10. Enhance your communication skills
Online learning creates opportunities to interact, participate, and communicate with your peers and instructors in different ways.
11. Develop self-motivation and self-discipline
In order to stick to a schedule and keep up with your coursework, you must learn self-motivation and self-discipline—qualities that will serve you well in your personal and professional life.
Additional Benefits of Online Learning
12. reduce your carbon footprint .
Not only do you save money on gas, which averages out to $1,300 per person annually in the United States—you can save paper by lessening your reliance on printed materials, ultimately reducing your carbon footprint.
13. Reduce stress
Learning at your own pace, participating in ways that feel more comfortable, and not having an arduous commute can help you limit stress.
14. Cook at home more instead of eating out
Spending more time at home makes cooking easier, which is ideal for healthy eating and saving money.
15. Expand your horizons
Connecting with classmates from all around the world can help you share different cultural perspectives on your field, which makes you a more broad-minded practitioner.
16. Network with your peers
Meeting classmates online can give you opportunities to network across a wider geographic region, which can help you advance your career. You might even find a mentor who can help support and guide you to apply your learning on the job.

Forbes Business Council. “3 Benefits of Remote Learning for Every Generation.” Forbes, 14 Oct. 2022, https://www.forbes.com/sites/forbesbusinesscouncil/2022/10/14/3-benefits-of-remote-learning-for-every-generation/?sh=3d7184e0528e . Accessed: March 20, 2024.
Cavanaugh, Joseph K. and Stephen J. Jacquemin. “A Large Sample Comparison of Grade Based Student Learning Outcomes in Online vs. Face-to-Face Courses.” Online Learning 19, no. 2 (February 2015). http://dx.doi.org/10.24059/olj.v19i2.454. Accessed: January 26, 2022
Shaw, Gabby. “Here’s How Much the Average Person Spends on Gas in Every State.” Business Insider. February 15, 2019. https://www.businessinsider.com/how-much-the-average-person-spends-on-gas-in-every-state-2019-2. Accessed: January 26, 2022
ManpowerGroup. “Closing the Skills Gap: Know What Workers Want.” 2019. https://workforce-resources.manpowergroup.com/closing-the-skills-gap-know-what-workers-want/closing-the-skills-gap-know-what-workers-want. Accessed: January 26, 2022
Levitz, Ruffalo Noel. “2018 National Student Satisfaction and Priorities Report.” 2018. http://learn.ruffalonl.com/rs/395-EOG-977/images/2018_National_Student_Satisfaction_Report_EM-031.pdf. Accessed: January 26, 2022
Roy, Robin, Stephen Potter, Karen Yarrow, and Mark Smith. “Towards Sustainable Higher Education: Environmental impacts of campus-based and distance higher education systems.” Design Innovation Group. March 2005. http://www3.open.ac.uk/events/3/2005331_47403_o1.pdf. Accessed: January 26, 2022
West, Robin L., Natalie C. Ebner, and Erin C. Hastings. “Linking Goals and Aging.” In New Developments in Goal Setting and Task Performance, edited by Edwin A. Locke and Gary P. Latham, 439–459. Routledge Taylor and Francis Group, 2013. https://www.researchgate.net/profile/Natalie-Ebner/publication/287491758_Linking_goals_and_aging_Experimental_and_life-span_approaches/links/56773c0008ae502c99d2f10a/Linking-goals-and-aging-Experimental-and-life-span-approaches.pdf. Accessed: January 26, 2022
ARE YOU INSPIRED?
There could be an article about you here one day. Take charge of your own life-story!
Take charge of your own life-story
More Editorial Articles

How to Become a Physical Therapist in 6 Steps

How To Study Effectively? 10 Best Study Techniques | USAHS

Occupational Therapy vs. Physical Therapy
Upcoming editorial events.
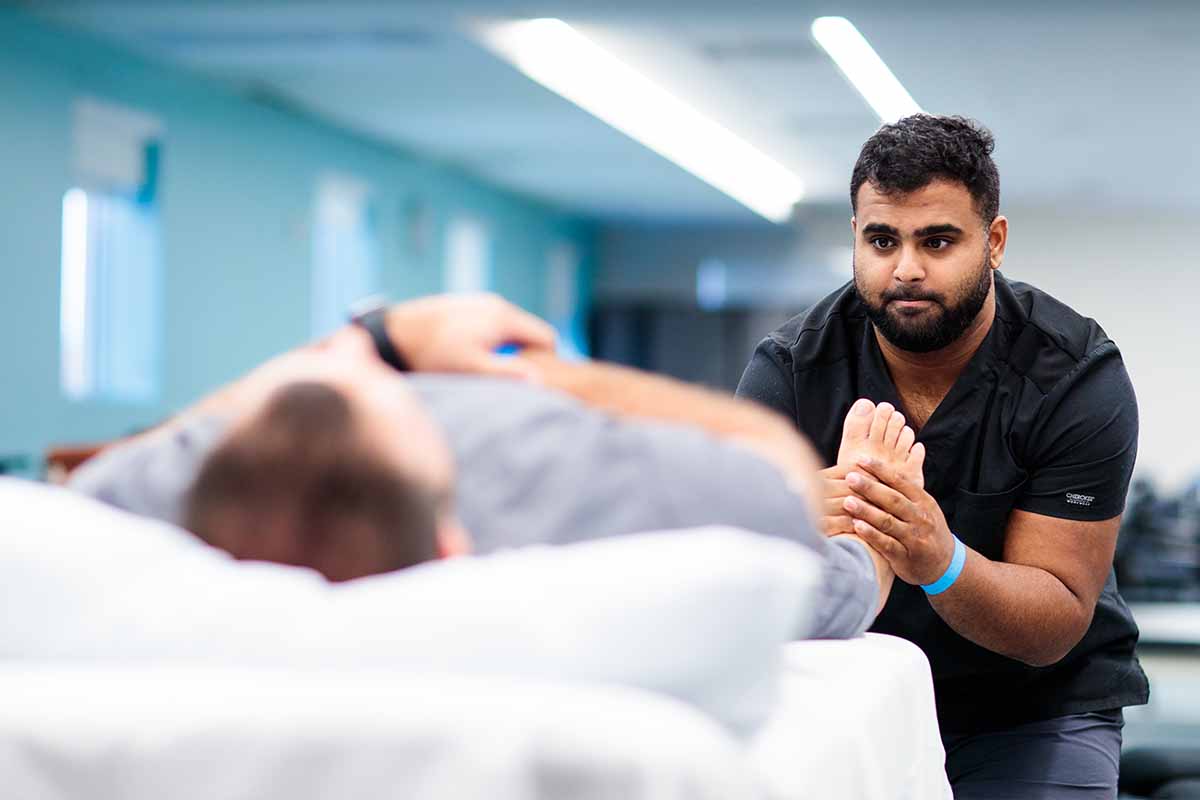
Doctor of Physical Therapy (DPT) Academic Webinar - July 15| USAHS
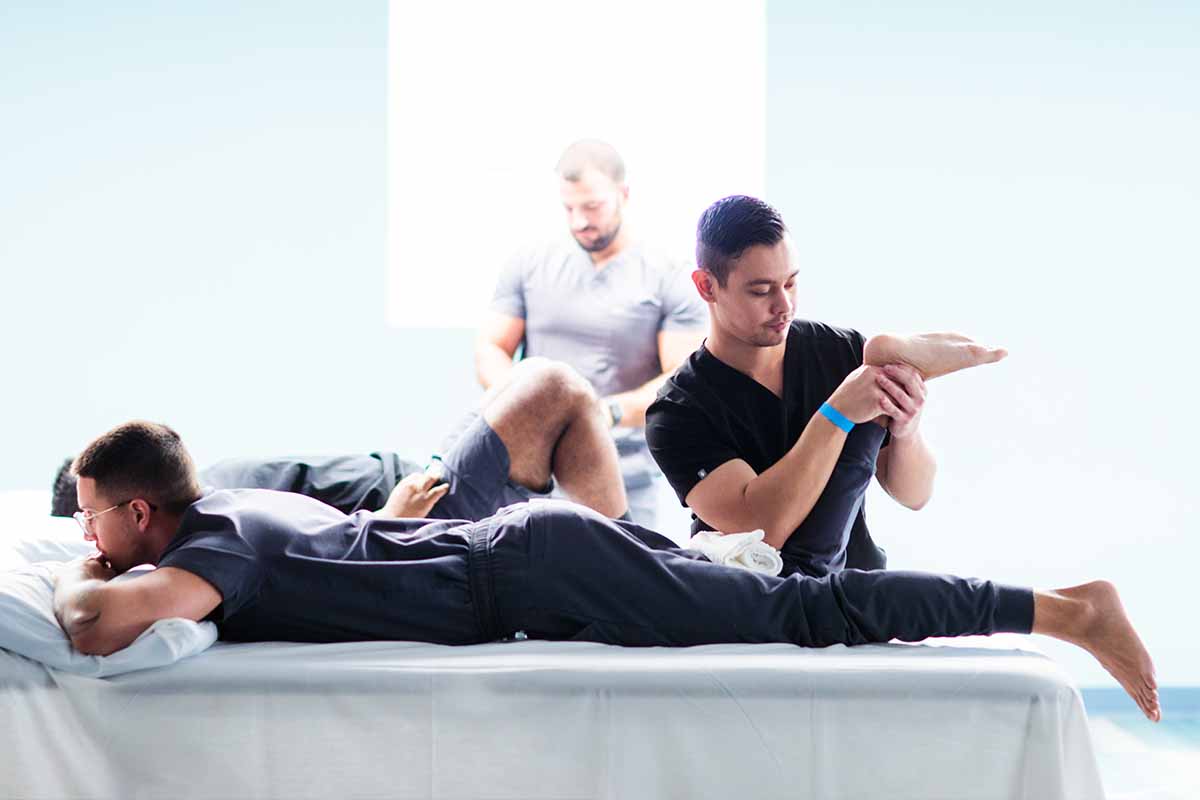
Flex Doctor of Physical Therapy (Flex DPT) - Academic Webinar - July 16| USAHS
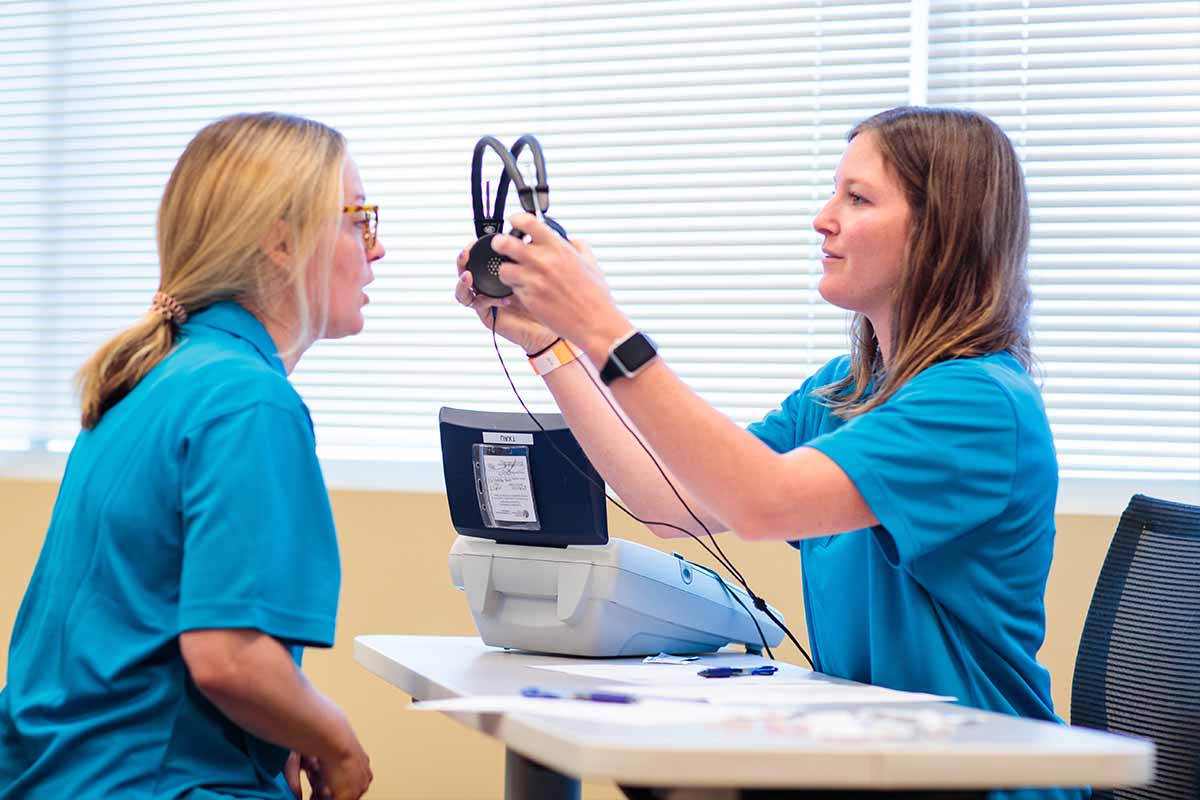
Master of Science in Speech-Language Pathology (MS-SLP) - Academic Webinar - July 17 | USAHS
ADVANTAGES AND DISADVANTAGES OF ONLINE LEARNING
- January 2022
- This person is not on ResearchGate, or hasn't claimed this research yet.

- Istinye Universitesi
Discover the world's research
- 25+ million members
- 160+ million publication pages
- 2.3+ billion citations
- Adi Idham Jailani
- Sumarni Maulan

- Umesh Acharya

- Allen Kitchel

- Sally E. Arnett
- AM J DRUG ALCOHOL AB

- Miriam Taylor

- Asma’ Fauzi
- Dairabi Kamil

- Natalya Alekseevna Zavyalova
- Anastasiya Igorevna Schemerova
- Manijeh Sadeghi

- J Wellington
- D B Ghoshal
- Recruit researchers
- Join for free
- Login Email Tip: Most researchers use their institutional email address as their ResearchGate login Password Forgot password? Keep me logged in Log in or Continue with Google Welcome back! Please log in. Email · Hint Tip: Most researchers use their institutional email address as their ResearchGate login Password Forgot password? Keep me logged in Log in or Continue with Google No account? Sign up
- Skip to main content
- Skip to main navigation
Engagement in Online Learning
Online learning has many advantages, such as quick access to material and convenience of taking classes at any location (Sun and Rueda, 2012; Simamora, 2020). But online learning presents challenges for student engagement, that is, the types and levels of effort students put into perform well and achieve their goals (Hu & Kuh, 2002; Richardson, Long & Foster, 2004). Students might lose the opportunities to interact, collaborate, and receive feedback from their instructors if they are not physically present in the classroom. But with thoughtful teaching practices, instructors can improve students’ engagement in online learning.
Online engagement strategies
Student-instructor strategies.
Research has shown many helpful strategies to maintain students’ engagement in online learning. For instance, students rate regular announcements, email reminders, and grading rubrics as the most beneficial strategies that instructors could incorporate in online classes (Bolliger and Martin, 2018). At Baylor, instructors can use Canvas options scheduling announcements for deadlines for major assignments.
Relatedly, Ryle and Cumming (2007) recommend posting welcome messages and announcements to establish expectations and set the tone for the course, as well as posting discussion topics ahead of time to stimulate interest and provide necessary resources.
Moreover, instructors can encourage student participation and learning by modeling online behaviors and establishing presence by participating in and facilitating online discussions. Instructors can also improve students’ sense of community by providing multiple communication channels, support and encouragement, and timely feedback, as well as by setting course expectations (Martin and Ertzberger, 2018).
Relatedly, previous research has indicated that certain online activities and tools, such as discussion boards, online debates and brainstorming, can potentially facilitate online student self-regulation in the learning process (Kanuka, 2005).
Student-student engagement strategies
A variety of strategies can be used to initiate and support learner-to-learner interaction and engagement. Building interactive introduction activities at the start of a course can improve learners’ engagement. This also increases the learner’s feelings of belonging (Stepich and Ertmer, 2003) and sense of community . For example, students could use online delivery platforms and other technologies to share photos and other personal media artifacts to explain their backgrounds and interests in ways that allow their fellow community of learners to comment and engage in conversations about shared interests and experiences. Research also supports icebreakers and collaborative work as the most beneficial engagement strategy expressed by the learners (Martin and Bolliger, 2018).
Student-content engagement strategies
Many factors can influence students’ engagement with course content. These factors, according to Vrasidas and Mclsaac (1999), are course structure, class size, students’ prior experiences with online courses, and feedback. The strategies for engaging students with the course content include providing structured discussions, assigning realistic scenarios (e.g., case studies, reports, research papers, and presentations), and providing more than one type of media interaction (e.g., text, video, audio, interactive games, and online resources; Su, Bonk, Magjuka, Liu, & Lee, 2005). Moreover, students should be provided with time and opportunities to reflect on course elements (e.g., use of communication tools, and their learning).
Previous research has shown that online activities and tools such as multimedia and discussion boards may increase engagement in online learning. This is salient for students who take online courses for the first time. Educators should identify students who are taking online courses for the first time and provide necessary technical help to increase their engagement and to offer students strategies for increasing their self-regulation in distance education environments. Previous research has also indicated that multimedia increases students’ situational interest ( that is, the “appealing effect of characteristics of an activity or a learning task on students.” Chen et al, 1999 p. 158), number of interactions, participation, and motivation (Guzley, Avanzino & Bor, 2001).
Relatedly, in online learning students can be engaged with the course content through asynchronous classes (wherein students learn at different times) and synchronous classes (wherein students learn at the same time). Examples for the former includes email, screencasts, and Flipgrid videos. Instructors can also benefit from the time-locked modules on Canvas and embedded quizzes in video lectures for asynchronous classes. Examples for the latter entail video conferencing, live chat, and live streamed videos wherein the instructors can use breakout room for students and assign them some discussion activities.
Recommendations to instructors for designing the course content:
- Design course content so that students are aware of the expectations and can easily navigate the course materials (Donham, Pohan, Menke, & Kranzfelder, 2022).
- Divide the course content into weekly modules, including an introductory module (or module 0). An introductory module establishes a framework for introducing yourself to your students and explaining why you are so passionate about the discipline and course (Davis, 2018).
- Post structured online discussion boards. Since discussion boards are commonly used in hybrid learning, such as flipped classrooms, to introduce students to course topics, the key is to make them meaningful, relevant, and instructive so that students see them as essential to the learning process rather than busywork.
- Align the assignments with course learning outcomes and assessments since they would positively affect the student’s motivation and engagement (Tharayil et al., 2018).
- Compile time-sensitive communications (e.g., time-released modules) into a weekly announcement sent on the same day each week. This helps the sense of connectedness and have clarity regarding expectations.
- Do regular “check-ins” at the beginning of class and/or provide a few minutes of silence throughout lectures to help keep students calm and focused on learning (Chick, 2013).
- Try to understand the students’ unique situations and remove deficit language from course material, such as harsh grading and attendance policies in the syllabus (see Inclusive Teaching ).
- Be available (e.g., Zoom office hours), supportive, and flexible.
- Incorporate introductory videos and recorded lectures in each module because these provide students with flexibility in their learning (Donham et al., 2022).
- Begin or expand the use of prerecorded lectures (wherein you can incorporate embedded quizzes on Canvas), which give students more opportunities to pause and rewatch the recording. This would assist students in taking and/or checking the adequacy of their course notes, processing the content more deeply (e.g., elaboration and reflection), and referring to the lecture if something was unclear or confusing. Prerecorded lecture videos can help students reduce cognitive load by allowing them to watch the videos multiple time at their own pace (Esson, 2016).
Bolliger, D. U., & Martin, F. (2018). Instructor and student perceptions of online student engagement strategies. Distance Education , 39 (4), 568-583.
Chen, A., Darst, P. W., & Pangrazi, R. P. (1999). What constitutes situational interest? Validating a construct in physical education. Measurement in physical education and exercise science , 3 (3), 157-180.
Chick N. 2013. Teaching in times of crisis. Center for Teaching, Vanderbilt University, Nashville, TN
Davis G. 2018. Creating module introduction videos. Wiley Education Services, Oak Brook, IL.
Donham, C., Pohan, C., Menke, E., & Kranzfelder, P. (2022). Increasing student engagement through course attributes, community, and classroom technology: lessons from the pandemic. Journal of Microbiology & Biology Education , e00268-21.
Esson JM. (2016). Flipping general and analytical chemistry at a primarily undergraduate institution, p 107–125. In Muzyka JL, Luker CS (ed), The flipped classroom , volume 2: results from practice. ACS Publications,Washington, DC.
Guzley, R., Avanzino, S., & Bor, A. (2001). Simulated computer-mediated/video-interactive distance learning: A test of motivation, interaction satisfaction, delivery, learning & perceived effectiveness. Journal of Computer-Mediated Communication , 6 (3), JCMC633.
Hu, S., & Kuh, G. D. (2002). Being (dis) engaged in educationally purposeful activities: The influences of student and institutional characteristics. Research in higher education , 43 (5), 555-575.
Kanuka, H. (2005). An exploration into facilitating higher levels of learning in a text-based internet learning environment using diverse instructional strategies. Journal of Computer-Mediated Communication , 10 (3), JCMC1032.
Martin, F., & Ertzberger, J. (2016). Effects of reflection type in the here and now mobile learning environment. British Journal of Educational Technology, 47 (5) , 932–944.
Richardson, J. T., Long, G. L., & Foster, S. B. (2004). Academic engagement in students with a hearing loss in distance education. Journal of Deaf Studies and Deaf Education , 9 (1), 68-85.
Ryle, A., & Cumming, K. (2007). Reflections on engagement in online learning communities. International Journal of Pedagogies and Learning , 3 (3), 35-46.
Simamora, R. M. (2020). The Challenges of online learning during the COVID-19 pandemic: An essay analysis of performing arts education students. Studies in Learning and Teaching , 1 (2), 86-103.
Stepich, D. A., & Ertmer, P. A. (2003). Building community as a critical element of online course design. Educational Technology , 33-43.
Su, B., Bonk, C. J., Magjuka, R. J., Liu, X., & Lee, S. H. (2005). The importance of interaction in web-based education: A program-level case study of online MBA courses. Journal of interactive online learning , 4 (1), 1-19.
Sun, J. C. Y., & Rueda, R. (2012). Situational interest, computer self-efficacy and self-regulation: Their impact on student engagement in distance education. British journal of educational technology , 43 (2), 191-204.
Tharayil S, Borrego M, Prince M, Nguyen KA, Shekhar P, Finelli CJ, Waters C. (2018). Strategies to mitigate student resistance to active learning. Int J STEM Educ 5:7.
Vrasidas, C. McIsaac, m. S.(1999). Factors influencing interaction in an online course. The American Journal of Distance Education , 13 (3).

Academy for Teaching and Learning
Moody Library, Suite 201
One Bear Place Box 97189 Waco, TX 76798-7189
- General Information
- Academics & Research
- Administration
- Gateways for ...
- About Baylor
- Give to Baylor
- Pro Futuris
- Social Media
- College of Arts & Sciences
- Diana R. Garland School of Social Work
- George W. Truett Theological Seminary
- Graduate School
- Hankamer School of Business
- Honors College
- Louise Herrington School of Nursing
- Research at Baylor University
- Robbins College of Health and Human Sciences
- School of Education
- School of Engineering & Computer Science
- School of Music
- University Libraries, Museums, and the Press
- More Academics
- Compliance, Risk and Safety
- Human Resources
- Marketing and Communications
- Office of General Counsel
- Office of the President
- Office of the Provost
- Operations, Finance & Administration
- Senior Administration
- Student Life
- University Advancement
- Undergraduate Admissions
- Graduate Admissions
- Baylor Law School Admissions
- Social Work Graduate Programs
- George W. Truett Theological Seminary Admissions
- Online Graduate Professional Education
- Virtual Tour
- Visit Campus
- Alumni & Friends
- Faculty & Staff
- Prospective Faculty & Staff
- Prospective Students
- Anonymous Reporting
- Annual Fire Safety and Security Notice
- Cost of Attendance
- Digital Privacy
- Legal Disclosures
- Mental Health Resources
- Web Accessibility
Thank you for visiting nature.com. You are using a browser version with limited support for CSS. To obtain the best experience, we recommend you use a more up to date browser (or turn off compatibility mode in Internet Explorer). In the meantime, to ensure continued support, we are displaying the site without styles and JavaScript.
- View all journals
- Explore content
- About the journal
- Publish with us
- Sign up for alerts
- Open access
- Published: 13 July 2024
Exploring the impact of artificial intelligence on higher education: The dynamics of ethical, social, and educational implications
- Abdulrahman M. Al-Zahrani ORCID: orcid.org/0009-0007-9885-0730 1 &
- Talal M. Alasmari ORCID: orcid.org/0000-0002-3330-1980 1
Humanities and Social Sciences Communications volume 11 , Article number: 912 ( 2024 ) Cite this article
247 Accesses
7 Altmetric
Metrics details
- Science, technology and society
The increasing prevalence of Artificial Intelligence (AI) in higher education underscores the necessity to explore its implications on ethical, social, and educational dynamics within the sector. This study aims to comprehensively investigate the impact of AI on higher education in Saudi Arabia, delving into stakeholders’ attitudes, perceptions, and expectations regarding its implementation. The research hones in on key facets of AI in higher education, encompassing its influence on teaching and learning, ethical and social implications, and the anticipated role of AI in the future. Employing a quantitative approach through an online survey questionnaire ( N = 1113), this study reveals positive attitudes toward AI in higher education. Stakeholders recognize its potential to enhance teaching and learning, streamline administration, and foster innovation. Emphasis is placed on ethical considerations and guidelines for AI implementation, highlighting the imperative need to address issues such as privacy, security, and bias. Participants envision a future characterized by personalized learning experiences, ethically integrated AI, collaboration, and ongoing support for lifelong learning. Furthermore, the results illuminate the intricate interplay between AI usage, purposes, difficulties, and their impact on attitudes, perceptions, and future implications. Accordingly, the research underscores the necessity for a comprehensive understanding of AI integration, considering not only its technical aspects but also the ethical, social, and educational dimensions. By acknowledging the role of AI uses, AI usage purposes, and addressing associated difficulties, educational stakeholders can work towards harnessing the benefits of AI while ensuring responsible and effective implementation in teaching and learning contexts.
Similar content being viewed by others
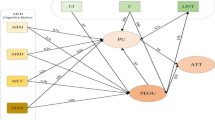
An explanatory study of factors influencing engagement in AI education at the K-12 Level: an extension of the classic TAM model
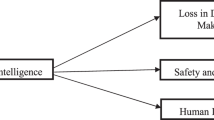
Impact of artificial intelligence on human loss in decision making, laziness and safety in education
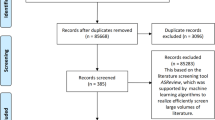
The dilemma and countermeasures of educational data ethics in the age of intelligence
Introduction.
Artificial Intelligence (AI) has become a transformative force, reshaping various industries such as communication systems, software applications, data storage, business operations, analytics, interactive platforms, cybersecurity, and social media (Al-Zahrani, 2023 ; Creely, 2022 ; Doncieux et al. 2022 ). Recent advancements in AI have profoundly impacted multiple aspects of life, including education and business. These advancements have fundamentally altered how we think, learn, operate, and thrive in an increasingly intelligent and interconnected world (Doncieux et al. 2022 ; Hassan et al. 2022 ; Jiang and Pang, 2023 ; Kuo et al. 2021 ; Vendraminelli et al. 2022 ; Zheng et al. 2023 ). Molenaar ( 2022 ) notes an ongoing fusion between humans and AI, resulting in the emergence of hybrid systems.
AI technologies have the potential to personalize learning experiences, automate administrative tasks, reduce workloads, offer instant feedback, tailor courses to individual progress, enhance student engagement, and optimize decision-making (Al-Zahrani, 2024a ; Chu et al. 2022 ; Dai and Ke, 2022 ; McKinsey and Company, 2022 ; UNESCO, 2021 ; Zheng et al. 2023 ). While the adoption of AI-powered tools in higher education initially progressed slowly, educators anticipate a future increase in their usage (McKinsey and Company, 2022 ).
In the realm of higher education, AI holds promise for addressing significant challenges and driving innovation in teaching and learning practices (Al-Zahrani, 2023 ; Al-Zahrani, 2024a ; Chu et al. 2022 ; Dai and Ke, 2022 ; McKinsey and Company, 2022 ; UNESCO, 2021 ). Educational settings, increasingly embracing AI technologies, include intelligent tutoring systems, adaptive learning platforms, chatbots, automated grading systems, and data analytics tools (Hassan et al. 2022 ; Jiang and Pang, 2023 ; Kuo et al. 2021 ; Vendraminelli et al. 2022 ; Zhao et al. 2022 ). To unlock these potential benefits, stakeholders in higher education and beyond must possess fundamental AI literacy to interact effectively with AI technology (Laupichler et al. 2022 ).
However, alongside these benefits, AI in higher education introduces several challenges and concerns, such as data privacy and security (Al-Zahrani, 2024b ; Carmody et al. 2021 ; Elliott and Soifer, 2022 ), algorithmic bias and discrimination (Bigman et al. 2022 ; Johnson, 2021 ; Kordzadeh and Ghasemaghaei, 2021 ; Wang et al. 2022 ), and various ethical considerations (Farisco et al. 2020 ; Kerr et al. 2020 ; Owe and Baum, 2021 ; Resseguier and Rodrigues, 2020 ; Ryan and Stahl, 2020 ; Stahl et al. 2021 ).
The research on AI in higher education is a dynamic and ever-evolving field. As AI gains prominence, it becomes essential to explore its impact on the educational, ethical, and social dynamics of the sector (e.g., Al-Zahrani, 2024b ; Chu et al. 2022 ; Dai and Ke, 2022 ; Zawacki-Richter et al. 2019 ). A significant knowledge gap exists regarding how various stakeholders in higher education, including students, faculty, and administrators, perceive the use of AI and envision its future role in the sector. Therefore, this study aims to thoroughly examine and understand the impact of AI on higher education, focusing on the following key aspects:
Attitudes and perceptions of students, faculty, and administrators: Investigating their attitudes, beliefs, and perspectives towards AI in higher education, exploring their concerns and expectations.
Impact on teaching and learning: Exploring how AI influences teaching and learning processes, assessing potential benefits and drawbacks in enhancing instructional approaches, personalizing learning experiences, and improving academic outcomes.
Ethical and social implications: Examining ethical considerations and social implications arising from AI implementation, including data privacy, algorithmic bias, fairness, transparency, and accountability.
Envisioned role of AI: Gaining insights into stakeholders’ visions for the future role of AI in higher education, exploring their expectations, aspirations, and concerns regarding integration and expansion.
By addressing these objectives, the study contributes to the understanding of AI’s impact on higher education and sheds light on the ethical, social, and educational dynamics that emerge. The research questions provide a framework to explore various aspects of AI in higher education, aligning with the study’s aim and scope:
RQ1: What are participants’ attitudes and perceptions towards the implementation of AI in higher education?
RQ2: What is the role of AI in teaching and learning in higher education?
RQ3: What ethical and social implications arise from the implementation of AI in higher education?
RQ4: How do participants envision the future role of AI in higher education?
RQ5: How do participants’ demographic characteristics impact their perspectives in terms of the ethical, social, and educational dynamics associated with AI implementation?
The remainder of this manuscript is organized as follows: Literature review presents a comprehensive review of the existing literature, covering key research areas related to AI in higher education, including pedagogical innovations, learning analytics and student support, assessment and grading, educators’ professional development, and ethical and social implications. Significance and novelty of the research describes the methodological approach employed in this study, detailing the research design, data collection procedures, and analytical methods. Methodology sheds light on the significance and novelty of this study, highlighting its holistic and comprehensive investigation of the multifaceted impact of AI on higher education. Results presents the study’s findings, organized according to the research questions, offering insights into participants’ attitudes and perceptions, the role of AI in teaching and learning, ethical and social implications, and envisioned future roles. Discussion provides a detailed discussion of the findings, situating them within the broader context of AI in higher education and drawing connections to existing literature. The final two sections (Conclusions and implications & Limitations and future research) conclude the manuscript by highlighting the study’s contributions, implications, limitations, and recommendations for future research.
Literature review
Research examining the impact of AI on higher education has witnessed substantial growth in recent years, as highlighted by notable studies (Al-Zahrani, 2023 ; Al-Zahrani, 2024a ; Bozkurt et al. 2021 ; Chu et al. 2022 ; Dai and Ke, 2022 ; Laupichler et al. 2022 ; Zawacki-Richter et al. 2019 ). Scholars from diverse fields, including education, computer science, psychology, and ethics, have explored various facets of AI implementation in higher education settings. Chu et al. ( 2022 ) scrutinized the top 50 AI studies in higher education from the Web of Science (WoS) database. Their analysis revealed a prevalent focus on predicting learners’ learning status, particularly dropout and retention rates, student models, and academic achievement. However, there is a noticeable lack of emphasis on higher-order thinking skills, collaboration, communication, self-efficacy, and AI skills in higher education studies (Chu et al. 2022 ). Laupichler et al. ( 2022 ) stress that research on AI in higher education is still in its early stages, necessitating refinement in defining AI literacy and determining appropriate content for non-experts to enhance their understanding of AI. This literature review provides an overview of key research areas and offers insights into existing knowledge.
Pedagogical innovations
One pivotal research domain explores the pedagogical implications of AI in higher education, recognizing its potential to revolutionize the educational process and enhance efficiency (Al-Zahrani, 2023 ; Al-Zahrani, 2024a ; Kuleto et al. 2021 ; Zheng et al. 2023 ). AI integration in transnational higher education, including distance and online education, holds the promise of improving efficiencies and transforming management, administration, student recruitment, and pedagogical processes, leading to enhanced sustainability and development (El-Ansari, 2021 ). Huang ( 2018 ) emphasizes AI’s role in innovating education, noting its ability to transform learning interactions from machine-focused to knowledge-centered approaches based on learner needs.
Numerous studies delve into how AI-powered technologies, such as intelligent tutoring systems and adaptive learning platforms, enhance personalized learning experiences, promote student engagement, and improve academic outcomes (Al-Zahrani, 2023 ; Al-Zahrani, 2024a ; Chu et al. 2022 ; Dai and Ke, 2022 ). Kuleto et al.‘s ( 2021 ) findings demonstrate the significance of AI in improving learning outcomes, particularly in enhancing students’ skills, promoting collaborative learning, and providing a more accessible research environment. Additionally, Seo et al. ( 2021 ) highlight the potential of incorporating AI systems in online learning to facilitate personalized learner-instructor interactions on a large scale. Kochmar et al. ( 2022 ) present experimental results showing that AI tutoring systems lead to significant overall improvements in student performance outcomes.
Furthermore, AI has the potential to transform higher education by enhancing teaching and learning, improving assessment and feedback, increasing access and retention, reducing costs and time, and supporting administration and management (Abdous, 2023 ; Al-Zahrani, 2024a ; Bates et al. 2020 ; Chu et al. 2022 ; Popenici and Kerr, 2017 ; UNESCO, 2021 ). Almaiah et al.‘s ( 2022 ) study suggests a positive inclination towards integrating AI into educational environments, attributing it to AI being recognized as an innovative teaching tool. Huang ( 2018 ) observes positive effects of AI teaching systems on environmental education for college students.
Moreover, AI can revolutionize social interactions within higher educational settings, impacting learners, teachers, and technological systems (Al-Zahrani, 2023 ; Al-Zahrani, 2024a ; Dai and Ke, 2022 ). Crown et al. ( 2011 ) demonstrate the positive impact of an interactive chatbot on engineering students’ engagement and motivation. Essel et al. ( 2022 ) find that students engaging with a virtual teaching assistant (chatbot) show improved academic performance. Kumar ( 2021 ) observes the positive impacts of chatbots on learning performance and teamwork.
Learning analytics and student support
AI’s potential for innovation in education is prominent in the realm of learning analytics and student support. There has been a shift towards utilizing student data and analytics to enhance the educational experience and improve learning outcomes (André Renz, 2021 ; Huang et al. 2021 ; Zheng et al. 2023 ). AI technologies enable real-time analysis of vast amounts of data not limited to students’ learning but about their emotions as well, offering advantages in identifying at-risk students, recommending personalized interventions, and facilitating timely feedback and assessment (Zhi Liu et al. 2024 ; X. Liu et al. 2023 ). Learning analytics and AI-driven student support systems can provide actionable insights to educators and enhance student success (André Renz, 2021 ; MET, 2022 ; Ouyang et al. 2023 ; Gallagher, 2020 ).
For example, Dong and Hu’s ( 2019 ) study successfully identified contextual factors differentiating high-achieving and low-achieving students in reading literacy using machine learning techniques. Li et al.‘s ( 2022 ) optimized AI-based genetic algorithm grouping method for collaborative groups in higher education outperformed traditional grouping methods. Ouyang et al. ( 2023 ) utilized AI algorithms and learning analytics to analyze groups’ collaboration patterns in online interaction settings.
Assessment and grading
AI’s role in automating assessment and grading processes is another significant area of interest. Scholars investigate the reliability and validity of AI-based grading systems, comparing them to traditional human grading methods, and explore the potential benefits and limitations of automated grading (Lockwood, 2014 ; CTL, 2023 ; CUPA, 2023 ; McNulty, 2023 ; Chen, 2023 ). AI in assessment, including Natural Language Processing (NLP) and plagiarism detection, can automate grading, reduce workload, and enable data-driven decision-making (Lockwood, 2014 ; CTL, 2023 ; CUPA, 2023 ; McNulty, 2023 ; Chen, 2023 ).
While the potential benefits of AI in assessment and grading are significant, it’s also important to consider its practical applications and impact on student outcomes. For instance, Susilawati et al.‘s ( 2022 ) study explored the positive influence of digital assessment and trust on student character and academic performance. Additionally, Hooda et al.‘s ( 2022 ) examination of AI-driven assessment and feedback practices revealed positive impacts on students’ learning outcomes. Learning analytics, in this context, enables higher education institutions to support the learning environment at multiple levels.
Educators’ professional development
AI contributes to pedagogical innovation in the domain of educators’ professional development. Research explores the implications of AI for educators’ professional development, focusing on how AI technologies can support instructors in developing positive perceptions and attitudes, adaptive teaching strategies, and personalized learning experiences (Al-Zahrani, 2023 ; Al-Zahrani, 2024a ; CTL, 2023 ; Chen, 2023 ; Seo et al. 2021 ).
As AI integration in education progresses, it becomes increasingly important to address the concerns and training needs of educators. Educational institutions, policymakers, and AI developers must collaboratively address concerns regarding AI integration and provide the necessary support and training for educators to effectively implement AI technologies in their teaching practices (Al-Zahrani, 2024a ).
One example of how AI can be applied to enhance educators’ professional development is through the use of machine learning to analyze student feedback. Esparza et al.‘s ( 2018 ) ‘SocialMining’ model utilizes machine learning algorithms to enhance teaching techniques based on student comments on teacher performance. Integrating AI into educators’ professional development holds promise for improving instructional practices and the overall quality of education, providing targeted support and personalized learning resources.
Ethical and social implications
The ethical and social dimensions of AI in higher education are critical considerations. AI’s advancement introduces ethical challenges and concerns, necessitating further research to explore the social implications of AI, including accountability in AI-mediated practices and its influence on teaching and learning relationships (Al-Zahrani, 2024b ; Bearman et al. 2022 ). Challenges related to privacy, ethics, and morality in AI-driven approaches require interdisciplinary collaborations for comprehensive research and development (Al-Zahrani, 2024b ; Hu et al. 2023 ; Zhang and Aslan, 2021 ).
Scholars delve into issues of algorithmic bias, discrimination, fairness, transparency, and accountability in AI-driven educational systems (Al-Zahrani, 2024b ; UNESCO, 2021 ; Abdous, 2023 ; Schiff, 2022 ). Ethical considerations in deploying AI technologies, ensuring equity and inclusivity, and balancing human instructors’ roles with AI tools are explored. The societal impact of AI, including changes in employment patterns and the transformation of the workforce, requires careful consideration (Bates et al. 2020 ; Popenici and Kerr, 2017 ; Lo Piano, 2020 ; Seo et al. 2021 ; Chen, 2023 ).
Ethical considerations in integrating AI into everyday environments should be thoroughly addressed (Al-Zahrani, 2024b ; Doncieux et al. 2022 ). This includes examining AI’s impact on human life and societies. Dignum ( 2017 ) emphasizes the importance of upholding societal values, considering moral and ethical implications, and ensuring transparency in AI reasoning processes. In Seo et al.‘s ( 2021 ) study, concerns arise regarding responsibility, agency, surveillance, and potential privacy violations by AI systems. Raising awareness about human-centered values and responsible, ethical AI development is crucial in addressing these concerns (Al-Zahrani, 2024b ; André Renz, 2021 ).
Table 1 summarizes the existing focus areas within each research domain related to AI in higher education and highlights the identified gaps that warrant further investigation. These gaps include aspects such as higher-order thinking skills, collaboration and communication, development of AI skills, large-scale implementation of learning analytics, ethical considerations in assessment and grading, addressing educators’ concerns and training needs, and a comprehensive exploration of ethical concerns and societal impacts.
Significance and novelty of the research
The novelty of this study lies in its holistic and comprehensive investigation of the multifaceted impact of AI on higher education in Saudi Arabia. It makes a unique contribution by capturing the perspectives of diverse stakeholders, including students, faculty, and administrators, through a robust quantitative approach with a large sample size ( N = 1113).
While previous research has explored specific aspects of AI in education, this study offers a comprehensive examination of stakeholders’ attitudes, perceptions, and expectations regarding AI implementation. Notably, it delves into the influence of AI on teaching and learning processes. Additionally, it explores the ethical and social implications arising from AI integration—an area that warrants further exploration.
Furthermore, the study sheds light on stakeholders’ visions for the future role of AI in higher education, providing valuable insights into their aspirations and concerns. By addressing these objectives within the Saudi Arabian context, the study contributes to the growing body of knowledge on AI in higher education and i.
Methodology
In this study, a quantitative approach was employed using a survey questionnaire to comprehensively explore the multifaceted impact of AI on higher education.
Research design
To gather insights into the attitudes, perceptions, and experiences of students and faculty members regarding AI in higher education, an online survey questionnaire was meticulously developed. The questionnaire comprised two main sections. The first section delved into participant demographics, encompassing age, gender, current occupation, education level, subjective AI expertise, utilized AI tools and services, frequency of usage, and purpose of usage.
The second section of the questionnaire consisted of 32 items designed to explore participants’ perspectives on AI in higher education, encompassing attitudes, perceptions, the role of AI in teaching and learning, ethical and social implications, and the envisioned future role of AI. These items were carefully developed based on an extensive review of the literature and aligned with the study’s research questions and objectives. The item development process involved identifying relevant constructs and themes, input from subject matter experts, and rigorous refinement to ensure clarity and relevance (see Appendix 1 ).
Validity and reliability
To ensure the questionnaire’s validity, three experts in the educational technology field meticulously reviewed it, providing suggestions and endorsing modifications.
The Cronbach’s alpha coefficient, a measure of internal consistency and reliability, was calculated to assess the questionnaire items. For the overall questionnaire, comprising all 32 items in the second section, the responses from the entire sample ( N = 1113) were coded and analyzed using SPSS (v. 22). This software computed the average inter-item correlation among the 32 items and used this to calculate the Cronbach’s alpha coefficient, yielding a robust value of α = 0.96. Furthermore, the Cronbach’s alpha was calculated separately for the sub-scales (attitudes and perceptions, role of AI in teaching and learning, ethical and social implications, and the future role of AI), following the same process of analyzing inter-item correlations within each subset of items. Table 2 shows detailed reliability statistics of the survey and its sub-scales.
The sampling strategy employed in this study aims to secure a representative sample that mirrors the diversity present in higher education institutions across Saudi Arabia. To achieve this objective, both public and private universities are included, thereby ensuring a comprehensive representation of various institutional contexts. The snowball technique, a method where existing participants recruit future participants from among their acquaintances, is employed to systematically identify participants for the current study.
By incorporating individuals from diverse disciplines, programs, and academic levels, our research endeavors to encompass a wide array of perspectives and experiences concerning the influence of AI in higher education. This inclusivity enhances the generalizability of our findings and contributes to a more thorough comprehension of the topic at hand. In total, a total of 1,113 participants were involved in the study.
Demographics
Table 3 presents an overview of the demographic characteristics of our study participants.
A significant majority (77.9%) falls within the age bracket of 24 years or younger, with comparatively smaller proportions in older age groups (Fig. 1 ).
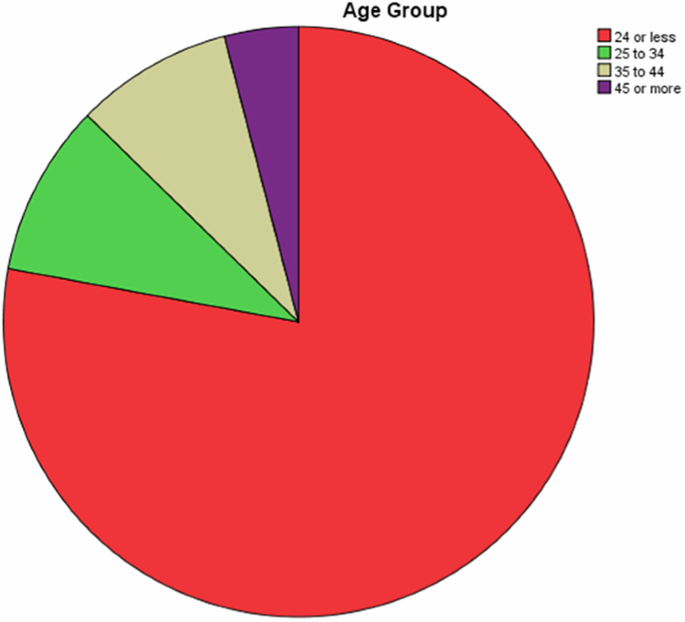
Sample Distribution based on Age.
Gender distribution reveals that females constitute a slightly higher percentage (55.3%) than males (44.7%). See Fig. 2 .

Sample Distribution based on Gender.
Examining the current occupations of participants, the predominant group (83.6%) identifies as students, followed by faculty members (10.1%) and administrators (6.3%) as shown in Fig. 3 .
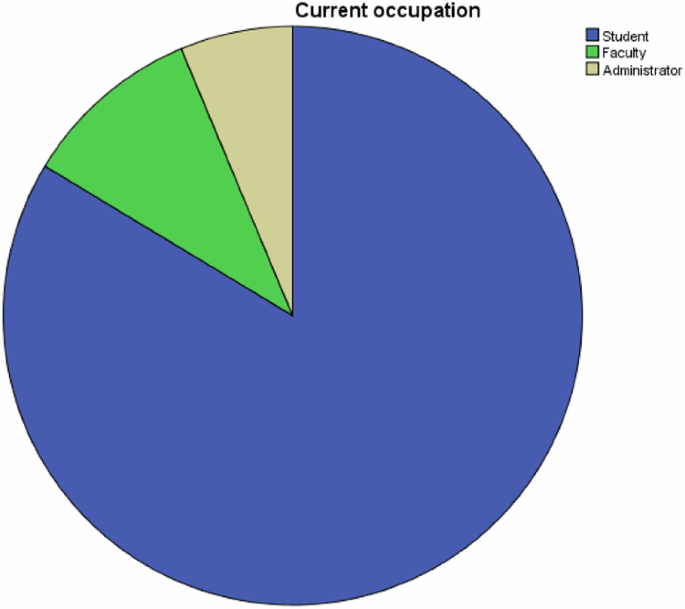
Sample Distribution based on Occupation.
Educational attainment varies (Fig. 4 ), with the majority (85.0%) holding a Bachelor’s degree, while a smaller percentage possess a Master’s degree (6.2%) or a PhD (8.8%).
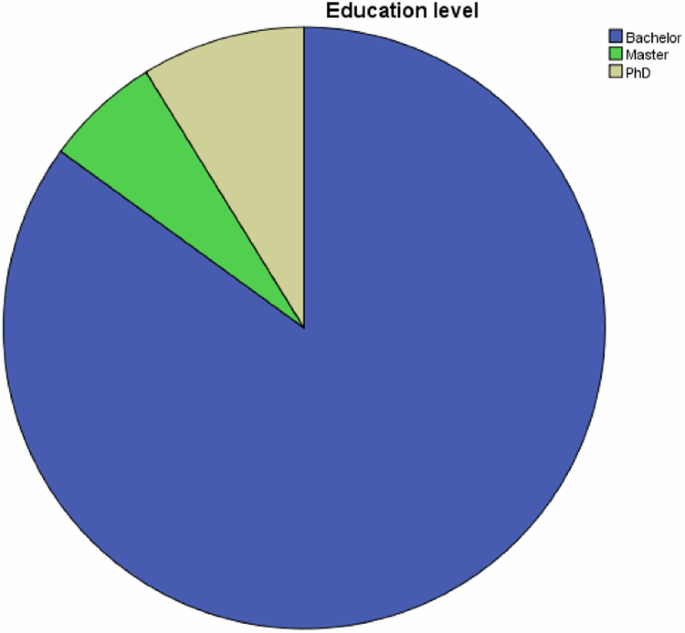
Sample Distribution based on Education Level.
Participants’ academic pursuits are diverse, with the most prevalent fields of study being Medicine, Engineering, or Computer Science (63.8%), followed by Literary, Humanities, or Education (21.7%), and Business, Commerce, or Law (14.5%). See Fig. 5 .
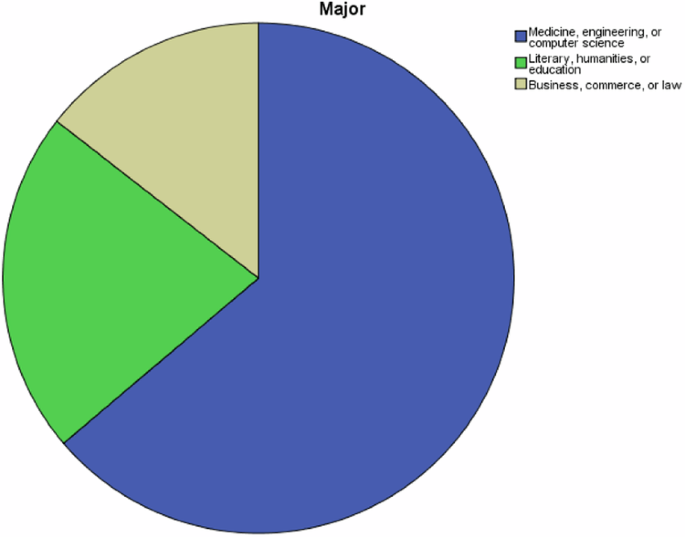
Sample Distribution based on Major.
In terms of self-perceived AI expertise, a significant portion (46.5%) rates their proficiency as low, while a slightly smaller percentage (43.9%) considers it to be medium, and a smaller fraction (9.6%) deems their AI expertise as high. See Fig. 6 .
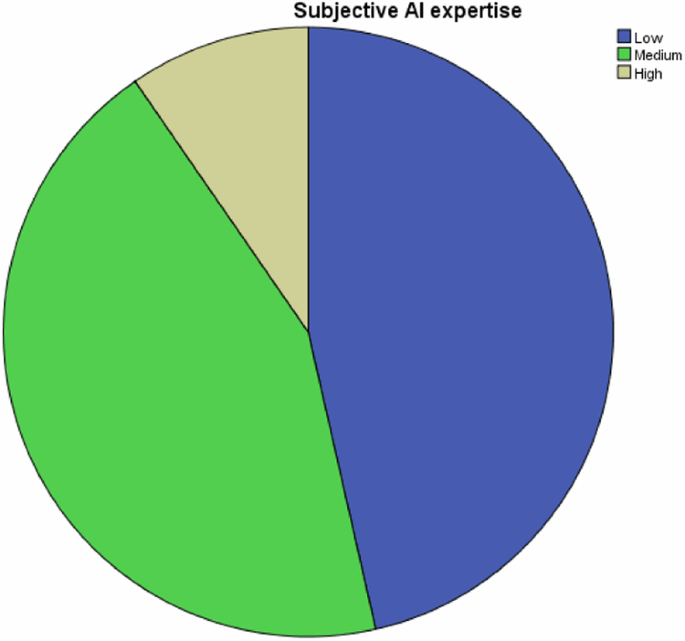
Sample Distribution based on Subjective AI Expertise.
Lastly, examining usage frequency, a noteworthy segment of participants (32.8%) engages with AI on a daily basis, while others utilize it on a weekly (21.2%), monthly (16.4%), or infrequent basis (29.6%). See Fig. 7 .
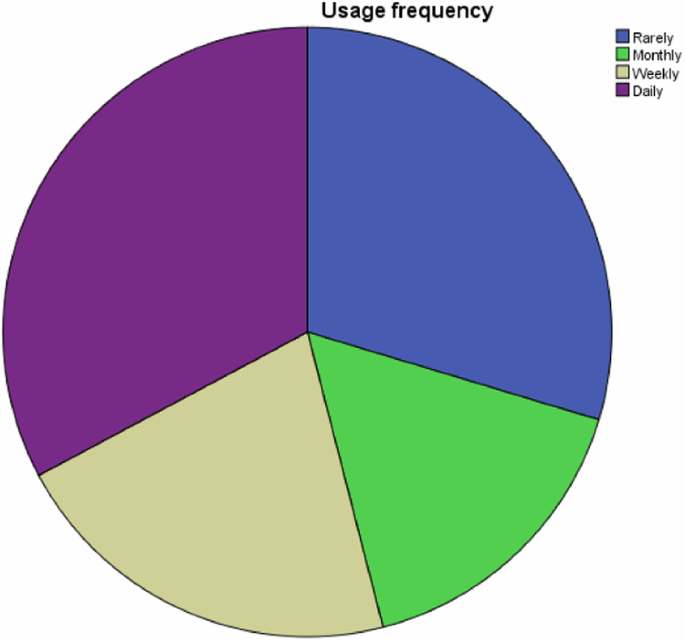
Sample Distribution based on Usage Frequency.
Table 4 presents a comprehensive overview of participant evaluations concerning AI tools, their purposes, and encountered negative experiences. When it comes to AI tools and services, face recognition services garnered the highest mean score of 4.32, signifying a positive evaluation among participants. Speech recognition services also received favorable ratings, boasting a mean score of 3.92. AI-Chatting tools obtained a commendable mean score of 3.85, reflecting a positive perception. On the other hand, AI-powered design, and creativity tools, along with Google AI services, received slightly lower mean scores of 3.60 each.
Examining the purposes of usage, general purposes received the highest mean score of 4.38, indicating strong positive evaluations. Educational purposes were also highly rated, achieving a mean score of 4.35. Research purposes garnered a positive evaluation with a mean score of 4.18, while entertainment purposes scored slightly lower at 3.96. e-Government and commercial purposes obtained lower mean scores of 3.49 and 3.34, respectively.
Delving into negative experiences, privacy and security concerns received a mean score of 3.57, indicating a moderate level of concern among participants. Technical issues during usage and installation both scored mean scores of 3.35, reflecting moderate challenges. Financial costs were rated with a mean score of 3.27, indicating a moderate level of cost-related concerns. Additionally, participants reported usage difficulties, which received a mean score of 3.02, suggesting a moderate level of difficulty encountered.

Attitudes and perceptions towards the use of AI in higher education (RQ1)
Table 5 reveals positive attitudes and perceptions towards the use of AI in higher education. Participants recognized AI’s potential to enhance the learning experience, improve access to resources, optimize administrative processes, and revolutionize higher education institutions. The mean scores ranged from 4.43 to 4.19, indicating strong agreement with these statements. These findings demonstrate participants’ openness and optimism towards integrating AI technologies in higher education.
Role of AI in Teaching and Learning in Higher Education (RQ2)
Table 6 highlights participants’ positive perceptions regarding the Role of AI in teaching and learning in higher education. They acknowledged AI’s potential to improve accessibility, facilitate personalized learning experiences, automate administrative tasks, create adaptive learning environments, provide real-time insights into student performance, enhance engagement and participation, influence teaching methods, and support the development of critical thinking and problem-solving skills. The mean scores ranged from 4.31 to 4.10, indicating strong agreement with these statements. Overall, participants recognized the positive effects of AI in higher education settings.
Ethical and social implications of AI in higher education (RQ3)
Table 7 reveals participants’ strong agreement regarding the ethical and social implications of using AI in higher education. They emphasized the need for ethical guidelines, respect for student autonomy, avoidance of societal inequalities, preservation of human interaction, addressing biases, prioritizing data ethics, ensuring transparency and accountability, and addressing privacy and security concerns. The mean scores ranged from 4.47 to 4.24, indicating a high level of agreement. Overall, participants recognized the importance of considering ethical and social aspects when integrating AI in higher education.
The future role of AI in higher education (RQ4)
Table 8 indicates participants’ positive expectations regarding the future role of AI in higher education. They recognized AI’s potential to contribute to the development of intelligent tutoring systems, prioritize ethical considerations and human values, transform teaching and learning, foster collaboration and interdisciplinary research, develop personalized learning pathways, enhance assessment processes, address individual learning needs, and support the development of lifelong learning skills. The mean scores ranged from 4.36 to 4.24, indicating a high level of agreement. Overall, participants expressed optimism about the future integration of AI in higher education.
The Impact of Demographic Characteristics on the Participants’ Perspectives (RQ5)
Multivariate Analysis of Variance tests (MANOVA) were conducted on various factors indicate their significant effects on the variables under consideration. Table 9 provides information on the effect, F-value, Wilks’ Lambda, significance level (p-value), and partial eta squared (ηp²).
The Multivariate test yielded significant results for the variables total uses, total purposes, and total difficulties. For total uses, the test showed a significant effect (Wilks’ Lambda = 0.492, F = 2.845, p < 0.001, ηp² = 0.163), indicating that the independent variable(s) have a statistically significant impact on the uses of AI. Similarly, for total purposes, the test revealed a significant effect (Wilks’ Lambda = 0.547, F = 2.272, p < 0.001, ηp² = 0.140), suggesting that the independent variable(s) significantly influence the purposes of AI. In the case of total difficulties, the test demonstrated a significant effect (Wilks’ Lambda = 0.614, F = 1.898, p < 0.001, ηp² = 0.115), indicating that the independent variable(s) have a statistically significant impact on the difficulties associated with AI. These findings highlight the importance of the independent variable(s) in shaping attitudes, goals, and challenges related to AI use.
Table 10 presents the results of the tests of between-subjects effects for the variables total uses, total purposes, and total difficulties.
These tests examine the significance of the independent variables on the dependent variables as follows:
Uses have a significant impact (F = 4.570, p = 0.000, ηp² = 0.237) on attitudes and perceptions.
Uses have a significant impact (F = 4.803, p = 0.000, ηp² = 0.246) on role of AI in teaching and learning.
Uses show a significant impact (F = 2.035, p = 0.006, ηp² = 0.121) on ethical and social implications.
Uses have a significant impact (F = 3.713, p = 0.000, ηp² = 0.201) on future role of AI.
For purposes:
Purposes have a significant impact (F = 4.870, p = 0.000, ηp² = 0.257) on attitudes and perceptions.
Purposes have a significant impact (F = 5.177, p = 0.000, ηp² = 0.269) on role of AI in teaching and learning.
Purposes show a significant impact (F = 2.331, p = 0.001, ηp² = 0.142) on ethical and social implications.
Purposes have a significant impact (F = 4.086, p = 0.000, ηp² = 0.225) on future role of AI.
For difficulties:
Difficulties have a significant impact (F = 2.298, p = 0.002, ηp² = 0.135) on attitudes and perceptions.
Difficulties have a significant impact (F = 1.980, p = 0.008, ηp² = 0.118) on role of AI in teaching and learning.
Difficulties have a significant impact (F = 1.676, p = 0.036, ηp² = 0.102) on future role of AI.
Figure 8 provides a visual representation of these relationships, illustrating how the uses, purposes, and difficulties associated with AI correlate with attitudes, perceptions, and views on the role and future of AI in higher education.
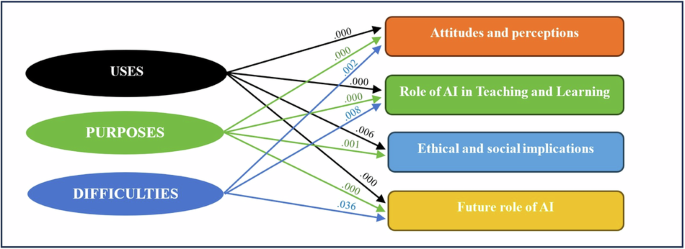
Summary of Relationships and p values.
This study delves into the repercussions of AI on higher education, scrutinizing its ethical, social, and educational ramifications. It investigates the viewpoints and attitudes of students, faculty, and administrators towards the implementation of AI, concentrating on AI’s role in teaching and learning, ethical concerns, and stakeholders’ expectations for its future integration in higher education. The findings contribute valuable insights for stakeholders in higher education, fostering a deeper comprehension of the impact of AI and emphasizing the ethical and educational dimensions of its application.
While a significant portion of participants reported low subjective expertise in AI, a noteworthy percentage indicated daily engagement with AI technologies. This suggests that despite perceiving limited expertise, individuals actively involve themselves with AI in their daily lives (Doncieux et al. 2022 ; Hassan et al. 2022 ; Jiang and Pang, 2023 ; Kuo et al. 2021 ; Vendraminelli et al. 2022 ). The increasing popularity of AI technologies among higher education stakeholders is a promising trend.
Results further unveiled positive evaluations of AI tools and services by participants. Face recognition services received the highest rating, followed by speech recognition services and AI-chatting tools like chatbots. This indicates a readiness within higher education to embrace these technologies, building on successful prior research findings, particularly regarding chatbot implementation (Crown et al. 2011 ; Dai and Ke, 2022 ; Essel et al. 2022 ; Kumar, 2021 ). Conversely, AI-powered design and creativity tools, along with Google AI services, received slightly lower ratings, likely due to the specialized knowledge and expertise required for operation. Participants acknowledge the value of AI for tasks like facial recognition, speech processing, and chatbots, yet there appears to be room for improvement in training and support for advanced AI-powered technologies.
Moreover, participants highly prioritize and value AI for general, educational, research, and entertainment purposes. However, there is slightly lower enthusiasm for AI in e-Government and commercial applications. This might stem from concerns about the perceived lack of impact, or ethical implications and potential risks associated with AI in these contexts, as established in prior research (Al-Zahrani, 2023 ; Al-Zahrani, 2024a ; Farisco et al. 2020 ; Kerr et al. 2020 ; Owe and Baum, 2021 ; Resseguier and Rodrigues, 2020 ; Ryan and Stahl, 2020 ; Stahl et al. 2021 ).
The study also delves into negative experiences associated with AI usage, revealing participants’ moderate concerns about privacy and security issues. This indicates an awareness of potential risks and implications, especially related to data privacy and security breaches. Robust privacy measures in AI applications are emphasized, aligning with previous research findings (André Renz, 2021 ; Crawford et al. 2023 ; Doncieux et al. 2022 ; Dignum, 2017 ; Seo et al. 2021 ; Zhang and Aslan, 2021 ). Additionally, challenges such as technical issues during usage, installation difficulties, usage problems, and financial costs were moderately reported. These results underscore the need for active administration, improved user interfaces, cost reduction, comprehensive training, and support to enhance the overall user experience of AI technologies.
Participants in the study exhibit positive attitudes and perceptions towards the use of AI in higher education. They recognize AI’s potential to enhance the learning experience, personalize instruction, improve student outcomes, and optimize administrative processes. Improved access to educational resources is also identified as a benefit of AI, enabling students to utilize digital libraries, online databases, and academic materials. This aligns with the broader discourse on technology’s transformative impact in education (Al-Zahrani, 2023 ; Al-Zahrani, 2024a ; Chu et al. 2022 ; Dai and Ke, 2022 ; El-Ansari, 2021 ; Kuleto et al. 2021 ; Kochmar et al. 2022 ; Huang, 2018 ; Seo et al. 2021 ; Z. Liu et al. 2023 ).
Furthermore, participants express positive perceptions regarding the role of AI in teaching and learning in higher education. They recognize its potential to improve accessibility, personalize learning experiences, automate administrative tasks, create adaptive learning environments, provide real-time insights into student performance, enhance engagement and participation, influence teaching methods, and support the development of critical thinking and problem-solving skills. These findings highlight the benefits and opportunities that AI brings to the educational process, including equal access to education, tailored instruction, efficient administrative operations, dynamic learning environments, data-driven decision-making, increased student engagement, and the cultivation of higher-order cognitive abilities.
Moreover, participants emphasize the importance of ethical and social implications of AI in higher education, underscoring the need for ethical guidelines to govern AI implementation. This includes respect for student autonomy, avoidance of societal inequalities, preservation of human interaction, addressing biases in AI systems, data ethics, transparency, accountability, and addressing privacy and security concerns. These results align with previous research (Al-Zahrani, 2024b ; André Renz, 2021 ; Crawford et al. 2023 ; Doncieux et al. 2022 ; Dignum, 2017 ; Seo et al. 2021 ; Zhang and Aslan, 2021 ).
Participants’ optimism regarding the future role of AI in higher education underscores its significant impact on various aspects of teaching, learning, and educational processes. This includes the development of intelligent tutoring systems for personalized and adaptive learning experiences, prioritizing ethical considerations and human values, transforming teaching and learning processes, fostering collaboration and interdisciplinary research, developing personalized learning pathways, enhancing assessment processes, addressing specific learning needs, and supporting the development of lifelong learning skills. These findings affirm the positive perceptions, attitudes, and the required ethical considerations identified in the current study, indicating the readiness of higher education to adopt recent advancements in AI technologies and their implications for educational contexts.
MANOVA tests emphasize the significance of total AI uses and total purposes in understanding attitudes, perceptions, and future implications of AI integration in teaching and learning. The results indicate that the extent and purposes of AI usage have notable impacts on various aspects of AI integration, influencing attitudes, perceptions, and perceived future implications. These findings underscore the importance of practical AI implementation in educational settings and its potential to shape individuals’ perceptions of AI’s role.
Furthermore, the significant effects of total purposes highlight the critical role of intentionality in AI implementation. The purposes for which AI is used in teaching and learning substantially impact attitudes, perceptions, and future implications. This underscores the need for clear goals and careful consideration of the ethical and social implications associated with AI integration.
Lastly, it is noteworthy that total difficulties had a significant influence on attitudes, perceptions, and the future role of AI, although to a lesser extent compared to total uses and total purposes. The difficulties encountered in AI usage are associated with individuals’ attitudes and perceptions towards AI, as well as their perspectives on its future role. These findings imply that addressing challenges and providing adequate support in AI implementation can contribute to more positive attitudes and perceptions, leading to effective integration of AI in higher education.
To sum up, these results illuminate the intricate interplay between AI usage, purposes, difficulties, and their impact on attitudes, perceptions, and future implications. They underscore the necessity for a comprehensive understanding of AI integration, considering not only its technical aspects but also the ethical, social, and educational dimensions. By acknowledging the role of AI uses, AI usage purposes, and addressing associated difficulties, educational stakeholders can work towards harnessing the benefits of AI while ensuring responsible and effective implementation in teaching and learning contexts.
Conclusions and implications
This study sheds light on the favorable attitudes and perceptions of stakeholders in higher education towards the adoption of AI. Participants not only acknowledged the value of AI in enriching teaching and learning experiences but also in improving resource accessibility, streamlining administrative processes, and fostering innovation within higher education institutions. Their enthusiasm extended particularly to AI tools and services, such as facial recognition, speech processing, and chatbots. However, they also recognized the imperative for advancements in more sophisticated AI technologies.
Ethical considerations took precedence among participants, underscoring the need for guidelines governing AI implementation. Privacy, security, and bias were identified as critical issues requiring attention. Despite these concerns, there was a prevailing optimism regarding AI’s future role in higher education, with participants envisioning personalized learning experiences, ethical AI integration, collaborative endeavors, and ongoing support for lifelong learning.
The study underscores how the utilization, purposes, and challenges of AI influence attitudes, perceptions, and future implications. Exposure and experience with AI emerged as key determinants shaping individuals’ perspectives. Aligning AI goals with educational objectives and effectively addressing associated challenges were identified as crucial factors in fostering positive attitudes and perceptions. These findings underscore the necessity for comprehensive implementation strategies, encompassing technical, ethical, social, and educational considerations.
In summary, this research offers insights for stakeholders in higher education, aiding their decision-making processes concerning the ethical and educational ramifications of AI. The study’s findings yield several implications for policy and practice in higher education:
Develop and implement ethical guidelines for AI integration.
Invest in professional development and training programs for faculty, administrators, and students.
Provide resources and infrastructure to support AI implementation.
Encourage collaboration and interdisciplinary research on AI in education.
Address data ethics and privacy concerns in AI implementation.
Establish evaluation frameworks to measure the impact of AI.
Foster collaborations with industry partners for AI development.
Continuously monitor and adapt AI implementations in response to challenges.
Limitations and future research
While this study offers valuable insights into AI’s impact on higher education, it is crucial to acknowledge its limitations. First, the study depends on participants’ self-reported data, which can be biased and prone to inaccuracies. Their responses may not always align with their actual behaviors or attitudes towards AI. Second, the study may not fully incorporate contextual factors that could influence participants’ attitudes and perceptions regarding AI in higher education. Factors like cultural, institutional, or regional differences could impact the findings. Finally, the study concentrates solely on AI’s impact on higher education and may not encompass the broader societal implications or perspectives from other sectors.
Future research can address the study’s limitations by conducting cross-cultural and comparative studies, conducting interdisciplinary research on AI in education, and performing comparative analysis with other educational contexts. This will enhance understanding of the influence of contextual factors, uncover broader societal implications, and identify unique considerations specific to higher education.
Data availability
Available as supplementary material.
Abdous M (2023) How AI Is Shaping the Future of Higher Ed. Inside Higher Ed | Higher Education News, Events and Jobs. https://www.insidehighered.com/views/2023/03/22/how-ai-shaping-future-higher-ed-opinion
Almaiah MA, Alfaisal R, Salloum SA, Hajjej F, Thabit S, El-Qirem FA, Al-Maroof RS (2022) Examining the Impact of Artificial Intelligence and Social and Computer Anxiety in E-Learning Settings: Students’ Perceptions at the University Level. Electronics 11(22):3662, https://www.mdpi.com/2079-9292/11/22/3662
Article Google Scholar
Al-Zahrani AM (2023) The impact of generative AI tools on researchers and research: Implications for academia in higher education. Innovations in Education and Teaching International, 1-15. https://doi.org/10.1080/14703297.2023.2271445
Al-Zahrani AM (2024a) From Traditionalism to Algorithms: Embracing Artificial Intelligence for Effective University Teaching and Learning. Educational Technology at IgMin 2(2):102–0112. https://doi.org/10.61927/igmin151
Article MathSciNet Google Scholar
Al-Zahrani AM (2024b) Unveiling the shadows: Beyond the hype of AI in education. Heliyon 10(9):e30696. https://doi.org/10.1016/j.heliyon.2024.e30696
Article PubMed PubMed Central Google Scholar
André Renz GV (2021) Reinvigorating the Discourse on Human-Centered Artificial Intelligence in Educational Technologies. Technology Innovation Management Review 11(5):5–16. https://doi.org/10.22215/timreview/1438
Bates T, Cobo C, Mariño O, Wheeler S (2020) Can artificial intelligence transform higher education? International Journal of Educational Technology in Higher Education 17(1):42. https://doi.org/10.1186/s41239-020-00218-x
Bearman M, Ryan J, Ajjawi R (2022) Discourses of artificial intelligence in higher education: a critical literature review. Higher Education. https://doi.org/10.1007/s10734-022-00937-2
Bigman YE, Wilson D, Arnestad MN, Waytz A, Gray K (2022) Algorithmic discrimination causes less moral outrage than human discrimination. Journal of Experimental Psychology 152(1):4–27. https://doi.org/10.1037/xge0001250
Article PubMed Google Scholar
Bozkurt A, Karadeniz A, Baneres D, Guerrero-Roldán AE, Rodríguez ME (2021) Artificial Intelligence and Reflections from Educational Landscape: A Review of AI Studies in Half a Century. Sustainability 13(2):800, https://www.mdpi.com/2071-1050/13/2/800
Cambridge University Press & Assessment (CUPA) (2023) The Cambridge approach to generative AI and assessment. https://www.cambridge.org/news-and-insights/news/The-Cambridge-approach-to-generative-AI-and-assessment
Carmody J, Shringarpure S, Van De Venter G (2021) AI and privacy concerns: a smart meter case study. Journal of Information, Communication and Ethics in Society 19(4):492–505. https://doi.org/10.1108/jices-04-2021-0042
Center for Teaching and Learning (CTL) (2023) AI Tools in Teaching and Learning: Guidance on understanding how AI tools can impact teaching and learning. https://teachingcommons.stanford.edu/news/ai-tools-teaching-and-learning
Chen C (2023) AI Will Transform Teaching and Learning. Let’s Get it Right. https://hai.stanford.edu/news/ai-will-transform-teaching-and-learning-lets-get-it-right
Chu HC, Hwang GH, Tu YF, Yang KH (2022) Roles and research trends of artificial intelligence in higher education: A systematic review of the top 50 most-cited articles. Australasian Journal of Educational Technology 38(3):22–42
Google Scholar
Crawford J, Cowling M, Allen K (2023) Leadership is needed for ethical ChatGPT: Character, assessment, and learning using artificial intelligence (AI). Journal of University Teaching & Learning Practice, 20(3). https://doi.org/10.53761/1.20.3.02
Creely E (2022) Conceiving Creativity and Learning in a World of Artificial Intelligence: A Thinking Model. In D Henriksen & P Mishra (Eds.), Creative Provocations: Speculations on the Future of Creativity, Technology & Learning (pp. 35-50). Springer International Publishing. https://doi.org/10.1007/978-3-031-14549-0_3
Crown S, Fuentes A, Jones R, Nambiar R, Crown D (2011) Anne G. Neering: Interactive chatbot to engage and motivate engineering students. 21 , 24-34
Dai C-P, Ke F (2022) Educational applications of artificial intelligence in simulation-based learning: A systematic mapping review. Computers and Education: Artificial Intelligence 3:100087. https://doi.org/10.1016/j.caeai.2022.100087
Dignum V (2017) Responsible Artificial Intelligence: Designing Ai for Human Values. ITU Journal: ICT Discoveries, 1 . https://dspace.daffodilvarsity.edu.bd:8080/handle/123456789/2181
Doncieux S, Chatila R, Straube S, Kirchner F (2022) Human-centered AI and robotics. AI Perspectives 4(1):1. https://doi.org/10.1186/s42467-021-00014-x
Dong X, Hu J (2019) An Exploration of Impact Factors Influencing Students’ Reading Literacy in Singapore with Machine Learning Approaches. International Journal of English Linguistics, 9 (5). https://doi.org/10.5539/ijel.v9n5p52
El-Ansari M (2021) Exploring the Applicability of Artificial Intelligence in Transnational Higher Education. International Journal of Management Cases 23-33(2):20, https://www.circleinternational.co.uk/wp-content/uploads/2021/07/IJMC-23.2.pdf
Elliott D, Soifer E (2022) AI Technologies, Privacy, and Security. Frontiers in Artificial Intelligence, 5 . https://doi.org/10.3389/frai.2022.826737
Esparza GG, Canul-Reich J, Zezzatti A, Margain L, Ponce J (2018) Mining: Students Comments about Teacher Performance Assessment using Machine Learning Algorithms. 9 (3), 26-40. http://cathi.uacj.mx/20.500.11961/5676
Essel HB, Vlachopoulos D, Tachie-Menson A, Johnson EE, Baah PK (2022) The impact of a virtual teaching assistant (chatbot) on students’ learning in Ghanaian higher education. International Journal of Educational Technology in Higher Education 19(1):57. https://doi.org/10.1186/s41239-022-00362-6
Farisco M, Evers K, Salles A (2020) Towards Establishing Criteria for the Ethical Analysis of Artificial Intelligence. Science and Engineering Ethics 26(5):2413–2425. https://doi.org/10.1007/s11948-020-00238-w
Gallagher S (2020) The Pandemic Pushed Universities Online. The Change Was Long Overdue. Harvard Business Review. https://hbr.org/2020/09/the-pandemic-pushed-universities-online-the-change-was-long-overdue
Hassan R, Ali A, Howe CW, Zin AM (2022) Constructive alignment by implementing design thinking approach in artificial intelligence course: Learners’ experience. AIP Conference Proceedings, 2433(1). https://doi.org/10.1063/5.0072986
Hooda M, Rana C, Dahiya O, Rizwan A, Hossain MS (2022) Artificial Intelligence for Assessment and Feedback to Enhance Student Success in Higher Education. Mathematical Problems in Engineering 2022:5215722. https://doi.org/10.1155/2022/5215722
Article CAS Google Scholar
Hu F, Qiu L, Wei S, Zhou H, Bathuure IA, Hu H (2023) The spatiotemporal evolution of global innovation networks and the changing position of China: a social network analysis based on cooperative patents. R and D Management. https://doi.org/10.1111/radm.12662
Huang C, Han Z, Li M, Wang X, Zhao W (2021) Sentiment evolution with interaction levels in blended learning environments: Using learning analytics and epistemic network analysis. Australasian Journal of Educational Technology 37(2):81–95. https://doi.org/10.14742/ajet.6749
Huang S-P (2018) Effects of Using Artificial Intelligence Teaching System for Environmental Education on Environmental Knowledge and Attitude. Eurasia Journal of Mathematics, Science and Technology Education 14(7):3277–3284. https://doi.org/10.29333/ejmste/91248
Jiang C, Pang Y (2023) Enhancing design thinking in engineering students with project-based learning. Computer Applications in Engineering Education, n/a(n/a). https://doi.org/10.1002/cae.22608
Johnson GM (2021) Algorithmic bias: on the implicit biases of social technology. Synthese 198(10):9941–9961. https://doi.org/10.1007/s11229-020-02696-y
Kerr A, Barry M, Kelleher JC (2020) Expectations of artificial intelligence and the performativity of ethics: Implications for communication governance. Big Data & Society 7(1):205395172091593. https://doi.org/10.1177/2053951720915939
Kochmar E, Vu DD, Belfer R, Gupta V, Serban IV, Pineau J (2022) Automated Data-Driven Generation of Personalized Pedagogical Interventions in Intelligent Tutoring Systems. International Journal of Artificial Intelligence in Education 32(2):323–349. https://doi.org/10.1007/s40593-021-00267-x
Kordzadeh N, Ghasemaghaei M (2021) Algorithmic bias: review, synthesis, and future research directions. European Journal of Information Systems 31(3):388–409. https://doi.org/10.1080/0960085x.2021.1927212
Kuleto V, Ilić M, Dumangiu M, Ranković M, Martins OMD, Păun D, Mihoreanu L (2021) Exploring Opportunities and Challenges of Artificial Intelligence and Machine Learning in Higher Education Institutions. Sustainability 13(18):10424, https://www.mdpi.com/2071-1050/13/18/10424
Kumar JA (2021) Educational chatbots for project-based learning: investigating learning outcomes for a team-based design course. International Journal of Educational Technology in Higher Education 18(1):65. https://doi.org/10.1186/s41239-021-00302-w
Kuo J, Song X, Chen C, Patel CD (2021) Fostering Design Thinking in Transdisciplinary Engineering Education. In Advances in transdisciplinary engineering. IOS Press. https://doi.org/10.3233/atde210083
Laupichler MC, Aster A, Schirch J, Raupach T (2022) Artificial intelligence literacy in higher and adult education: A scoping literature review. Computers and Education: Artificial Intelligence 3:100101. https://doi.org/10.1016/j.caeai.2022.100101
Li X, Ouyang F, Chen W (2022) Examining the effect of a genetic algorithm-enabled grouping method on collaborative performances, processes, and perceptions. Journal of Computing in Higher Education 34(3):790–819. https://doi.org/10.1007/s12528-022-09321-6
Liu X, Wang S, Lu S, Yin Z, Li X, Yin L, Tian J, Zheng W (2023) Adapting feature selection algorithms for the classification of Chinese texts. Systems 11(9):483. https://doi.org/10.3390/systems11090483
Liu Z, Kong X, Liu S, Yang Z (2023) Effects of computer-based mind mapping on students’ reflection, cognitive presence, and learning outcomes in an online course. Distance Education 44(3):544–562. https://doi.org/10.1080/01587919.2023.2226615
Liu Z, Wen C, Su Z, Liu S, Sun J, Kong W, Yang Z (2024) Emotion-semantic-aware dual contrastive learning for epistemic emotion identification of learner-generated reviews in MOOCs. IEEE Transactions on Neural Networks and Learning Systems, 1–14. https://doi.org/10.1109/tnnls.2023.3294636
Lo Piano S (2020) Ethical principles in machine learning and artificial intelligence: cases from the field and possible ways forward. Humanities and Social Sciences Communications 7(1):9. https://doi.org/10.1057/s41599-020-0501-9
Lockwood J (2014) Handbook of Automated Essay Evaluation Current Applications and New Directions Mark D. Shermis and Jill Burstein (eds.) (2013) New York: Routledge. pp. 194 ISBN: 9780415810968. Writing & Pedagogy 6(2):437–441. https://doi.org/10.1558/wap.v6i2.437
McKinsey & Company (2022). How technology is shaping learning in higher education. McKinsey & Company. https://www.mckinsey.com/industries/education/our-insights/how-technology-is-shaping-learning-in-higher-education#
McNulty N (2023) Using AI for Auto-Marking of Assessment: Revolutionising the Grading Process. https://www.niallmcnulty.com/2023/05/using-ai-for-auto-marking-of-assessment-revolutionising-the-grading-process/
Microsoft Education Team (MET) (2022) How data and AI are changing the world of education. https://educationblog.microsoft.com/en-us/2022/04/how-data-and-ai-are-changing-the-world-of-education
Molenaar I (2022) The concept of hybrid human-AI regulation: Exemplifying how to support young learners’ self-regulated learning. Computers and Education: Artificial Intelligence 3:100070. https://doi.org/10.1016/j.caeai.2022.100070
Ouyang F, Xu W, Cukurova M (2023) An artificial intelligence-driven learning analytics method to examine the collaborative problem-solving process from the complex adaptive systems perspective. International Journal of Computer-Supported Collaborative Learning 18(1):39–66. https://doi.org/10.1007/s11412-023-09387-z
Owe A, Baum SD (2021) Moral consideration of nonhumans in the ethics of artificial intelligence. AI And Ethics 1(4):517–528. https://doi.org/10.1007/s43681-021-00065-0
Popenici SAD, Kerr S (2017) Exploring the impact of artificial intelligence on teaching and learning in higher education. Research and Practice in Technology Enhanced Learning 12(1):22. https://doi.org/10.1186/s41039-017-0062-8
Resseguier A, Rodrigues R (2020) AI ethics should not remain toothless! A call to bring back the teeth of ethics. Big Data & Society 7(2):205395172094254. https://doi.org/10.1177/2053951720942541
Ryan M, Stahl BC (2020) Artificial intelligence ethics guidelines for developers and users: clarifying their content and normative implications. Journal of Information, Communication and Ethics in Society 19(1):61–86. https://doi.org/10.1108/jices-12-2019-0138
Schiff D (2022) Education for AI, not AI for Education: The Role of Education and Ethics in National AI Policy Strategies. International Journal of Artificial Intelligence in Education 32(3):527–563. https://doi.org/10.1007/s40593-021-00270-2
Seo K, Tang J, Roll I, Fels S, Yoon D (2021) The impact of artificial intelligence on learner–instructor interaction in online learning. International Journal of Educational Technology in Higher Education 18(1):54. https://doi.org/10.1186/s41239-021-00292-9
Stahl BC, Antoniou J, Ryan M, Macnish K, Jiya T (2021) Organisational responses to the ethical issues of artificial intelligence. AI & SOCIETY 37(1):23–37. https://doi.org/10.1007/s00146-021-01148-6
Susilawati E, Lubis H, Kesuma S, Pratama I (2022) Antecedents of Student Character in Higher Education: The role of the Automated Short Essay Scoring (ASES) digital technology-based assessment model. Eurasian Journal of Educational Research 98:203–220. https://doi.org/10.14689/ejer.2022.98.013
UNESCO (2021) Artificial Intelligence and Education. Guidance for Policy-makers. The United Nations Educational, Scientific and Cultural Organization, 1-50. https://doi.org/10.54675/PCSP7350
Vendraminelli L, Macchion L, Nosella A, Vinelli A (2022). Design thinking: strategy for digital transformation. Journal of Business Strategy, ahead-of-print(ahead-of-print). https://doi.org/10.1108/JBS-01-2022-0009
Wang C, Wang K, Bian AA, Islam MR, Keya KN, Foulds JR, Pan S (2022). Do Humans Prefer Debiased AI Algorithms? A Case Study in Career Recommendation. https://doi.org/10.1145/3490099.3511108
Zawacki-Richter O, Marín VI, Bond M, Gouverneur F (2019) Systematic review of research on artificial intelligence applications in higher education – where are the educators? International Journal of Educational Technology in Higher Education 16(1):39. https://doi.org/10.1186/s41239-019-0171-0
Zhang K, Aslan AB (2021) AI technologies for education: Recent research & future directions. Computers and Education: Artificial Intelligence 2:100025. https://doi.org/10.1016/j.caeai.2021.100025
Zhao X, Yang M, Qu Q, Xu R, Li J (2022) Exploring privileged features for relation extraction with contrastive student-teacher learning. IEEE Transactions on Knowledge and Data Engineering, 1–1. https://doi.org/10.1109/tkde.2022.3161584
Zheng W, Lu S, Cai Z, Wang R, Wang L, Yin L (2023) PAL-BERT: An Improved Question Answering Model. Computer Modeling in Engineering & Sciences, 1-17. https://doi.org/10.32604/cmes.2023.046692
Download references
Author information
Authors and affiliations.
University of Jeddah, Jeddah, Saudi Arabia
Abdulrahman M. Al-Zahrani & Talal M. Alasmari
You can also search for this author in PubMed Google Scholar
Contributions
Abdulrahman M. Al-Zahrani and Talal M. Al-Asmari contributed equally to this work.
Corresponding author
Correspondence to Abdulrahman M. Al-Zahrani .
Ethics declarations
Competing interests.
The authors declare no competing interests.
Ethical approval
The questionnaire and methodology for this study was approved by the Learning Design and Technology Department at University of Jeddah (#23-241 on Feb. 07, 2023).
Informed consent
Prior to data collection, informed consent was obtained from all participants. Each individual was provided with information regarding the purpose of the study, their rights as participants (including the right to withdraw at any point), and the measures taken to protect their personal data. Participants gave their consent to participate voluntarily.
Additional information
Publisher’s note Springer Nature remains neutral with regard to jurisdictional claims in published maps and institutional affiliations.
Supplementary information
Appendix 1: survey, research data, rights and permissions.
Open Access This article is licensed under a Creative Commons Attribution 4.0 International License, which permits use, sharing, adaptation, distribution and reproduction in any medium or format, as long as you give appropriate credit to the original author(s) and the source, provide a link to the Creative Commons licence, and indicate if changes were made. The images or other third party material in this article are included in the article’s Creative Commons licence, unless indicated otherwise in a credit line to the material. If material is not included in the article’s Creative Commons licence and your intended use is not permitted by statutory regulation or exceeds the permitted use, you will need to obtain permission directly from the copyright holder. To view a copy of this licence, visit http://creativecommons.org/licenses/by/4.0/ .
Reprints and permissions
About this article
Cite this article.
Al-Zahrani, A.M., Alasmari, T.M. Exploring the impact of artificial intelligence on higher education: The dynamics of ethical, social, and educational implications. Humanit Soc Sci Commun 11 , 912 (2024). https://doi.org/10.1057/s41599-024-03432-4
Download citation
Received : 04 December 2023
Accepted : 03 July 2024
Published : 13 July 2024
DOI : https://doi.org/10.1057/s41599-024-03432-4
Share this article
Anyone you share the following link with will be able to read this content:
Sorry, a shareable link is not currently available for this article.
Provided by the Springer Nature SharedIt content-sharing initiative
Quick links
- Explore articles by subject
- Guide to authors
- Editorial policies


An official website of the United States government
The .gov means it’s official. Federal government websites often end in .gov or .mil. Before sharing sensitive information, make sure you’re on a federal government site.
The site is secure. The https:// ensures that you are connecting to the official website and that any information you provide is encrypted and transmitted securely.
- Publications
- Account settings
Preview improvements coming to the PMC website in October 2024. Learn More or Try it out now .
- Advanced Search
- Journal List
- Pak J Med Sci
- v.36(COVID19-S4); 2020 May
Advantages, Limitations and Recommendations for online learning during COVID-19 pandemic era
Khadijah mukhtar.
1 Khadijah Mukhtar, BDS, MME. Assistant Professor, DME. University College of Medicine and Dentistry, The University of Lahore, Lahore, Pakistan
Kainat Javed
2 Kainat Javed, MBBS, MME. Assistant Professor, DME. University College of Medicine and Dentistry, The University of Lahore, Lahore, Pakistan
Mahwish Arooj
3 Mahwish Arooj, MBBS, M. Phil, MME, PhD Physiology. Associate Professor, Physiology and Director DME, University College of Medicine and Dentistry, The University of Lahore, Lahore, Pakistan
Ahsan Sethi
4 Ahsan Sethi, BDS, MPH, MMEd, FHEA, MAcadMEd, FDTFEd, PhD Medical Education Assistant Professor, Institute of Health Professions Education and Research, Khyber Medical University, Peshawar, Pakistan
During COVID-19 pandemic, the institutions in Pakistan have started online learning. This study explores the perception of teachers and students regarding its advantages, limitations and recommendations.
This qualitative case study was conducted from March to April 2020. Using maximum variation sampling, 12 faculty members and 12 students from University College of Medicine and University College of Dentistry, Lahore were invited to participate. Four focus group interviews, two each with the faculty and students of medicine and dentistry were carried out. Data were transcribed verbatim and thematically analyzed using Atlas Ti.
The advantages included remote learning, comfort, accessibility, while the limitations involved inefficiency and difficulty in maintaining academic integrity. The recommendations were to train faculty on using online modalities and developing lesson plan with reduced cognitive load and increased interactivities.
Conclusion:
The current study supports the use of online learning in medical and dental institutes, considering its various advantages. Online learning modalities encourage student-centered learning and they are easily manageable during this lockdown situation.
INTRODUCTION
The spread of COVID-19 has led to the closure of educational institutions all over the world. This tested the preparedness of universities to deal with a crisis that requires the help of advanced technology including hardware and software to enable effective online learning. Such closure accelerated the development of the online learning environments so that learning would not be disrupted. 1 Many institutions have become interested in how to best deliver course content online, engage learners and conduct assessments. Hence, COVID-19 while being a hazard to humanity, has evolved institutions to invest in online learning.
Online learning systems are web-based software for distributing, tracking, and managing courses over the Internet. 2 It involves the implementation of advancements in technology to direct, design and deliver the learning content, and to facilitate two-way communication between students and faculty. 3 They contain features such as whiteboards, chat rooms, polls, quizzes, discussion forums and surveys that allow instructors and students to communicate online and share course content side by side. These can offer productive and convenient ways to achieve learning goals. In Pakistan, the institutions are using Microsoft Teams, Google meet, Edmodo and Moodle as learning management systems along with their applications for video conferencing. 4 Other commonly used video conferencing solutions include Zoom, Skype for business, WebEx and Adobe connect etc.
According to our literature review, three previous studies were found, 5 - 7 supporting online learning from Pakistan. The two studies at Dow University of Health Sciences, Karachi and Lahore Medical and Dental College, Lahore reported high student satisfaction with online learning modalities. The study from Khyber Pakhtunkhwa assessed the feasibility of online learning among students, trainees and faculty members. They reported good technology access, online skills, and preparedness for online discussions among participants across the medical education continuum.
With the increase in use of online modalities during COVID-19, it is necessary to assess their effectiveness with regards to teaching and learning from various stakeholders. 8 Therefore, the current study explores the perception of faculty members and students regarding the advantages, limitations and recommendations for online learning in Pakistan. The study is timely as Higher Education Commission (HEC) is in the process of implementing online learning across all the universities in Pakistan. The findings will help identify the required changes on priority basis to make it more practical and worthwhile.
This qualitative case study was conducted from March to April 2020 in two medical and dental institutes. Ethical approval for this study was taken from ethical review board of University of Lahore (Ref No. ERC/02/20/02, dated February 25, 2020). Using maximum variation sampling 12 faculty members and 12 students from University College of Medicine and University College of Dentistry, Lahore were invited to participate. In addition to learning management system ‘Moodle’, these colleges have recently adopted ‘Zoom’ for interactive teaching in small and large group formats. The participants were also involved in online Problem-Based Learning sessions, along with regular online assessments during COVID-19 pandemic.
An interview guide was developed to explore faculty and students’ perception about online learning modalities, its advantages, limitations and recommendations. The interview guide was piloted to ensure comprehensiveness and then also validated by two medical education experts. 9 Two interviewers who were not involved in teaching and assessment of students conducted four focus group interviews (n=6 in each group) with faculty members (n=12) and students (n=12) of medicine and dentistry. The faculty and students were from both basic sciences (1 st and 2 nd year) and clinical sciences (3 rd , 4 th and final year). All interviews were recorded through ‘Zoom’ and subsequently transcribed verbatim. The data were thematically analyzed: compiling, disassembling, reassembling and interpretation by all the authors independently and then corroborated to ensure analytical triangulation.
The faculty members were predominantly females from both basic and clinical sciences with age range from 30-64 years. The students were from all professional years of MBBS and BDS program ( Table-I ).
Participant characteristics.
Faculty (n=12) | Students (n=12) | |||
---|---|---|---|---|
Male | 3(25%) | 7(58%) | ||
Female | 9(75%) | 5(42%) | ||
18-29 | 12 (100%) | |||
30-49 | 9(75%) | |||
50-64 | 3(25%) | |||
MBBS | BDS | |||
Basic Sciences | 2 (34%) | 3 (50%) | ||
Clinical Sciences | 4 (66%) | 3 (50%) | ||
MBBS | BDS | |||
1 year | 1 | 1 | ||
2 year | 1 | 1 | ||
3 Year | 1 | 2 | ||
4 Year | 1 | 2 | ||
5 Year | 2 |
Total six themes, two each for advantages, limitations and recommendations were extracted from the transcribed data after qualitative analysis ( Table-II ).
E-learning advantages, limitations and recommendations by Students and Faculty.
Themes | Sub-Themes | Excerpts |
---|---|---|
Advantages | ||
Flexibility | Remote learning | “It is useful in distant learning and during COVID 19 situation we can continue our education system”. |
Easy administration | “Our teacher has authority to unmute our mics and video. And can see and check whether we are listening attentively or not”. | |
Accessibility | “The students who are not much confident, they contact through the WhatsApp easily”. | |
Comfortable | “You can easily and comfortably listen to the lecture and learn”. | |
Student-centered learning | Self-directed learning | “I think eLearning is making good students more active and self-learner.” |
Asynchronous learning | “Second thing is that lectures have been recorded and will uploaded soon. It is easy for us to go back and go through the whole video for a summary or even revising it”. | |
Inefficiency | Unable to teach skills | “In anatomy, the study through models was good. But hands on training is not possible, the student will not be able to understand properly. Skills needs actual hands on training”. |
Lack of student feedback | “I find it annoying that during lectures you don’t have students feedback whether they are getting the point or not”. | |
Limited attention span | “There is no continuity of lecture. We lose our concentration and the syllabus is so lengthy.” | |
Lack of attentiveness | “As the students know that they will get the recordings, they don’t listen the lecture properly”. | |
Resource intensive | “Lots of people might not be having these gadgets. Buying these gadgets comes an extra burden on them in such stressful situation”. | |
Maintaining academic integrity | Lack of discipline | “There is some problem coming with discipline, some students use to misbehave during lectures”. |
Plagiarism | As this system is new to everyone, it is difficult to have individual assessment. During assignment, they easily copy paste stuff from web.” | |
Teaching and Assessment | Reduce cognitive load | “If you try to fix all the LOs in 40 minutes, then the interaction will not be possible.” |
Faculty development | “But we have to work with modality which institute has decided and using. But there is need of throughout training sessions”. | |
Increase Interactivities | “We should interact with students who are not active listeners. The student interaction is only through the assessments and we will be able to access the students.” | |
Incorporate CBL | “Case based learning is very important. It is the closest thing to the practical life. Making it easier, rather than making it complicated.” | |
Revision classes | “After this lockdown when the university will open, there should be a revision session and practical work.” | |
Integrate proper Assessment | “Assessment should be live videos and live recordings.” | |
Develop SOP’s | “The student should log in through proper ID and only they can listen the lecture and see video”. | |
Quality enhancement | Proctoring | “There should be plagiarism software to check assignment.” |
Buy Premium Applications | “I guess institute should buy premium package for ZOOM app so there will no time limit while having lectures.” |
Faculty opined that online learning helped ensure remote learning, it was manageable, and students could conveniently access teachers and teaching materials. It also reduced use of traveling resources and other expenses. It eased administrative tasks such as recording of lectures and marking attendance. Both the students and teachers had an opinion that online learning modalities had encouraged student-centeredness during this lockdown situation. The student had become self-directed learners and they learnt asynchronously at any time in a day.
Limitations
Faculty members and students said that through online learning modalities they were unable to teach and learn practical and clinical work. They could only teach and assess knowledge component. Due to lack of immediate feedback, teachers were unable to assess students’ understanding during online lecturing. The students also reported limited attention span and resource intensive nature of online learning as a limitation. Some teachers also mentioned that during online study, students misbehaved and tried to access online resources during assessments.
Recommendations
Teachers and students suggested continuous faculty development. They recommended a reduction in cognitive load and increased interactivities during online teaching. Those in clinical years suggested ways to start online Case Based Learning. However, some were also of the opinion that there should be revision classes along with psychomotor hands on teaching after the COVID-19 pandemic is under control. To enhance quality, they suggested buying premium software and other proctoring software to detect cheating and plagiarism.
The current study reported advantages, limitations and recommendations to improve online learning during lockdown of institutions due to COVID-19 pandemic. This study interprets perspectives of medical/dental students and faculty members, which showed that online learning modalities are flexible and effective source of teaching and learning along with some pitfalls. According to the teachers and students, online learning is a flexible and effective source of teaching and learning as most of them agreed upon the fact that this helps in distant learning with easy administration and accessibility along with less use resource and time. Regardless of time limit, students can easily access the learning material. This flexibility over face to face teaching has been reported in the literature as well. 2 The students also become self-directed learners, which is an important competency for encouraging lifelong learning among health professionals. 10 , 11
Both the faculty members and students viewed inefficiency to teach psychomotor skills, resource intensiveness and mismanaged decorum during sessions as limitations of online learning. Even though, hands-on sessions such as laboratory and clinical skills teaching have been disrupted during COVID-19 pandemic, we believe that online simulated patients or role plays can be used teach history taking, clinical reasoning and communication skills. Sharing recorded videos of laboratory and clinical skills demonstration is also worthwhile. Faculty members also complained about lack of students’ feedback regarding understanding of subject. Research showed that regular two-way feedback helps enhance self-efficacy and motivation. 12 The interaction between facilitator, learner and study material along with emotional and social support are essential ingredients for effective learning. 13 , 14 Internet connectivity issues also adversely impacted learning through online modalities, however, simply improving internet package/speed would help resolve this. Government should also take immediate measures and telecommunication companies should invest in expanding its 4G services across the country.
Recommendations reflect that decorum can be maintained by thorough supervision of students, setting ground rules for online interaction, counselling and disciplinary actions. 15 According to students, the attention span during online learning was even shorter than face to face sessions as also supported by the literature. 16 This can be managed by using flipped classroom learning modalities, giving shorter lectures and increasing teacher-student interaction. As ‘assessment drives learning’, so online formative assessments can be conducted through Socrative and Kahoot etc. Faculty needs training and students orientation in using online learning tools. 17 Investment in buying premium software packages will also help overcome many limitations and is therefore recommended.
Limitations of the Study
As the study participants belonged to the medical and dental college from a single private-sector university of Punjab, therefore the findings are only applicable to similar contexts. For generalizability, a survey based on our findings should be conducted across the province or country. Despite the limitations, the findings offer an understanding of the advantages, limitations and recommendations for improvement in online learning, which is the need of the day.
The current study supports the use of online learning in medical and dental institutes, considering its various advantages. E-learning modalities encourage student-centered learning and they are easily manageable during this lockdown situation. It is worth considering here that currently online learning is at a nascent stage in Pakistan. It started as ‘emergency remote learning’, and with further investments we can overcome any limitations. There is a need to train faculty on the use of online modalities and developing lesson plan with reduced cognitive load and increased interactivities.
Author’s Contribution
AS and MA conceived the idea , designed the study and are responsible for integrity of research.
KM and KJ collected the data.
All the authors contributed towards data analysis and writing the manuscript and approved the final version.
Acknowledgements
The authors would like to acknowledge the participants for their time and contributions.
Conflict of interest: None.
- Open access
- Published: 09 July 2024
Peer creation and sharing of mnemonics in collaborative documents for pathology education: a pilot study
- Bernd F. M. Romeike 1
BMC Medical Education volume 24 , Article number: 742 ( 2024 ) Cite this article
106 Accesses
Metrics details
Mnemonic techniques are memory aids that could help improve memory encoding, storage, and retrieval. Using the brain's natural propensity for pattern recognition and association, new information is associated with something familiar, such as an image, a structure, or a pattern. This should be particularly useful for learning complex medical information. Collaborative documents have the potential to revolutionize online learning because they could increase the creativity, productivity, and efficiency of learning. The purpose of this study was to investigate the feasibility of combining peer creation and sharing of mnemonics with collaborative online documents to improve pathology education.
We carried out a prospective, quasi-experimental, pretest–posttest pilot study. The intervention group was trained to create and share mnemonics in collaborative documents for pathological cases, based on histopathological slides. The control group compared analog and digital microscopy.
Both groups consisted of 41 students and did not reveal demographic differences. Performance evaluations did not reveal significant differences between the groups' pretest and posttest scores. Our pilot study revealed several pitfalls, especially in instructional design, time on task, and digital literacy, that could have masked possible learning benefits.
Conclusions
There is a gap in evidence-based research, both on mnemonics and on CD in pathology didactics. Even though, the combination of peer creation and sharing of mnemonics is very promising from a cognitive neurobiological standpoint, and collaborative documents have great potential to promote the digital transformation of medical education and increase cooperation, creativity, productivity, and efficiency of learning. However, the incorporation of such innovative techniques requires meticulous instructional design by teachers and additional time for students to become familiar with new learning methods and the application of new digital tools to promote also digital literacy. Future studies should also take into account validated high-stakes testing for more reliable pre-posttest results, a larger cohort of students, and anticipate technical difficulties regarding new digital tools.
Peer Review reports
Mnemonics in pathology education
For students in the medical field, the sheer volume of information in pathology can be overwhelming. The slightest tissue changes in histopathology can signify a wide spectrum of differential diagnoses. There are many patterns and medical terms that must be learned. Traditional pedagogy often fails to address the diverse learning styles of today's digital native students. This requires the exploration of new educational strategies to enhance learning outcomes.
Mnemonics have been used as a memory enhancement tool in pathology education [ 1 , 2 , 3 , 4 , 5 , 6 , 7 , 8 , 9 , 10 , 11 ]. Their value lies in condensing complex information into easily digestible and memorable fragments. Using cognitive shortcuts and associative memory, mnemonics transform challenging concepts into tangible and accessible pieces of knowledge. This involves fundamental linking of new information with existing long-term memory, usually with the help of mental images, which ultimately result in a memorable story. The most popular types of mnemonics in medical education are described in Table 1 .
In the realm of medicine, where intricate processes and numerous facts are the norm, mnemonics should prove useful.
Collaborative documents (CD)
Platforms such as Google Docs, Google Drive, Microsoft OneDrive, Wikis, and others could revolutionize the way we approach collaborative work and learning. The CDs on these platforms enable simultaneous input from multiple users and provide a space for creative interactions like document sharing, co-creating media, synchronous and asynchronous discussions, and modification of content in a fluid manner. This study opted for a device-independent, browser-based platform, in order to enable students to participate, regardless of their own devices. Therefore, we intentionally decided against solutions that would have required the installation of special software or applications.
The question arises whether CDs could have positive effects, such as improved collaboration, behavioral change, learning, or knowledge management [ 12 ]. There is some evidence that collaborative and peer-assisted learning can improve learning in the medical field [ 13 , 14 ] In pathology, especially digital slides have been shown to improve student learning and collaboration [ 14 , 15 , 16 , 17 ]. Surprisingly, a PubMed search for ‘collaborative document’ in August 2023 yielded only 15 hits. None of these articles is related to medical education or pathology training. Important healthcare applications that use collaborative documents include clinical information systems, document repositories, or research environments [ 18 , 19 , 20 ].
In this paper, we present a pilot study of the combination of these two powerful tools, mnemonics, and CD.
We propose that peer creation and sharing of mnemonics in collaborative online platforms offers a dual benefit: the mnemonic aids in memory retention, while the collaborative nature of the platform allows for the refinement, discussion, creativity, and personalization of these mnemonics. This approach democratizes the process of creating and sharing mnemonics and fosters a sense of community and shared understanding among learners.
This study also contributes to the awareness of mnemonics and the possible uses of CD in medical education, in general.
Participants and setting
Third-year full-time students in human medicine were followed during the course of systemic histopathology. The intervention group (IG) was encouraged to generate creative mnemonics and share them on CD. The control group (CG) was asked to compare analog and digital microscopy. The curriculum included 10 weekly courses that lasted 60 min each. Thus, the time on task was identical for IG and CG. All courses were given by the author of this manuscript on Wednesdays when groups 3 and 4 were taught. Groups 1 and 2 were taught on Mondays and groups 5 and 6 Fridays by other lecturers. The first course was used for a pretest (T0), an introduction of the course, and a description of learning strategies, followed by 8 courses (cardiovascular system, respiratory system, gastrointestinal system, liver, lymph nodes, urinary system, genital system, mamma and skin), during which a total of 39 microscopic slides were taught. The digital slides of the course are freely available on the homepage of our university medical center, although individual slides or courses are subject to change [ 21 ]. The final course was used for the posttest (T1), evaluation, and general questions and answers.
Students were required to attend course sessions, but participation in the study was completely voluntary. There were no explicit assignments for students to prepare for courses or study for the tests. The pretest and posttest were administered only for this study and were not a mandatory requirement for obtaining the course certificate. To pass the official final exam of the course, students had to recognize and describe two out of three randomly assigned histopathological slides.
Introduction to mnemonics
During the first course, students of the IG were introduced to mnemonics through a brief presentation. They also received a list of links to informative websites for self-directed learning of mnemonics (Table 2 ). Due to the limited available time, specific training of mnemonics could not be implemented during the courses.
Introduction to collaborative documents (CD)
For each pathological case or histologic slide, a collaborative document was generated at https://drive.google.com/ [ 26 ]. A device-independent, browser-based platform was chosen so that students could participate regardless of their devices, without having to install special software or applications. A blank template can be found as an additional file [see Additional file 1]. The CD included four different sections.
Section A: Mnemonics that connect the correct diagnosis with the macroscopic overview of the tissue on the slides, including outlines, shapes, or colors.
Section B: Mnemonics that connect the terms of histopathologic highlights with familiar words that sound similar and make a memorable sentence or story.
Section C: Mnemonics for other similarity of other new terms related to the specimen or the underlying disease.
Section D: Mnemonics that would minimize the risk of confusion of slides with similar appearance or terms with a risk of confusion.
During the first course, students of the IG were also introduced to the benefits of peer creation and collaboration in CD. Students received live instructions on how to use the platform-independent browser-based CD. During the courses, the CDs were projected onto the screen and worked on while the respective microscopic slides were demonstrated by the lecturer and studied by the students. The co-creating process should lead to a greater number of mnemonics, inspiration from peers, supporting discussions, and also later asynchronous personalization and refinement of mnemonics. CD might even foster a sense of community among students. To overcome inhibition thresholds, all students could work completely anonymously. Diversity and equity could be considered appropriately by letting students choose an avatar of choice.
Analog and digital microscopy
The students of the CG were free to choose digital slides with mobile devices or glass slides with analog microscopes. All digital slides of the course were freely available on the homepage of our university medical center 24/7 [ 21 ].
Course design
All courses started traditionally for both groups. The teacher described histopathological slides using digital slides throughout the course. All cardinal features were demonstrated and explained and the students were allowed to ask questions.
CG students were asked to compare analog and digital microscopy. IG students received QR codes that led them to the CD, where they could simultaneously create and share mnemonics during and even after the course. All CDs were available to IG students throughout the semester.
Pre- posttest design (quantitative data)
Participation in the study was voluntary. A pretest (T0) was performed before the first lesson and the interventions. The posttest (T1) was performed at the end of the semester. All tests were pseudonymized and included single choice and image recognition questions. In free text questions, students had to explain the pathogenesis and pathomechanisms or describe histopathological parameters.
Interest, previous knowledge, and learning strategies (qualitative data)
The pretest before intervention (T0) and the posttest after intervention (T1) tests were supplemented with short questionnaires that were distributed to all students. Ratings were on a Likert scale from 1 (high / strongly agree) to 6 (low / strongly disagree).
The students were asked for:
general interest in pathology
their estimation of the importance of pathological learning objectives for their own future post-graduate medical training
their previous knowledge of pathology
their preference to study alone rather than collaboratively
prior knowledge of mnemonics
their preference for digital versus analog microscopy
At the end of the semester, open questions concerned:
advantages and disadvantages of mnemonics (IG)
advantages and disadvantages of collaborative documents (IG)
advantages and disadvantages of analog or digital microscopy (CG)
The participants' responses were translated, structured, and paraphrased to capture the core meanings.
Data analysis
The sample size was calculated with an online tool [ 27 ]. With an effect size of η 2 = 0.1 (corresponding to an f of approximately.333, i.e., a small effect) and a power of 0.8, 37 subjects per group (74 total) were needed to obtain a significant result with a one-factor ANOVA (α = 0.05).
Quantitative data were analyzed by partial correlation, analysis of variance (ANOVA), multivariate analysis of variance (MANOVA), Shapiro–Wilk test for normal distribution, and Levene test for homogeneity of variances with SPSS 27 (IBM, Armonk, New York, USA).
Qualitative data were summarized by content and narrative analysis. Data from Likert scales were analyzed by the two-sample t-test assuming equal variances with SPSS 27 (IBM, Armonk, New York, USA).
The study was carried out according to the Declaration of Helsinki and was approved by the institutional ethics committee of the Rostock university hospital, reference number A 2019–0086.
Paticipants and setting
Due to organizational reasons, a randomization was not possible. Therefore, we relied on a pseudo-experimental study design. The entire semester of about 240 students consisted of six study groups (1–6). Two groups were enrolled in the pilot study described here, because the author of this article only taught groups 3 and 4, whose courses took place consecutively on Wednesdays. Group 4 was randomly assigned the IG and group 3 the CG. In each group, 41 students voluntarily participated in the study. The sample size of 41 can be considered sufficient as the power calculation suggested a minimum of 37 students per group. In the CG, 78% of the participants were women (32/9) compared to 68% (28/13) in the IG ( p = 0.4). There were no significant differences in age, which was 23.3 years in the IG and 22.9 in the CG ( p = 0.6).
The creation of mnemonics with CDs
The IG used CD for all 39 pathological cases / histopathological samples (100%). An abbreviated summary of all written entries of the students is provided as supplementary material [see Additional file 2]. Sometimes, students added screenshots of the digital slide overview ( n = 16) to better recall the shapes and outlines of the tissue. Each of the 39 CD sections A (outlines, form, and color) contained between one and three mnemonics. Often, an analogous picture was used that resembled the shape or outline of the sample, occasionally including color. There were a total of 59 entries (1.5 entries / slide). In Section B, which had a total of 56 entries, 10 CD did not have entries related to histopathological highlights. An example in German is the pathological term ‘Ballen’, which refers to bales. The students created a mnemonic with a word that sounds similar: ‘Quallen’, which means jellyfish in English. Section C had only five entries, one of which was Bilharziose (English equivalent of Schistosomiasis), which students associated with ‘Bill Clinton’ stuck in 'Harz' (English equivalent of resin) with ‘aliens sprouting from his liver’, as the original histological slide showed the parasites in the liver. Section D had only one entry, which was pathological Langhans cells represented as pennies on a plate (for the distribution pattern of nuclei in multinucleated giant cells), not to be confused with physiological single nucleated dendritic LangERhans cells of the skin.
Qualitative assessment of mnemonics and CD at the end of the semester
The open questions were summarized by content and narrative analysis. The IG was asked about the advantages of mnemonics and CDs. The most common answer was that the students could better remember the slides when they made associations of the shapes and outlines of the tissue with optical similarities. This simplified the learning process for 12 participants. Three learners each expressed their positive experiences with cooperation, collaboration, or finding it fun. Two students praised the effectiveness of mnemonics in improving their memory. Other students have reported being inspired by the innovation and have also found it useful that the CDs were available 24 h a day, 7 days a week.
As disadvantages, the students have mentioned that identification and documentation of mnemonics in collaborative documents takes additional time that should have been used for actual microscopic training ( n = 5). Furthermore, the students mentioned that the mnemonics could distract from the actual pathological learning objectives ( n = 5). Two students have reported experiencing technical difficulties with collaborative digital documents. They were unable to write or save documents on their mobile devices, especially smartphones. Other issues were inappropriate associations of other students that did not fit their own associations, lack of motivation, not enough pictures on the CD, not suitable for retrieval practice, and finally the statement ‘I don't use digital media for learning’.
Quantitative evaluation of the pretest (T0) and posttest (T1) results
In the IG, 40 of 41 students completed the pretest T0 (98%), and 33 (80%) completed the posttest T1. In the CG, 36 of 41 students completed the pretest T0 (88%), and the posttest T1 by 34 of 41 (83%). No statistical differences were found for the test scores (Fig. 1 ).
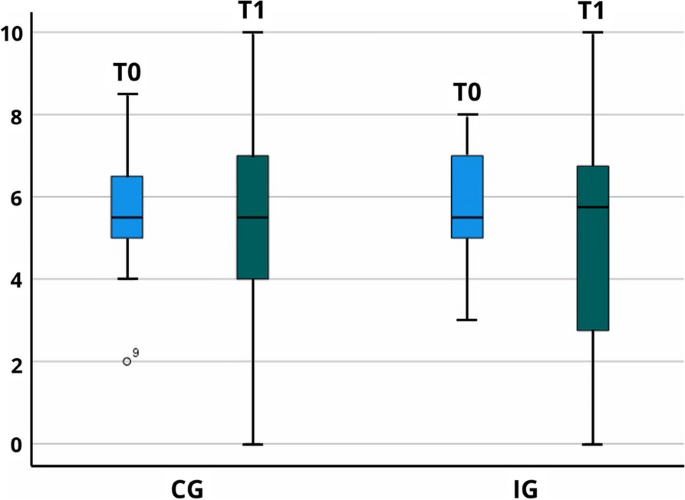
Box plot of IG and CG at T0 and T1
The ANOVA in T0 revealed no significant differences in the test scores for IG (M = 5.7, SD = 1.4) and CG (M = 5.6; SD = 1.3) (F = 0.02; p = 0.9). The homogeneity of the variances was asserted using the Levene test, which showed that equal variances could be assumed at T0 ( p = 0.5). ANOVA analysis in T1 also did not reveal significant differences in the test scores for IG (M = 5.1, SD = 2.6) and CG (M = 5.0; SD = 2.3) (F = 0.02; p = 0.9). Homogeneity of variances was asserted using the Levene test, which showed that equal variances could be assumed, also at T1 ( p = 0.4).
In MANOVA analysis, the test scores for T0 and T1 were normally distributed for both groups, as assessed by the Shapiro–Wilk test ( p > 0.05). There was homogeneity in error variances, as assessed by the Levene test ( p > 0.05). The homogeneity of the covariance was verified using the box test ( p = 0.844). Finally, there were no statistically significant differences between test scores and group, F (1.658) = 0.196, p = 0.66, partial η 2 = 0.003.
Qualitative assessment of interest, previous knowledge, and learning strategies
Our Likert scales ranged from 1 (high / strongly agree) to 6 (low / strongly disagree) and were analyzed using the two-sample t-test assuming equal variances. At T0, there were no significant differences in the means between the IG and the CG in terms of general interest in pathology (IG: 2.9; CG: 3; p = 0.5), the importance of pathological learning objectives for future postgraduate medical training (IG: 2.7; CG: 2.4; p = 0.2) or previous knowledge of pathology (IG: 3.7; CG: 3.7; p = 0.7).
In terms of learning strategies, IG and CG were not significantly different in their preference to study alone rather than collaboratively (IG: 3; CG: 2.4; p = 0.06), previous knowledge of mnemonics (IG: 3.2; CG: 3; p = 0.7) or in their preference for digital versus analog microscopy (IG: 3.2; CG: 3.4; p = 0.6).
Qualitative assessment of analog and digital microscopy at the end of the semester
The open questions were summarized by content and narrative analysis. The most common response from CG to the advantages of digital microscopy was the availability 24/7 for self-directed learning ( n = 17). This was followed by the quality and resolution of the digital slides ( n = 8). Regarding the advantages of digital microscopy, two students appreciated its better usability for collaborative peer learning, the ability to identify structures with the cursor, easy handling, and the opportunity to learn using identical specimens, not parallel slides. Other comments included better orientation, students' ability to select their own slides, the opportunity to measure objects, zooming possibilities, annotations, and improved motivation.
The most common problems mentioned by the students were sometimes limited server availability and slow internet connections ( n = 9), the quality and resolution of some slides ( n = 3), differences between analog and digital slides, or loss of experience handling classical analog microscopes (two each). A single student reported that using digital slides was less fun.
Synthesizing peer creation and sharing of mnemonics in collaborative online documents in histopathological education represents an innovative teaching method that adapts to cognitive neurobiology and the digital transformation of medical education. In this pilot study, we investigated the feasibility and effectiveness of integrating the creation and sharing of mnemonics on CD platforms to improve pathology learning among third-year medical students. Our study design included qualitative and quantitative measures to capture the nuances of student experiences and learning outcomes.
Whereas eponyms and acronyms, especially with food analogies, are an essential part of pathological textbooks, acrostics and other mnemonics are much rarer. A comprehensive literature search on mnemonics in pathology education revealed only 11 results [ 1 , 2 , 3 , 4 , 5 , 6 , 7 , 8 , 9 , 10 , 11 ]. Five of these are in the field of dermatopathology [ 2 , 5 , 6 , 8 , 10 ]. Most of these articles simply list a variable number of mnemonics. One larger study centered on food analogies and visualizations, but did not include a control group [ 9 ]. No prospective, randomized, controlled studies could be identified. In summary, this current literature does not reflect evidence-based research approaches. No obvious clues can be drawn for potential benefits or limitations, when we focus solely on pathology education. If we leave pathology didactics, a prospective randomized trial could show significant effects of mnemonics in the field of physiology [ 28 ]. Here, students in the mnemonics group scored significantly better in a pre-posttest setting. Although, our study could not prove a significant gain in pathological competences, the students stated that visual mnemonics simplified their learning process and improved their memory and that it was fun creating mnemonics. In family medicine, students also rated mnemonics very favorably, and they have proven useful for the generation of differential diagnosis [ 29 ].
As a limitation, our students mentioned inappropriate associations of other students’ metaphors that did not fit their own associations. This is a well-known phenomenon that is even more pronounced in students with different sociocultural backgrounds, which could have totally different associations with food-related medical terms [ 1 , 11 ].
Other limitations were lack of motivation and that learning mnemonics distracted them from learning pathological learning objectives. These statements make it clear that whenever a new technology is used, educators and students should know why they are using the technique and why it is appropriate to use it in that particular context [ 30 ]. Another study found that students who received specific instruction in metaphor comprehension strategies performed better than those who did not receive specific instruction and who even tended to adopt a violation strategy [ 31 ]. We were able to observe something similar with our students, especially since visual similarities of specimen outlines were the most popular mnemonic aid. This is actually an incorrect use of mnemonics because students do not learn pathological learning objectives, but only remember the slides by the outline and color of the specimens instead of the histopathological features. Our students obviously took the easy route and focused only on passing the final exam, during which they had to recognize three randomly assigned histopathological slides. This unintended shortcut should be considered in future studies, where we will try to counteract the preference of our medical students for superficial learning approaches [ 32 ].
- Collaborative documents
The students praised and found it fun using CD by creating and sharing mnemonics. By giving students the privilege of creating and witnessing the development of mnemonics in real time, the CD served as a means of facilitating this process. Although our own data remain inconclusive in terms of knowledge gain, there is good reason to continue to monitor and encourage collaborative learning [ 12 , 13 , 17 ].
Other students mentioned the benefits of 24/7 access to the CD. The inclusion of mnemonics on the CD was intended to facilitate learning by drawing connections between histological characteristics and common memorable cues. Central to this approach is the belief that collaboration leads to greater expertise and fosters motivation and creativity for the creation of mnemonics [ 13 , 15 ].
As a limitation, students have reported technical difficulties with collaborative digital documents, especially when using smartphones. This suggests that not all of our participants can be considered digital natives [ 33 ].
Digital vs. analog microscopy
Digital microscopy has its advantages: availability, high resolution, and potential for collaborative peer learning [ 14 , 15 , 16 , 17 ]. However, challenges such as slow Internet connections and server problems cannot be ignored. As evidenced by our student feedback, there is a distinct nostalgia among other students for analog methods and the tangible experience they provide. Integrating digital and analog microscopy methods into future curricula could provide a more holistic learning experience.
Limitations
The pilot study revealed three main limitations.
Wrong focus for mnemonics and not enough time for mnemonic creation, leading to a low number of mnemonics in CD.
Uniform test scores in the pre- and posttests.
Technical difficulties with the Internet and CD.
Too few mnemonics
Above, we already described the wrong focus of the students, using mnemonics mainly in section A only to memorize the specimen outlines and color, instead of the histopathological features. In sections B – C, only a few mnemonics were documented. The major problem was that it was too time-consuming to create useful mnemonics. Most of the students had only limited experience with mnemonics and, as with every new method, there is a variable learning curve that makes the creation of new mnemonics challenging for certain terms or concepts. Some students found the process to be not only time consuming, but also distracting. These findings suggest that while the integration of mnemonics in CDs has potential in pathology education [ 2 , 9 , 11 ], the time management, the process, and a more meticulous design of the instructions need to be refined. A more meticulous educational design [ 34 , 35 ] could prove more effectively, against our students for superficial learning approaches [ 32 ].
Uniform test scores
Despite the introduction of this promising and novel cognitive neurobiological method, no significant differences were observed in the pretest and posttest scores between the IG and the CG. Although all students received the same set of questions, we restricted the test questions to a minimum and did not validate them. Even more importantly, our pre- and posttests were not a prerequisite for passing the course and getting all credits. The tests were only conducted for the purpose of this study. Although exams are one of the main motivators to learn, they lose their effect when all theories of adult learning are bypassed if the exams are not relevant to passing [ 36 ]. In our cohort, the mediocre performance of all students in the pre- and posttest ultimately suggests that the two tests were too difficult for the students and were also not taken seriously. A follow-up study would have to use validated tests, which would also be decisive for passing the course.
Technical difficulties
Lack of accessibility or other problems with digital tools can deter participation and compromise learning outcomes. The students identified two different technical problems. One was related to digital microscopy and the other to CD. Regarding digital microscopy, our old platform in 2019 was obviously insufficient. It was a locally administered server with password protection. Meanwhile, all data are barrier-free on high-performance servers, allowing freely, reliably, easily, and fast access [ 21 ]. Regarding CD, we thought that we would already work with 'digital native students'. However, it became clear that the students had little or no experience using CDs with peers. Furthermore, some students stated that not all mobile devices, especially smartphones, managed CD with no difficulties. Therefore, we conclude that only a few, if any, ‘digital natives’ were enrolled in the 2019 course [ 33 ].
Implications for future studies
Various interpretations and potential improvements arise from our data.
Clear instructions
To establish a new learning technique and implement new digital learning tools, care must be taken to provide adequate explanations and follow instructional design rules for mnemonics and for CD [ 34 , 35 ].
Counteracting students’ bypasses
It must be taken into account that mnemonics focus on real pathological learning objectives and not support the preference of medical students for superficial learning approaches [ 32 ].
Enhanced training
Future implementations should consider more comprehensive training for students in the creation and sharing of effective mnemonics. The faster students develop mnemonics, the more time they will have for practical pathological training. An online inverted classroom model might help to learn how to create more functional mnemonics [ 37 ].
Mnemonic Individuality and quality
The collaborative nature, while beneficial for brainstorming, could become a source of confusion for some students. The challenge in a collaborative setting is to ensure mnemonics that resonate with the majority or to have enough mnemonics so that students can choose one that works best for them. However, the efficacy of a mnemonic is largely dependent on its relevance and ease of understanding. If the mnemonics created were not sufficiently relevant or memorable, their utility would be limited [ 2 ]. Our study did not assess the quality or relevance of the generated mnemonics. Future studies could include a qualitative analysis of mnemonics to assess their relevance and potential impact on learning outcomes.
Cognitive load
The process of developing mnemonics could create an additional cognitive load [ 38 ], potentially distracting from the core histopathological content. This is consistent with student feedback, where some found the mnemonics distracting. Students are required to acquire a vast amount of knowledge and skills, and a deliberate approach to instructional design is needed to provide students with effective learning strategies [ 34 , 35 ].
Time on task
As the students noted, the extra time spent on identification and documentation of mnemonics was removed from hands-on microscopy time. Obviously, there is a learning curve associated with adopting new study methods like creating mnemonics and working collaboratively on CD. Students may need more time and guidance to effectively integrate this technique into their study habits, which could dilute short-term results but prove beneficial in the long run.
Validated high-stakes testing
Most educators agree that (especially standardized) assessment drives learning [ 39 ]. For quality assurance, the tests should be validated and standardized in advance. Furthermore, the test should be mandatory to receive full credit for the course [ 36 ].
All digital tools, be they digital microscopy or CD, must be barrier-free (no passwords, free of charge), robust, user-friendly, and compatible with multiple devices, that is, platform-independent and browser-based.
Train the trainer
Pathologists as long-term teachers could be trained in a pathological didactic curriculum to better address the needs of their students and keep up with new evidence-based teaching methods [ 40 ].
Larger sample size
Although the sample size in this study was statistically appropriate for a pilot study or a feasibility study, a larger cohort could provide more nuanced information.
This pilot study provides a foundation on which future research can be built. Although the creation and sharing of mnemonics in CD offers promising opportunities to improve memory in pathology education, it is clear that the approach needs optimization. Despite the evidence-based, prospective, quasi-experimental, pre- posttest, mixed methods approach, a greater focus is imperative on educational and instructional design [ 34 , 35 ]. Dividing the sessions into mnemonic creation and practical histopathology could ensure focused learning. An online blended learning approach might also be beneficial [ 37 ]. Additionally, more reliable technological platforms are needed to evaluate digital slides, and working on CD may be the way forward. Although there is some evidence that student performance can be enhanced by desirable difficulty [ 38 ], we must be careful not to overburden our students. Finally, validated high-stakes tests should be used for our future pre-posttest study.
Availability of data and materials
No datasets were generated or analysed during the current study.
Abbreviations
Analysis of variance
Control group
International business machines
Id est: Latin for ‘in other words’
Intervention group
Multivariate analysis of variance
Statistical package for social sciences
United States of America
Ahmed H, Ogala WN, Ibrahim M. Culinary metaphors in western medicine: a dilemma of medical students in africa. Med Educ. 1992;26(5):423–4. https://doi.org/10.1111/j.1365-2923.1992.tb00195.x . PMID:1435383.
Article Google Scholar
Al Aboud K. Mnemonics in dermatopathology. Our Dermatol Online. 2012;3(4):359–60. https://doi.org/10.7241/ourd.20124.82 .
Batistatou A, Zolota V, Scopa CD. The “gourmet” pathologist. Int J Surg Pathol. 2000;8(4):341–2. https://doi.org/10.1177/106689690000800416 . PMID:11494014.
Bloomfield RL, Chandler ET. Mnemonics, rhetoric, and poetics for medics: Knowledge worth spewing not requiring emetics. Winston-Salem, N.C.: Harbinger Press; 1982.
Google Scholar
Bouganim N, Barankin B, Freiman A. Mnemonics in dermatology. Int J Dermatol. 2006;45(1):81–2. https://doi.org/10.1111/j.1365-4632.2004.02555.x . PMID:16426384.
Elston DM, Ferringer T, editors. Dermatopathology: requisites in dermatology. Saunders/Elsevier: Edinburgh, New York; 2008.
Pena GP, Andrade-Filho JDS. Analogies in medicine: valuable for learning, reasoning, remembering and naming. Adv Health Sci Educ Theory Pract. 2010;15(4):609–19. https://doi.org/10.1007/s10459-008-9126-2 . PMID:18528776.
Rubakovic S, Steffen C. Mnemonics in dermatology. Clin Dermatol. 2011;29(5):523–30. https://doi.org/10.1016/j.clindermatol.2010.09.019 . PMID:21855728.
Seto C, Zayat V. A spoonful of eponyms helps the pathology go down: using food eponyms and visual mnemonics in preclinical pathology education. Med Sci Educ. 2022;32(1):131–40. https://doi.org/10.1007/s40670-021-01474-w . PMID:35154897.
Smith J, Feldman SR. DERM: dermatologic education reinforced mnemonically. J Dermatolog Treat. 2015;26(6):491–2. https://doi.org/10.3109/09546634.2015.1106643 . PMID:26515160.
Masukume G, Zumla A. Analogies and metaphors in clinical medicine. Clin Med (Lond). 2012;12(1):55–6. https://doi.org/10.7861/clinmedicine.12-1-55 . PMID:22372224.
Archambault PM, van de Belt TH, Kuziemsky C, et al. Collaborative writing applications in healthcare: effects on professional practice and healthcare outcomes. Cochrane Database Syst Rev. 2017;5(5):1–68. https://doi.org/10.1002/14651858.CD011388.pub2 . PMID:28489282.
Sevenhuysen S, Haines T, Kiegaldie D, Molloy E. Implementing collaborative and peer-assisted learning. Clin Teach. 2016;13(5):325–31. https://doi.org/10.1111/tct.12583 . PMID:27624197.
Yang C, Davis K, Head M, et al. Collaborative learning communities with medical students as teachers. J Med Educ Curric Dev. 2023;10:1–10. https://doi.org/10.1177/23821205231183878 . PMID:37362582.
Braun MW, Kearns KD. Improved learning efficiency and increased student collaboration through use of virtual microscopy in the teaching of human pathology. Anat Sci Educ. 2008;1(6):240–6.https://doi.org/10.1002/ase.53. PMID:19109852.
Sahota M, Leung B, Dowdell S, Velan GM. Learning pathology using collaborative vs. individual annotation of whole slide images: a mixed methods trial. BMC Med Educ 2016;16(1). [https://doi.org/10.1186/s12909-016-0831-x][PMID:27955651]
Romeike BFM, Fischer M. Buzz groups facilitate collaborative learning and improve histopathological competencies of students. Clin Neuropathol. 2019;38(6):235–93. https://doi.org/10.5414/NP301195 . PMID:31296286.
Stein DM, Wrenn JO, Johnson SB, Stetson PD. Signout: a collaborative document with implications for the future of clinical information systems. AMIA Annu Symp Proc. 2007;2007:696–700 PMID:18693926.
Pinelle D, Gutwin C. A collaborative document repository for home care teams. AMIA Annu Symp Proc. 2005;2005:1082 PMID:16779369.
Romano P, Giugno R, Pulvirenti A. Tools and collaborative environments for bioinformatics research. Brief Bioinform. 2011;12(6):549–61. https://doi.org/10.1093/bib/bbr055 . PMID:21984743.
Romeike BFM. 3D Makroskopie und digitale Mikroskopie: Frei verfügbare 3D-Modelle und digitale Mikroskopie von normalen und pathologischen Organen und Geweben: Dean’s Office for Student Affairs, Medical Education, University Medical Center; 2019. Available from: URL: https://www.med.uni-rostock.de/forschung-lehre/studium-und-lehre/3d-makroskopie-und-digitale-mikroskopie . Cited 2024 May 18.
Mnemotechnik - Wikipedia. Available from: URL: https://de.wikipedia.org/wiki/Mnemotechnik . Cited 2024 May 18.
Memocamp - das Portal für Gedächtnistraining. Available from: URL: https://memocamp.com/de . Cited 2024 May 18.
Art of memory | Improve your memory with free brain training games and software. Available from:https://artofmemory.com/. Cited 2024 May 18.
Nelson Dellis - 5x USA memory champion - Teaching the world how to remember. Available from: URL: https://www.nelsondellis.com/. Cited 2024 May 18.
Google drive. Available from: URL:https://drive.google.com/drive/home. Cited 2024 May 18.
Hemmerich WA. StatistikGuru: Stichprobengröße für die einfaktorielle ANOVA berechnen.; 2018. Available from: URL:https://statistikguru.de/rechner/stichprobengroesse-einfaktorielle-anova.html. Cited 2024 May 18.
Meenu S, Zachariah AM, Balakrishnan S. Effectiveness of mnemonics based teaching in medical education. ijhs 2022; 6(S2):9635–40. [ https://doi.org/10.53730/ijhs.v6nS2.7599 ]
Leeds FS, Atwa KM, Cook AM, Conway KA, Crawford TN. Teaching heuristics and mnemonics to improve generation of differential diagnoses. Med Educ Online. 2020;25(1):1742967. https://doi.org/10.1080/10872981.2020.1742967 . PMID:32182197.
Putnam AL. Mnemonics in education: Current research and applications. Translational Issues in Psychological Science 2015; 1(2): 130–9. [ https://doi.org/10.1037/tps0000023 ]
Canziani T. Metaphors in the mirror: the influence of teaching metaphors in a medical education programme. Language Learning in Higher Education. 2016;6:185–206.
Hilliard RI. How do medical students learn: medical student learning styles and factors that affect these learning styles. Teach Learn Med. 1995;7(4):201–10. https://doi.org/10.1080/10401339509539745 .
Smith EE, Kahlke R, Judd T. Not just digital natives: integrating technologies in professional education contexts. AJET. 2020;36(3):1–13. https://doi.org/10.14742/ajet.5689 .
Andreea E. Instructional design in education. IJAEDU. 2022;8(24):219–24. https://doi.org/10.18768/ijaedu.1204180 .
Chen W, Reeves T. Twelve tips for conducting educational design research in medical education. Med Teach. 2019;42:980–6.
Taylor DCM, Hamdy H. Adult learning theories: implications for learning and teaching in medical education: AMEE Guide No. 83. Medical Teacher. 2013;35(11):1561–72. https://doi.org/10.3109/0142159X.2013.828153 . PMID:24004029.
Tolks D, Romeike BF, Ehlers J, et al. The online inverted classroom model (oICM). A blueprint to adapt the inverted classroom to an online learning setting in medical and health education. MedEdPublish. 2016;2020(9):113. https://doi.org/10.15694/mep.2020.000113.1 . PMID:38073851.
Nelson A, Eliasz KL. Desirable difficulty: theory and application of intentionally challenging learning. Med Educ. 2023;57(2):123–30. https://doi.org/10.1111/medu.14916 . PMID:35950522.
Wormald BW, Schoeman S, Somasunderam A, Penn M. Assessment drives learning: an unavoidable truth? Anat Sci Educ. 2009;2(5):199–204. https://doi.org/10.1002/ase.102 . PMID:19743508.
Romeike B, Schnabel K. Pathology didactics : Development and pilot study of a pathology didactics curriculum (PaDiCu. Pathologe. 2019;40(2):169–71. https://doi.org/10.1007/s00292-018-0557-0 . PMID:30617604.
Download references
Acknowledgements
This study was supported by Prof. Dr. med. Andreas Erbersdobler, Director of the Institute of Pathology of the University Medical Center, Strempelstrasse 14, D-18057 Rostock, Germany and his staff.
Clinical trial number
No clinical trial number was obtained because our research study did not prospectively assign human participants or groups of humans to one or more health-related interventions to evaluate the effects on health outcomes.
Open Access funding enabled and organized by Projekt DEAL. This research did not receive funding.
Author information
Authors and affiliations.
Dean’s Office for Student Affairs, Medical Education, University Medical Center, Ernst-Heydemann-Strasse 8, D-18057, Rostock, Germany
Bernd F. M. Romeike
You can also search for this author in PubMed Google Scholar
Contributions
B.F.M.R. conceived the study design, performed the data collection, carried out the statistical analyses, and wrote and proofread the final manuscript.
Corresponding author
Correspondence to Bernd F. M. Romeike .
Ethics declarations
Ethics approval and consent to participate.
The study was carried out according to the Declaration of Helsinki and was approved by the Institutional Ethics Committee of the Rostock University Hospital, reference number A 2019–0086; May 2019.
Informed consent to participate in the study was obtained verbally during the first lecture. Participants received all information necessary to make an informed decision about their participation, including the purpose of the study, procedures, risks, benefits, and their rights as participants. Participation in the study was completely voluntary for all students. All data utilized were strictly pseudonymized, ensuring that the identity of individual participants remained confidential and protected throughout the research process. Given the nature and scope of our research, we determined that written consent would be impractical, primarily due to logistical constraints and the nature of data collection. Moreover, our assessment concluded that the research involves minimal risk to participants. The study neither involves sensitive personal data nor does it pose any psychological, social, or physical risks to the individuals involved.
Consent for publication
Not applicable.
Competing interests
The authors declare no competing interests.
Additional information
Publisher’s note.
Springer Nature remains neutral with regard to jurisdictional claims in published maps and institutional affiliations.
Supplementary Information
Additional file 1. blank template of a collaborative document, translated into english..
Additional file 2: Supplementary Table 3. Summary of translated student entries in CD; Description: Sections A and B appear in columns 2 and 3. The few entries in Sections C (new terms related to the specimen or the underlying disease reminded me of,…) and D (Risk of confusion with…) appear in additional inserted rows.
Rights and permissions
Open Access This article is licensed under a Creative Commons Attribution 4.0 International License, which permits use, sharing, adaptation, distribution and reproduction in any medium or format, as long as you give appropriate credit to the original author(s) and the source, provide a link to the Creative Commons licence, and indicate if changes were made. The images or other third party material in this article are included in the article's Creative Commons licence, unless indicated otherwise in a credit line to the material. If material is not included in the article's Creative Commons licence and your intended use is not permitted by statutory regulation or exceeds the permitted use, you will need to obtain permission directly from the copyright holder. To view a copy of this licence, visit http://creativecommons.org/licenses/by/4.0/ . The Creative Commons Public Domain Dedication waiver ( http://creativecommons.org/publicdomain/zero/1.0/ ) applies to the data made available in this article, unless otherwise stated in a credit line to the data.
Reprints and permissions
About this article
Cite this article.
Romeike, B.F.M. Peer creation and sharing of mnemonics in collaborative documents for pathology education: a pilot study. BMC Med Educ 24 , 742 (2024). https://doi.org/10.1186/s12909-024-05743-1
Download citation
Received : 07 January 2024
Accepted : 03 July 2024
Published : 09 July 2024
DOI : https://doi.org/10.1186/s12909-024-05743-1
Share this article
Anyone you share the following link with will be able to read this content:
Sorry, a shareable link is not currently available for this article.
Provided by the Springer Nature SharedIt content-sharing initiative
- Digital literacy
- Dynamic online learning
- Instructional design
- Medical education
- Pathology education
- Peer creating
- Peer sharing
BMC Medical Education
ISSN: 1472-6920
- Submission enquiries: [email protected]
- General enquiries: [email protected]
- My Account |
- StudentHome |
- TutorHome |
- IntranetHome |
- Contact the OU Contact the OU Contact the OU |
- Accessibility hub Accessibility hub
Postgraduate
- International
- News & media
- Business & apprenticeships
Centre for Policing Research and Learning
You are here, online seminar: how to make collaborative governance work: potential benefits and challenges of network management and user and community co-production.

2023 Annual Report
This annual report features a selection of the work undertaken across 2023 which we consider to be highly relevant for the current policing environment.
- Launch of Openlearn Policing Practice and Leadership Hub 14th May 2024
- Ten-years of Policing at the OU 5th April 2024
- Launch of CPRL Small Grants Pilot Scheme: Testing Policing Innovations 26th March 2024
The Open University
- Study with us
- Work with us
- Supported distance learning
- Funding your studies
- International students
- Global reputation
- Sustainability
- Apprenticeships
- Develop your workforce
- Contact the OU
Undergraduate
- Arts and Humanities
- Art History
- Business and Management
- Combined Studies
- Computing and IT
- Counselling
- Creative Arts
- Creative Writing
- Criminology
- Early Years
- Electronic Engineering
- Engineering
- Environment
- Film and Media
- Health and Social Care
- Health and Wellbeing
- Health Sciences
- International Studies
- Mathematics
- Mental Health
- Nursing and Healthcare
- Religious Studies
- Social Sciences
- Social Work
- Software Engineering
- Sport and Fitness
- Postgraduate study
- Research degrees
- Masters in Social Work (MA)
- Masters in Economics (MSc)
- Masters in Creative Writing (MA)
- Masters in Education (MA/MEd)
- Masters in Engineering (MSc)
- Masters in English Literature (MA)
- Masters in History (MA)
- Masters in International Relations (MA)
- Masters in Finance (MSc)
- Masters in Cyber Security (MSc)
- Masters in Psychology (MSc)
- A to Z of Masters degrees
- OU Accessibility statement
- Conditions of use
- Privacy policy
- Cookie policy
- Manage cookie preferences
- Modern slavery act (pdf 149kb)
Follow us on Social media
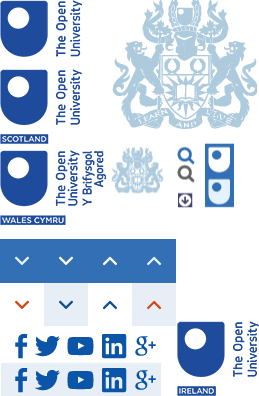
- Student Policies and Regulations
- Student Charter
- System Status
- Contact the OU Contact the OU
- Modern Slavery Act (pdf 149kb)
© . . .

IMAGES
VIDEO
COMMENTS
The advantages of online learning compared to face-to-face learning in the classroom is the flexibility of learning time in online learning, the learning time does not include a single program, and it can be shaped according to circumstances (Lai et al., 2019). The next advantage is the ease of collecting assignments for students, as these can ...
This systematic analysis examines effectiveness research on online and blended learning from schools, particularly relevant during the Covid-19 pandemic, and also educational games, computer-supported cooperative learning (CSCL) and computer-assisted instruction (CAI), largely used in schools but with potential for outside school.
This review enabled us to identify the online learning research themes examined from 2009 to 2018. In the section below, we review the most studied research themes, engagement and learner characteristics along with implications, limitations, and directions for future research. 5.1. Most studied research themes.
Online education has advantages and disadvantages. The advantages of online learning compared to face-to-face learning in the classroom is the flexibility of learning time in online learning, the learning time does not include a single program, and it can be shaped according to circumstances (Lai et al., 2019). The next advantage is the ease of ...
In response to the COVID-19 pandemic, technological and administrative systems for implementing online learning, and the infrastructure that supports its access and delivery, had to adapt quickly.
Nashville, TN 3720 3 USA. t [email protected]. Abstract. The physical "brick and mortar" classroom is starting to lose its monopoly as the place of. learning. The Internet has made ...
In a recent study, researchers found that 18% of parents pointed to greater flexibility in a child's schedule or way of learning as the biggest benefit or positive outcome related to remote learning ( School Psychology, Roy, A., et al., in press).
Online learning is one of the educational solutions for students during the COVID-19 pandemic. Worldwide, most universities have shifted much of their learning frameworks to an online learning model to limit physical interaction between people and slow the spread of COVID-19. The effectiveness of online learning depends on many factors, including student and instructor self-efficacy, attitudes ...
In research examining student outcomes in the context of online learning, the prevailing trend is the consistent observation that online learners often achieve less favorable results when compared ...
Research focusing on problem- and project-based learning in online K-12 is quite sparse, however the benefits of collaborative work, problem solving, and grounding learning in real-world contexts—all key components of these approaches—are supported by the literature base in K-12 online learning (e.g., Boston et al., 2009; Di Pietro et ...
Most online courses, however, particularly those serving K-12 students, have a format much more similar to in-person courses. The teacher helps to run virtual discussion among the students ...
Not everyone loved online learning during the pandemic — especially in the early stages, when it was at its most haphazard. Nearly three in 10 students in a Strada Education survey in the fall ...
Abstract. Online learning—learning that involves interactions that are mediated through using digital, typically internet-based, technology—is pervasive, multi-faceted, and evolving, creating opportunities and challenges for educational research in the wake of the COVID-19 pandemic.In this special issue, we advance an interdisciplinary agenda for online learning research at the ...
1.1. Related literature. Online learning is a form of distance education which mainly involves internet‐based education where courses are offered synchronously (i.e. live sessions online) and/or asynchronously (i.e. students access course materials online in their own time, which is associated with the more traditional distance education).
Rapid developments in technology have made distance education easy (McBrien et al., 2009).). "Most of the terms (online learning, open learning, web-based learning, computer-mediated learning, blended learning, m-learning, for ex.) have in common the ability to use a computer connected to a network, that offers the possibility to learn from anywhere, anytime, in any rhythm, with any means ...
Finally, although this research has the advantages of large sample size and ease of online data collection, it does rely on self-reported measures, which may not always be objective. ... While there may be some benefits of online learning in the realm of engagement, it seems that there are also some sacrifices online learners make when it comes ...
Despite the advantages of online learning, there are still numerous challenges for students, administration, and faculty, whether in regular environments or emergencies. ... Boolaky M. (2020). The missing HEROs: The absence of, and need for, PsyCap research of online university students. Open Learning: The Journal of Open, Distance and e ...
Online learning is a popular alternative to traditional on-campus, in-person learning. In 2019, Research and Markets forecasted that the online education market will reach $230 billion by 2025—and given the major impact of COVID-19, it's likely that online programs will see even greater growth. In fact, the pandemic has demonstrated what a practical, sustainable model online learning is.
Learning through online has advantages and disadvantages. Online learning activities can make learning not monotonous, time efficiency and the materials that are very easy to access (Yuhanna et al ...
This research article focused on the advantages and disadvantages of online learning in an international school called Cambridge International School in Istanbul, Turkey.
This allows students to access the learning material at a time of their comfort. Thus, online learning offers students the accessibility of time and place in education. 3. Affordability. Another advantage of online learning is reduced financial costs. Online education is far more affordable as compared to physical learning.
Online learning has many advantages, such as quick access to material and convenience of taking classes at any location (Sun and Rueda, 2012; Simamora, 2020). But online learning presents challenges for student engagement, that is, the types and levels of effort students put into perform well and achieve their goals (Hu & Kuh, 2002; Richardson, Long & Foster, 2004).
Moreover, much of the existing research focuses on specific aspects of digital learning such as access to online learning materials (e.g. Summers et al., Citation 2023), whereas students need devices that can produce presentations and reports, access online assessments, and run technical and specialist software, as well as facilitating ...
The increasing prevalence of Artificial Intelligence (AI) in higher education underscores the necessity to explore its implications on ethical, social, and educational dynamics within the sector.
The advantages included remote learning, comfort, accessibility, while the limitations involved inefficiency and difficulty in maintaining academic integrity. The recommendations were to train faculty on using online modalities and developing lesson plan with reduced cognitive load and increased interactivities.
This review enabled us to identify the online learning research themes examined from 2009 to 2018. In the section below, we review the most studied research themes, engagement and learner characteristics along with implications, limitations, and directions for future research. 5.1. Most studied research themes.
Background Mnemonic techniques are memory aids that could help improve memory encoding, storage, and retrieval. Using the brain's natural propensity for pattern recognition and association, new information is associated with something familiar, such as an image, a structure, or a pattern. This should be particularly useful for learning complex medical information. Collaborative documents have ...
Online Seminar: How to make collaborative governance work: Potential benefits and challenges of network management and user and community co-production Dates Monday, July 1, 2024 - 13:00 to 14:30